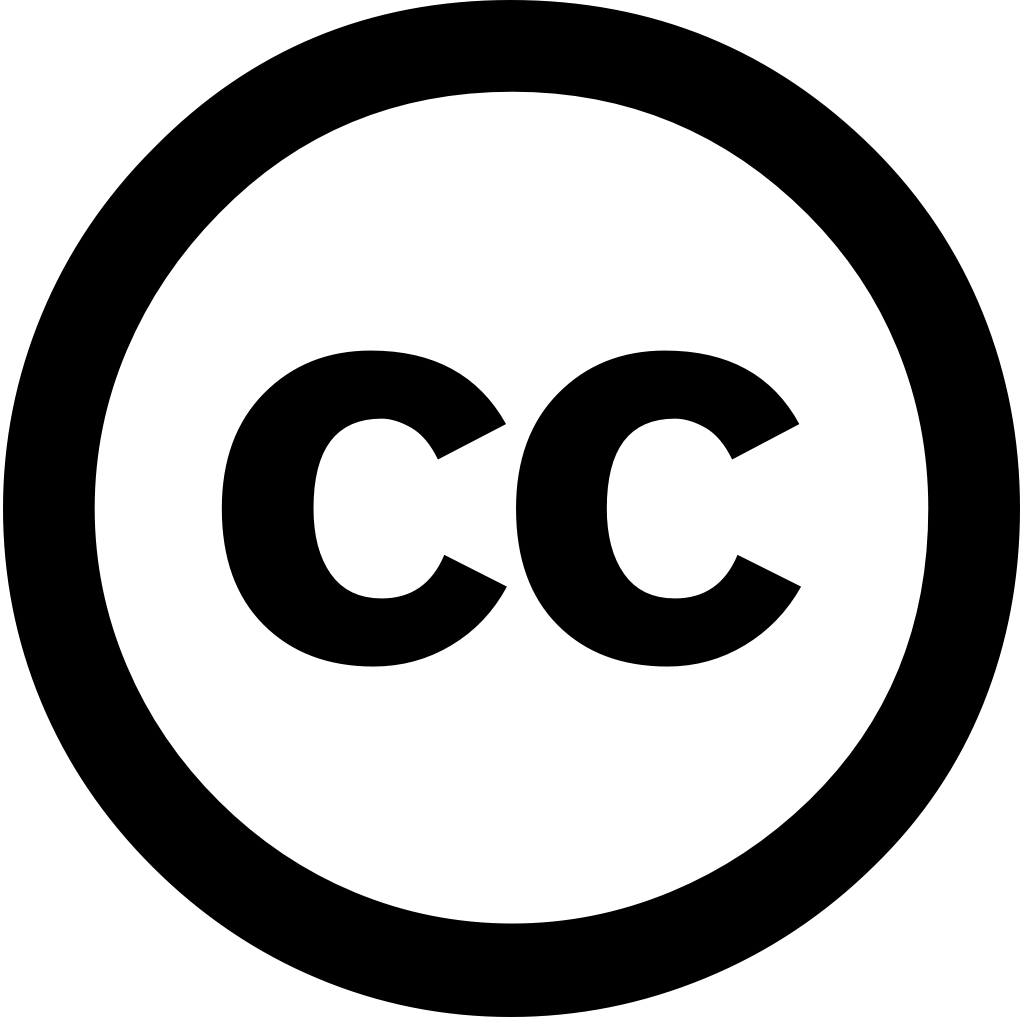
The Journal of Prevention of Alzheimer s Disease, Journal Year: 2025, Volume and Issue: unknown, P. 100115 - 100115
Published: March 1, 2025
Integrating machine learning with medical records offers potential for early detection of Alzheimer's disease (AD), enabling timely interventions. This study aimed to evaluate the effectiveness in constructing a predictive model AD, designed predict AD data up three years before diagnosis. Using clinical data, including prior diagnoses and treatments, we sought enhance sensitivity specificity diagnostic procedures. A second aim was identify most important factors models, as these may be predictors AD. The employed Stochastic Gradient Boosting, method, using primary healthcare data. analyses were stratified by sex age groups. included individuals within Region Stockholm, Sweden, from 2010 2022. analyzed over 40. Patients an diagnosis (ICD-10-SE codes F00 or G30) during 2010-2012 excluded ensure prospective modeling. In total, identified 3,407 patients aged 41-69 25,796 69. ranked diagnoses, performance assessed area under receiver operating characteristic curve (AUC). Known novel evaluated their contribution risk. AUC values ranged 0.748 (women 41-69) 0.816 69), men across groups falling this range. Sensitivity 0.73 0.79 0.66 0.79, respectively, gender Negative consistently high (≥0.954), while positive lower (0.199-0.351). Additionally, confirmed known risk that warrant further investigation. Key observations, cognitive symptoms, antidepressant treatment, visit frequency, vitamin B12/folic acid treatment. Machine applied shows promise predicting robust findings factors, such depression B12 deficiency, also identifying guide future research. Clinically, approach could stratification, facilitating interventions improving patient outcomes.
Language: Английский