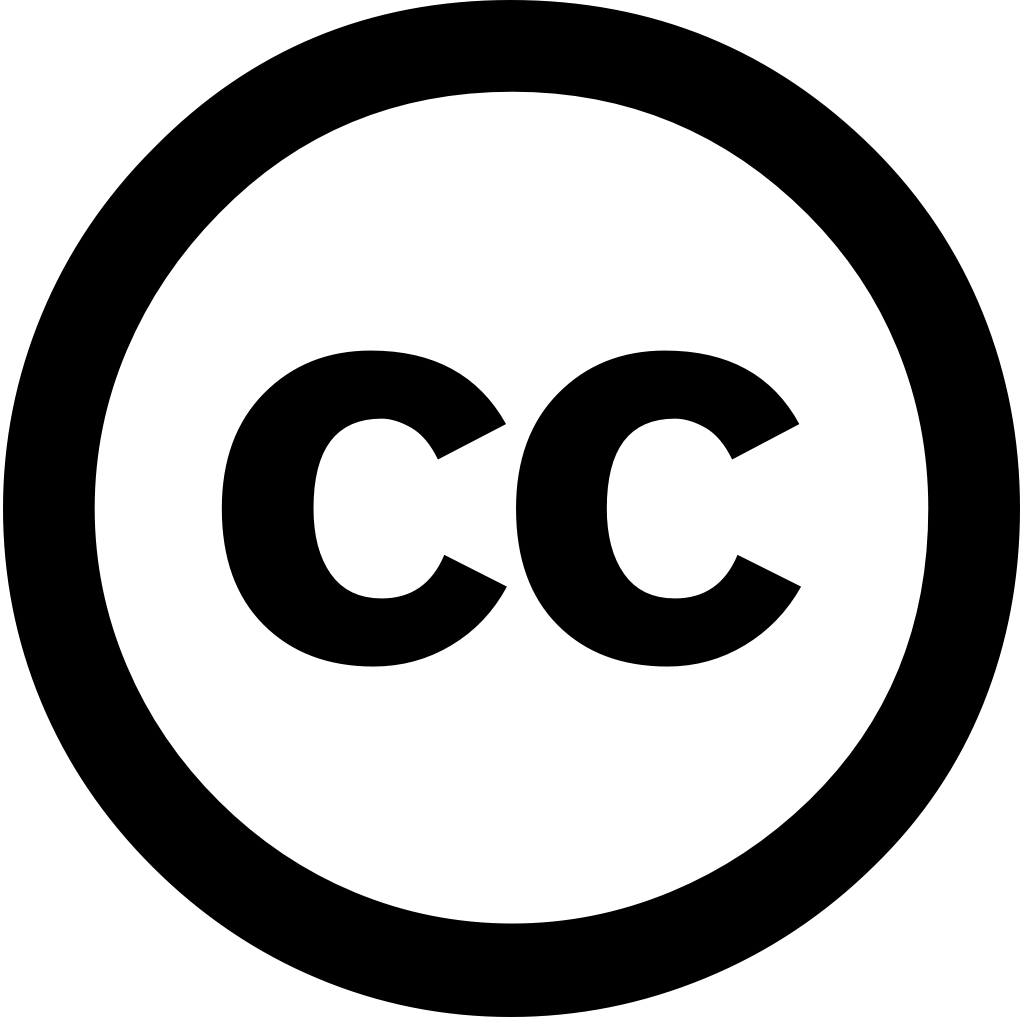
Published: Aug. 29, 2024
The dynamic properties of enzymatic reaction networks (ERNs) are difficult to predict due the emergence allosteric interactions, product inhibitions and competition for resources, that all only materialize once have been assembled. Combining experimental kinetics studies with computational modelling allows us extract information on these emergent build predictive models. Here, we utilized pentose phosphate pathway demonstrate previously reported approaches construct maximally informative datasets can be significantly improved by pulsing both enzymes substrates into microfluidic flow reactors (instead only). Our method augments available from online databases, map behaviours a network.
Language: Английский