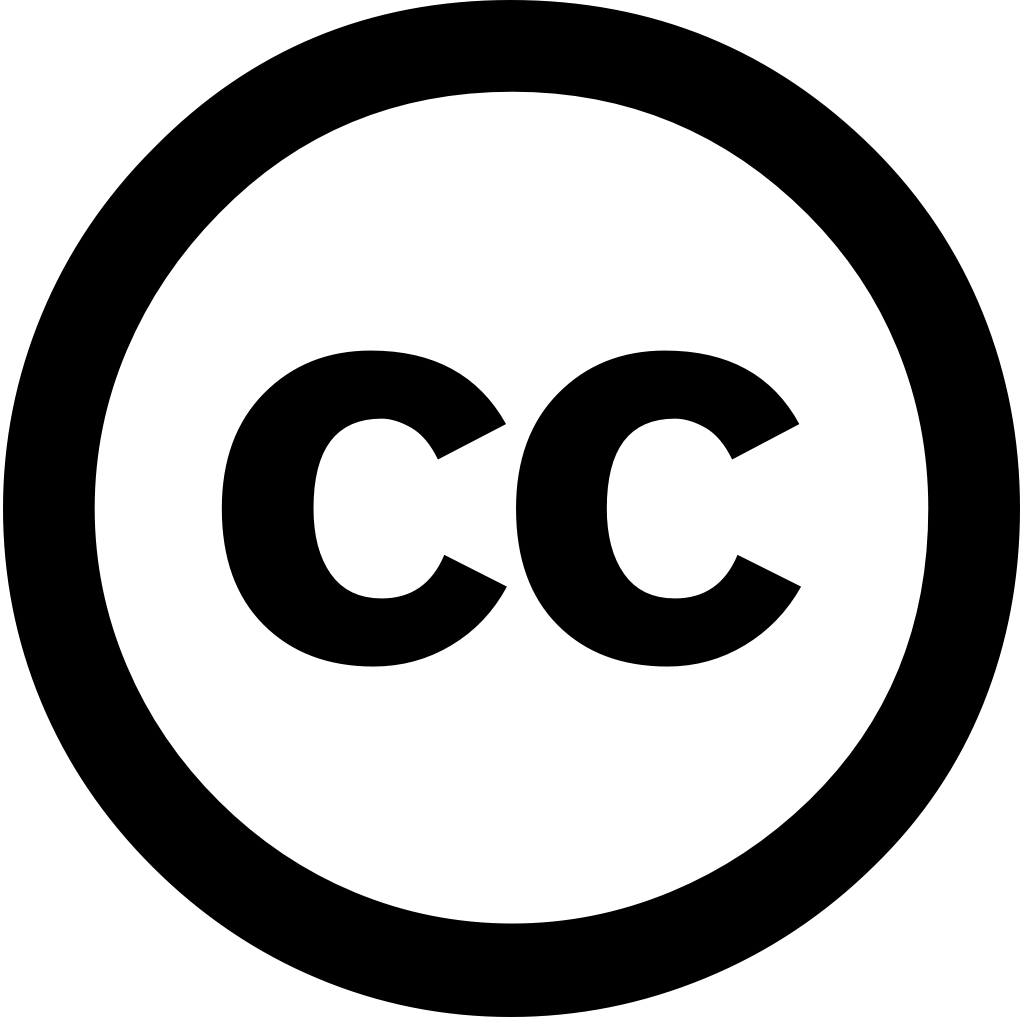
Drones, Journal Year: 2025, Volume and Issue: 9(4), P. 256 - 256
Published: March 27, 2025
Monitoring dust on plant canopies around open-pit coal mines is crucial to assessing environmental pollution and developing effective suppression strategies. This research focuses the Ha’erwusu mine in Inner Mongolia, China, using measured content UAV-borne VNIR hyperspectral data as sources. The study employed five spectral transformation forms—first derivative (FD), second (SD), logarithm (LT), reciprocal (RT), square root (SR)—alongside competitive adaptive reweighted sampling (CARS) method extract characteristic bands associated with canopy dust. Various regression models, including extreme learning machine (ELM), random forest (RF), partial least squares (PLSR), support vector (SVM), were utilized establish inversion models. spatial distribution of was then analyzed. results demonstrate that geometric radiometric correction images successfully restored true information features. transformations significantly enhance feature for CARS algorithm extracted representing 20 30% total bands, evenly spread across entire range, thereby reducing estimation model’s computational complexity. Both extraction model selection influence accuracy, LT-CARS RF combination offering best predictive performance. Canopy decreases increasing distance from source. These findings offer valuable insights retention monitoring a solid foundation management development
Language: Английский