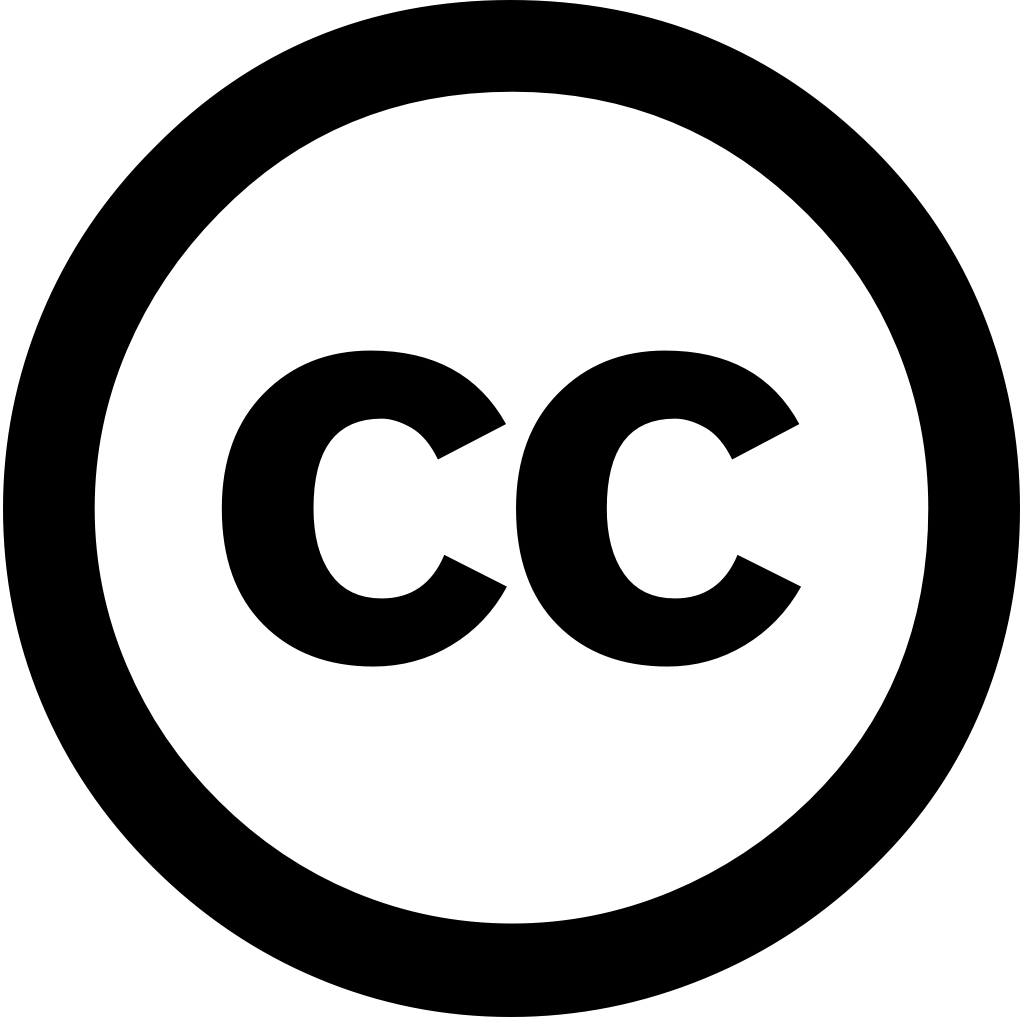
Journal of Mind and Medical Sciences, Journal Year: 2025, Volume and Issue: 12(1), P. 29 - 29
Published: May 4, 2025
Background: The accurate identification of malignant lymph nodes in cervical ultrasound images is crucial for early diagnosis and treatment planning. Traditional evaluation metrics, such as accuracy the Dice Similarity Coefficient (DSC), often fail to provide a realistic assessment segmentation performance, they do not account partial overlaps between predictions ground truth. This study addresses this gap by introducing Intersection over Union (IoU) an additional metric offer more comprehensive model performance. Specifically, we aimed develop convolutional neural network (CNN) capable detecting suspicious assess its effectiveness using both conventional IoU-based performance metrics. Methods: A dataset consisting 992 node was extracted from 166 scans labeled ImgLab annotation tool. CNN developed Python, Keras, TensorFlow employed within Jupyter Notebook environment. architecture consists four layers trained distinguish nodes. Results: achieved training 97% validation 99%. DSC score 0.984, indicating strong although it limited positive cases. An IoU applied test revealed average overlap 74% ground-truth labels predictions, offering nuanced measure accuracy. Conclusions: demonstrated high scores, confirming identifying However, values, while lower than provided model’s highlighting areas potential improvement underscores importance alongside traditional metrics obtain reliable deep learning-based medical image analysis models.
Language: Английский