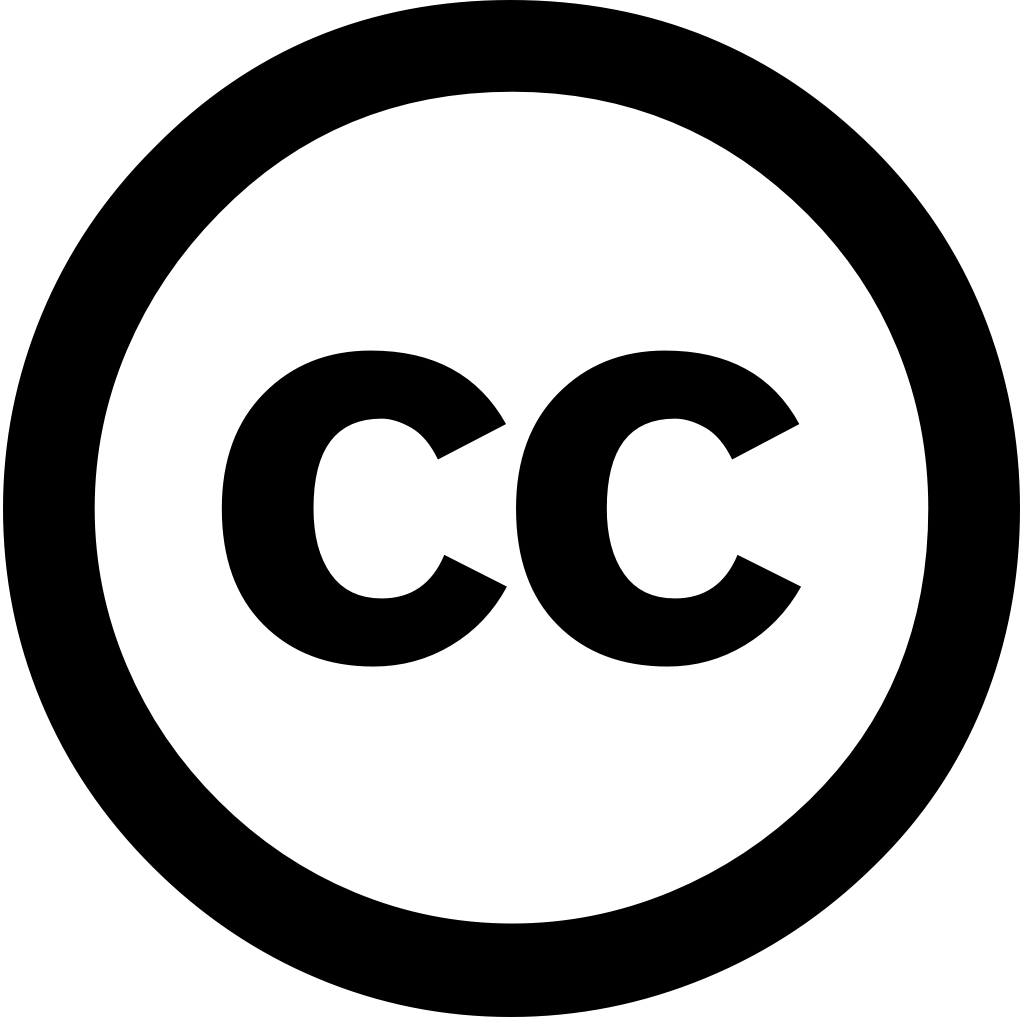
Agriculture, Journal Year: 2025, Volume and Issue: 15(7), P. 746 - 746
Published: March 31, 2025
Plant moisture content (PMC) serves as a crucial indicator of crop water status, directly affecting agricultural productivity, product quality, and the effectiveness precision irrigation. Conventional methods for PMC assessment predominantly rely on destructive sampling techniques, which are labor-intensive impede real-time monitoring. This study investigates silage maize cultivated in Hexi region China, leveraging multispectral data acquired via an unmanned aerial vehicle (UAV) to estimate across different phenological stages. A stacked ensemble learning framework was developed, integrating Back Propagation Neural Network (BPNN), Random Forest Regression (RFR), Support Vector (SVR), with Partial Least Squares (PLSR) employed feature fusion. The findings indicate that incorporating vegetation indices into spectral variables significantly improved prediction performance. standalone models demonstrated coefficient determination (R2) values ranging from 0.43 0.69, root mean square error (RMSE) spanning 0.61% 1.43%. In contrast, model exhibited superior accuracy, achieving R2 between 0.61 0.87 RMSE 0.54% 1.38%. methodology offers scalable, non-invasive alternative estimation, facilitating data-driven irrigation optimization regions facing scarcity.
Language: Английский