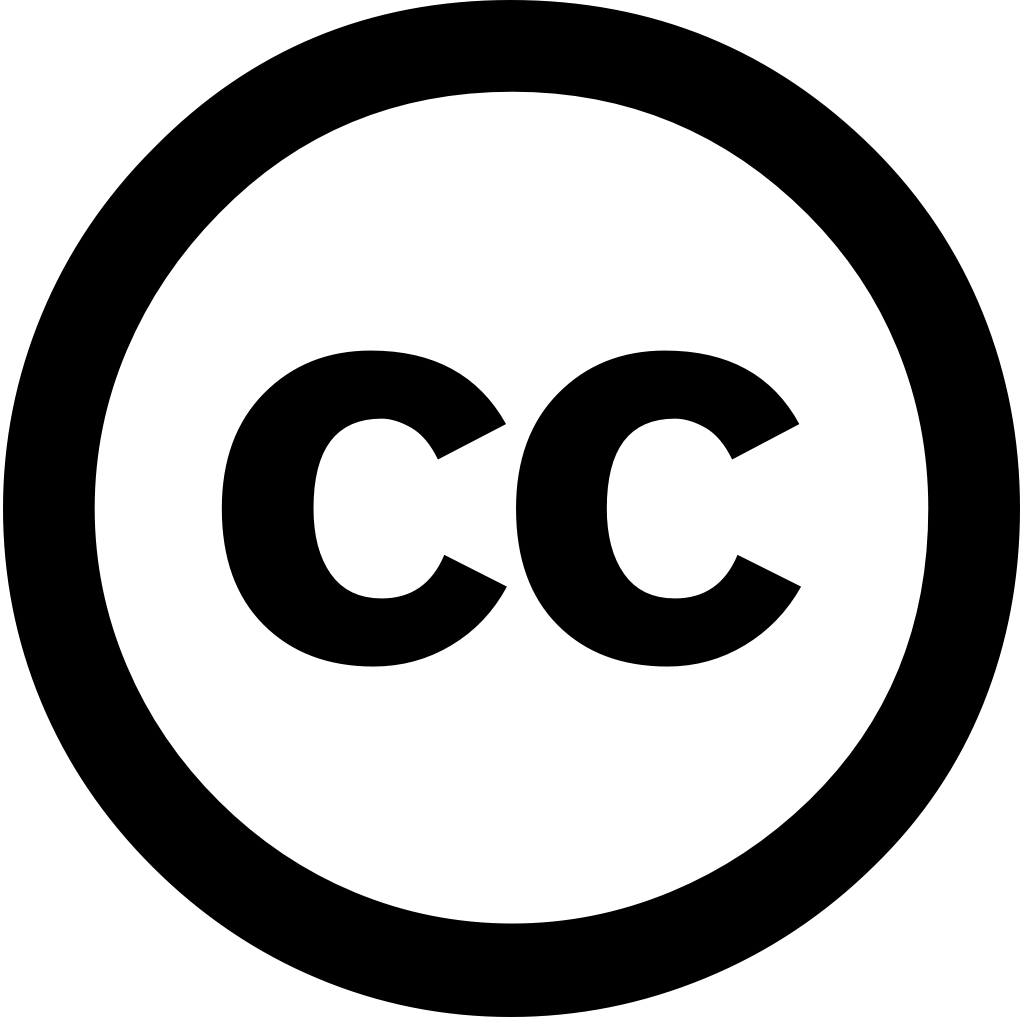
Frontiers in Marine Science, Journal Year: 2024, Volume and Issue: 11
Published: Dec. 24, 2024
Introduction In order to explore the impact of climate factors on bigeye tuna catch, monthly data nine factors, including El Niño-related indices (Niño1 + 2, Niño3, Niño4, and Niño3.4), Southern Oscillation Index (SOI), North Atlantic (NAO), Pacific Decadal (PDO), (NPI), global sea–air temperature anomaly index (dT), were combined with annual catch. Methods The relationship between low-frequency catch was studied using long short-term memory(LSTM) model, random forest (RF) BP neural network extreme gradient boosting tree (XGBoost) Sparrow search optimization algorithm (SSA-XGBoost) model. Results results show that optimal lag periods corresponding change characterization Niño1 dT, SOI, NPI, NAO, PDO are 15 years,12 years, 12 1 year, 14 4 respectively. SSA-XGBoost model have highest prediction accuracy, followed by XGBoost, BP, LSTM, RF. fitting degree predicted values actual is 0.853, mean absolute error 0.104, root square 0.124. Discussion trend generally consistent, indicating good performance, which can provide a basis for management fisheries.
Language: Английский