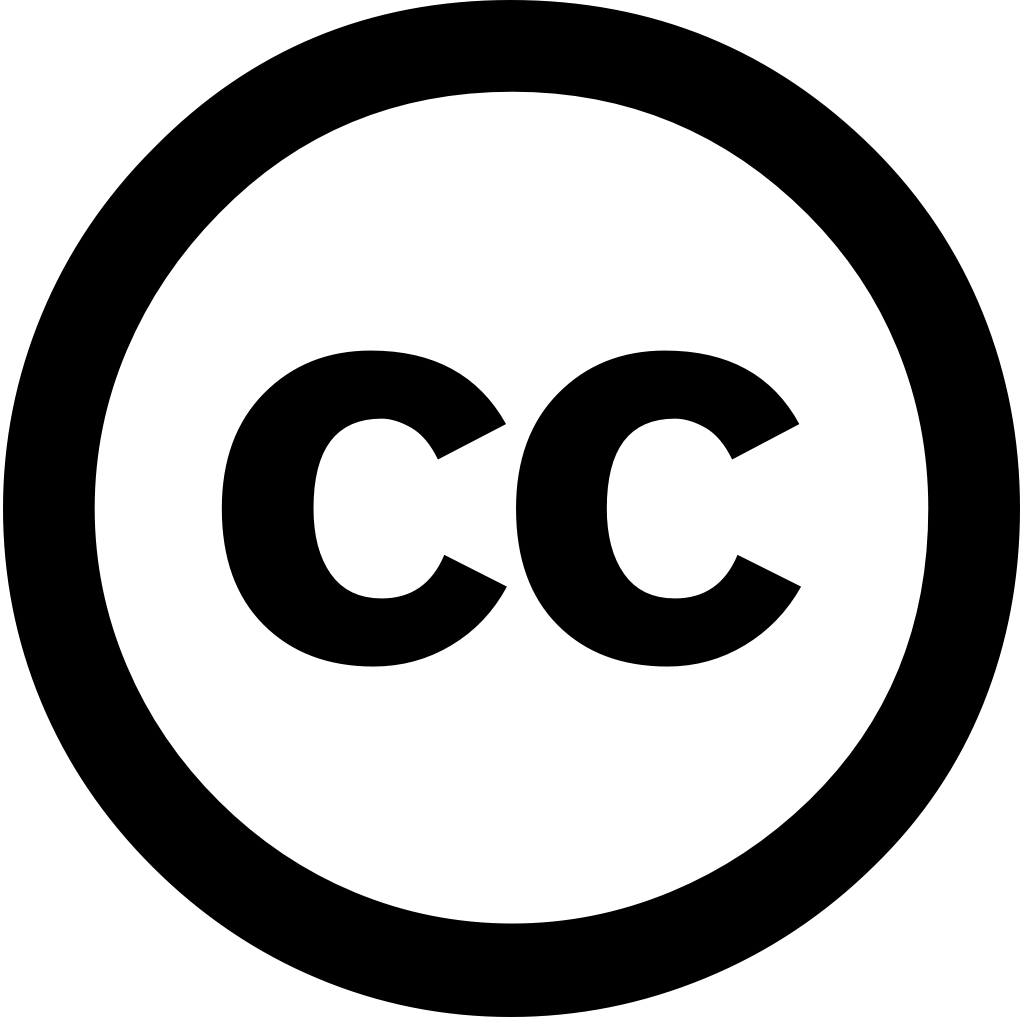
AI, Journal Year: 2025, Volume and Issue: 6(3), P. 58 - 58
Published: March 13, 2025
Accurate and reliable crop yield prediction is essential for optimizing agricultural management, resource allocation, decision-making, while also supporting farmers stakeholders in adapting to climate change increasing global demand. This study introduces an innovative approach by incorporating spatially lagged spectral data (SLSD) through the spatial-lagged machine learning (SLML) model, enhanced version of spatial lag X (SLX) model. The research aims show that SLSD improves compared traditional vegetation index (VI)-based methods. Conducted on a 19-hectare cornfield at ARS Grassland, Soil, Water Research Laboratory during 2023 growing season, this used five-band multispectral image 8581 measurements ranging from 1.69 15.86 Mg/Ha. Four predictor sets were evaluated: Set 1 (spectral bands), 2 bands + neighborhood data), 3 VIs), 4 top VIs data). These evaluated using SLX model four decision-tree-based SLML models (RF, XGB, ET, GBR), with performance assessed R2 RMSE. Results showed (Set 2) outperformed VI-based approaches 3), emphasizing importance context. models, particularly RF, performed best 4–8 neighbors, excessive neighbors slightly reduced accuracy. In 3, improved predictions, but smaller subset (10–15 indices) was sufficient optimal prediction. slight gains over Sets XGB RF achieving highest values. Key predictors included (e.g., Green_lag, NIR_lag, RedEdge_lag) CREI, GCI, NCPI, ARI, CCCI), highlighting value integrating corn underscores context lays foundation future across diverse settings, focusing size, data, refining dependencies localized search algorithms.
Language: Английский