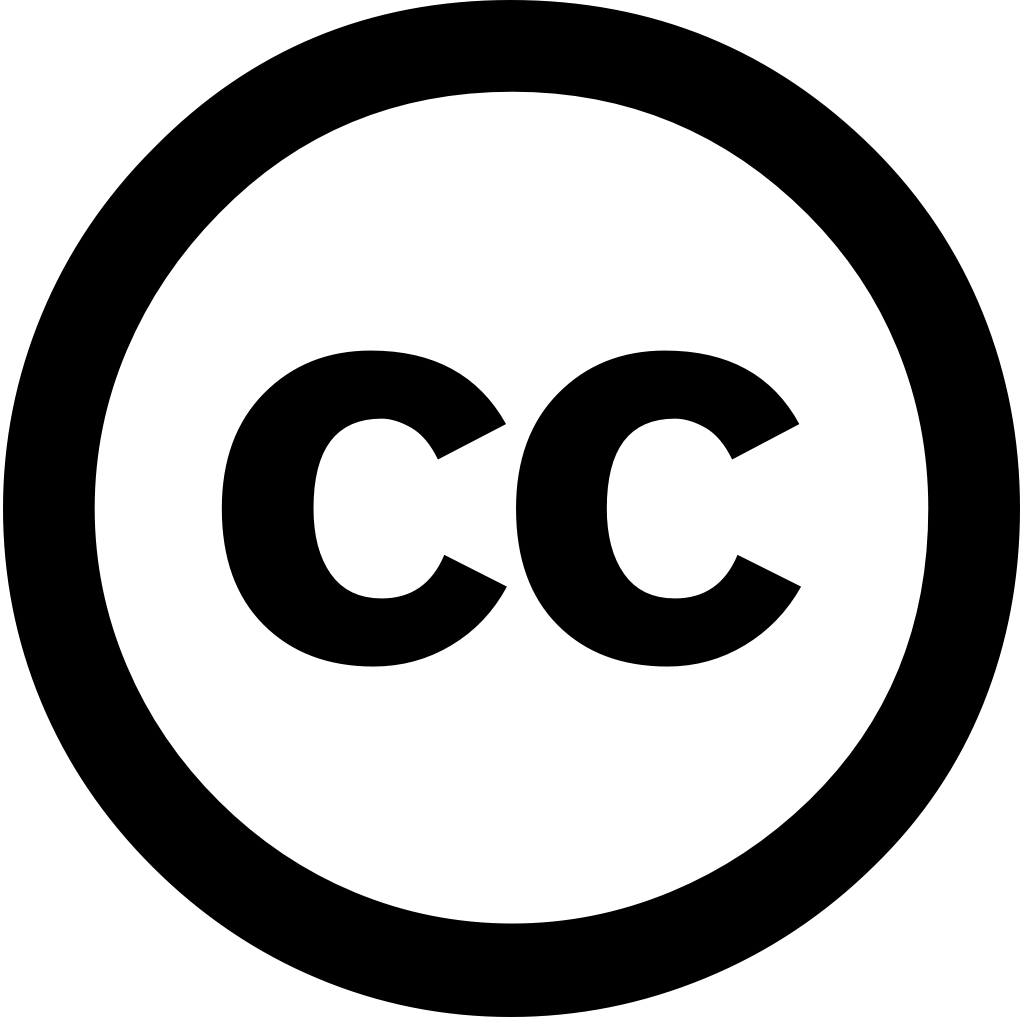
Frontiers in Materials, Journal Year: 2025, Volume and Issue: 12
Published: April 8, 2025
Introduction Deep learning has significantly advanced medical image analysis, enabling precise feature extraction and pattern recognition. However, its application in computational material science remains underexplored, despite the increasing need for automated microstructure analysis defect detection. Traditional processing methods often rely on handcrafted threshold-based segmentation, which lack adaptability to complex microstructural variations. Conventional machine approaches struggle with data heterogeneity extensive labeled datasets. Methods To overcome these limitations, we propose a deep learning-driven framework that integrates convolutional neural networks (CNNs) transformer-based architectures enhanced representation. Our method incorporates domain-adaptive transfer multi-modal fusion techniques improve generalizability of analysis. Results Experimental evaluations diverse datasets demonstrate superior performance segmentation accuracy, detection robustness, efficiency compared traditional methods. Discussion By bridging gap between science, our approach contributes more effective, automated, scalable characterization processes.
Language: Английский