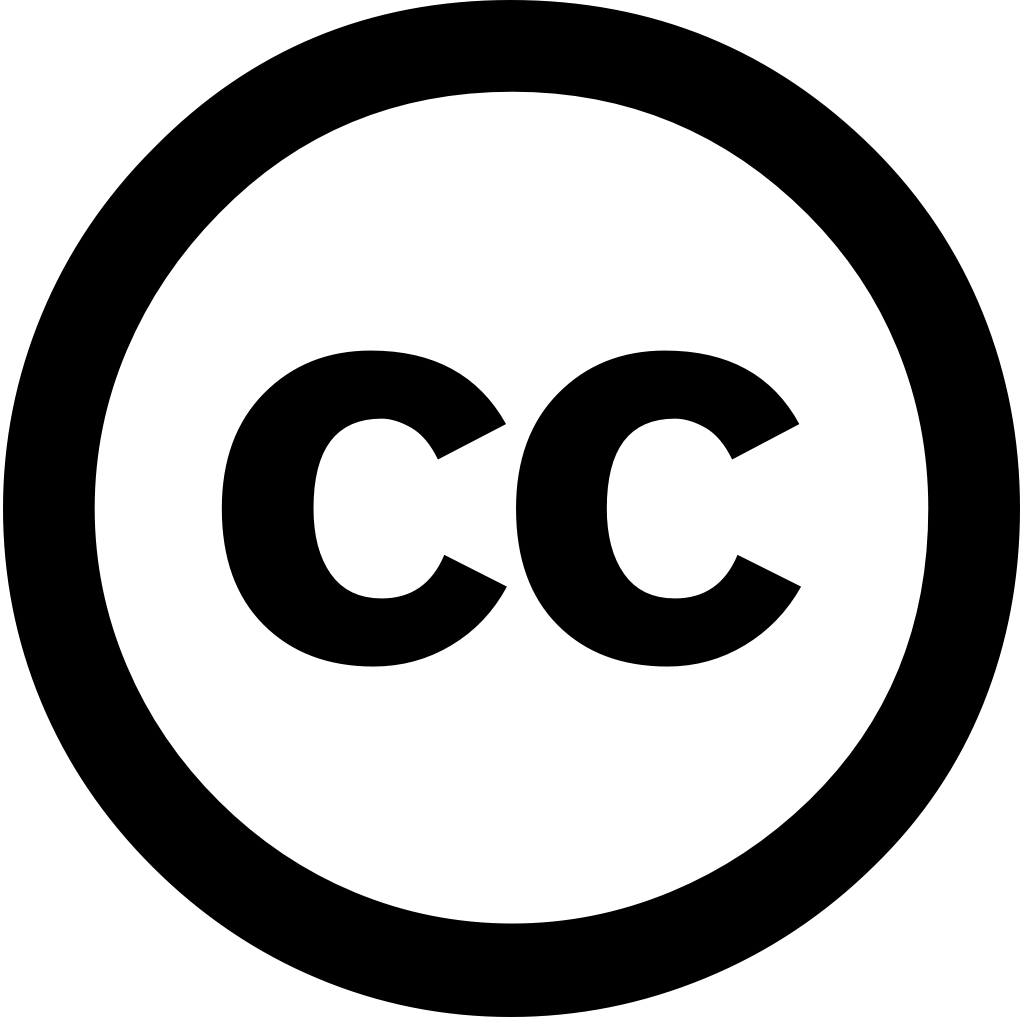
Health care science, Journal Year: 2025, Volume and Issue: 4(2), P. 110 - 143
Published: April 1, 2025
Deep learning (DL) has become the prevailing method in chest radiograph analysis, yet its performance heavily depends on large quantities of annotated images. To mitigate cost, cold-start active (AL), comprising an initialization followed by subsequent learning, selects a small subset informative data points for labeling. Recent advancements pretrained models supervised or self-supervised tailored to have shown broad applicability diverse downstream tasks. However, their potential AL remains unexplored. validate efficacy domain-specific pretraining, we compared two foundation models: TXRV and REMEDIS with general domain counterparts ImageNet. Model was evaluated at both stages diagnostic tasks: psychiatric pneumonia COVID-19. For initialization, assessed integration three strategies: diversity, uncertainty, hybrid sampling. focused uncertainty sampling powered different models. We also conducted statistical tests compare ImageNet counterparts, investigate relationship between examine one-shot against full process, influence class balance samples learning. First, failed outperform six out eight experiments sample selection. Both were unable generate representations that could substitute original images as model inputs seven scenarios. model-based surpassed random sampling, default approach AL. Second, positively correlated performance, highlighting importance strategies. Third, performed comparably demonstrating reducing experts' repeated waiting during iterations. Last, U-shaped correlation observed suggesting is more strongly associated middle budget levels than low high budgets. In this study, highlighted limitations medical pretraining context identified promising outcomes related AL, including based models, positive middle-budget Researchers are encouraged improve versatile DL foundations explore novel methods.
Language: Английский