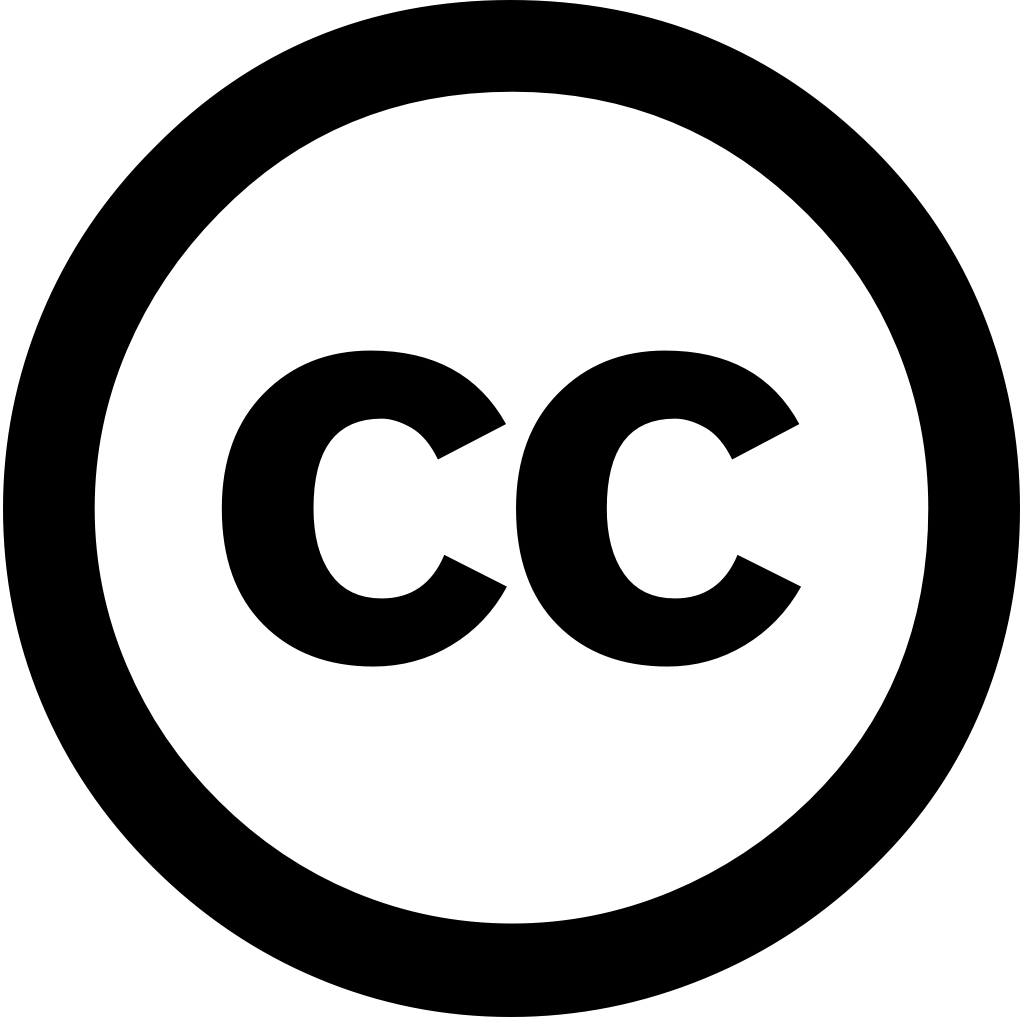
Applied Sciences, Journal Year: 2025, Volume and Issue: 15(9), P. 4652 - 4652
Published: April 23, 2025
This study explores the potential of using convolutional neural networks (CNNs) to diagnose dementia early and manage it in an individualized way. Segmented brain magnetic resonance imaging (MRI) images from Alzheimer’s Disease Neuroimaging Initiative (ADNI) database represented disease (AD), mild cognitive impairment (MCI), cognitively normal (CN) subjects. These classes served train, validate, test CNN-based models. The first four models were developed entirely scratch, other employed transfer learning (TL). While both approaches demonstrated high classification accuracy (93.69% on average), TL-based outperformed independently ones, achieving 97.64% compared with 89.75%. yielded information about detected type, diagnosis confidence level, gradient-weighted class activation mapping (Grad-CAM)-generated heatmaps highlighting pathologically affected regions. results indicate for enhancing detection differentiation offer a promising basis developing deep (DL)-based clinical decision support systems (CDSSs). Such could assist healthcare professionals reducing time, optimizing patient-tailored management treatment strategies, improving quality life individuals dementia.
Language: Английский