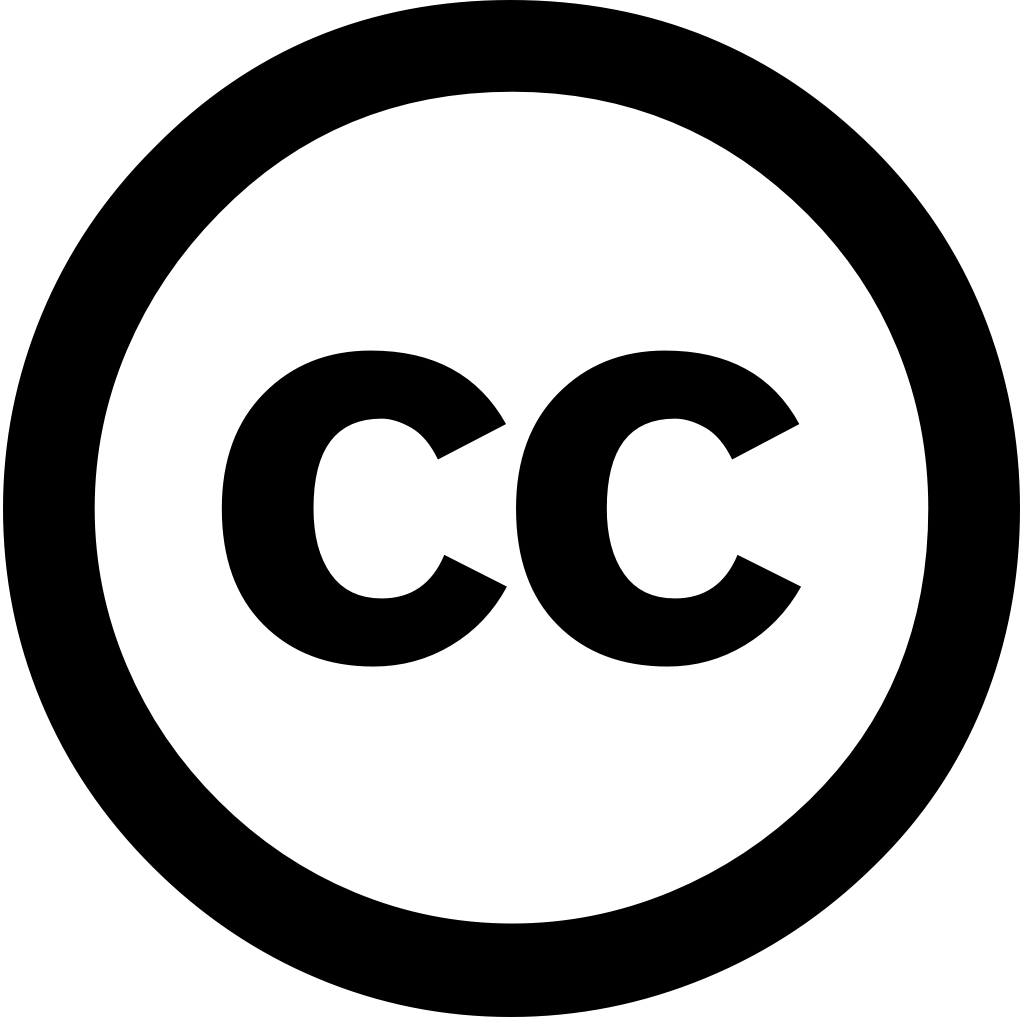
Bioengineering, Journal Year: 2025, Volume and Issue: 12(4), P. 356 - 356
Published: March 29, 2025
According to recent global public health studies, chronic kidney disease (CKD) is becoming more and recognized as a serious risk many people are suffering from this disease. Machine learning techniques have demonstrated high efficiency in identifying CKD, but their opaque decision-making processes limit adoption clinical settings. To address this, study employs generative adversarial network (GAN) handle missing values CKD datasets utilizes few-shot techniques, such prototypical networks model-agnostic meta-learning (MAML), combined with explainable machine predict CKD. Additionally, traditional models, including support vector machines (SVM), logistic regression (LR), decision trees (DT), random forests (RF), voting ensemble (VEL), applied for comparison. unravel the “black box” nature of predictions, various AI, SHapley Additive exPlanations (SHAP) local interpretable explanations (LIME), understand predictions made by model, thereby contributing process significant parameters diagnosis Model performance evaluated using predefined metrics, results indicate that models integrated GANs significantly outperform techniques. Prototypical achieve highest accuracy 99.99%, while MAML reaches 99.92%. Furthermore, attain F1-score, recall, precision, Matthews correlation coefficient (MCC) 99.89%, 99.9%, 100%, respectively, on raw dataset. As result, experimental clearly demonstrate effectiveness suggested method, offering reliable trustworthy model classify This framework supports objectives Medical Internet Things (MIoT) enhancing smart medical applications services, enabling accurate prediction detection facilitating optimal making.
Language: Английский