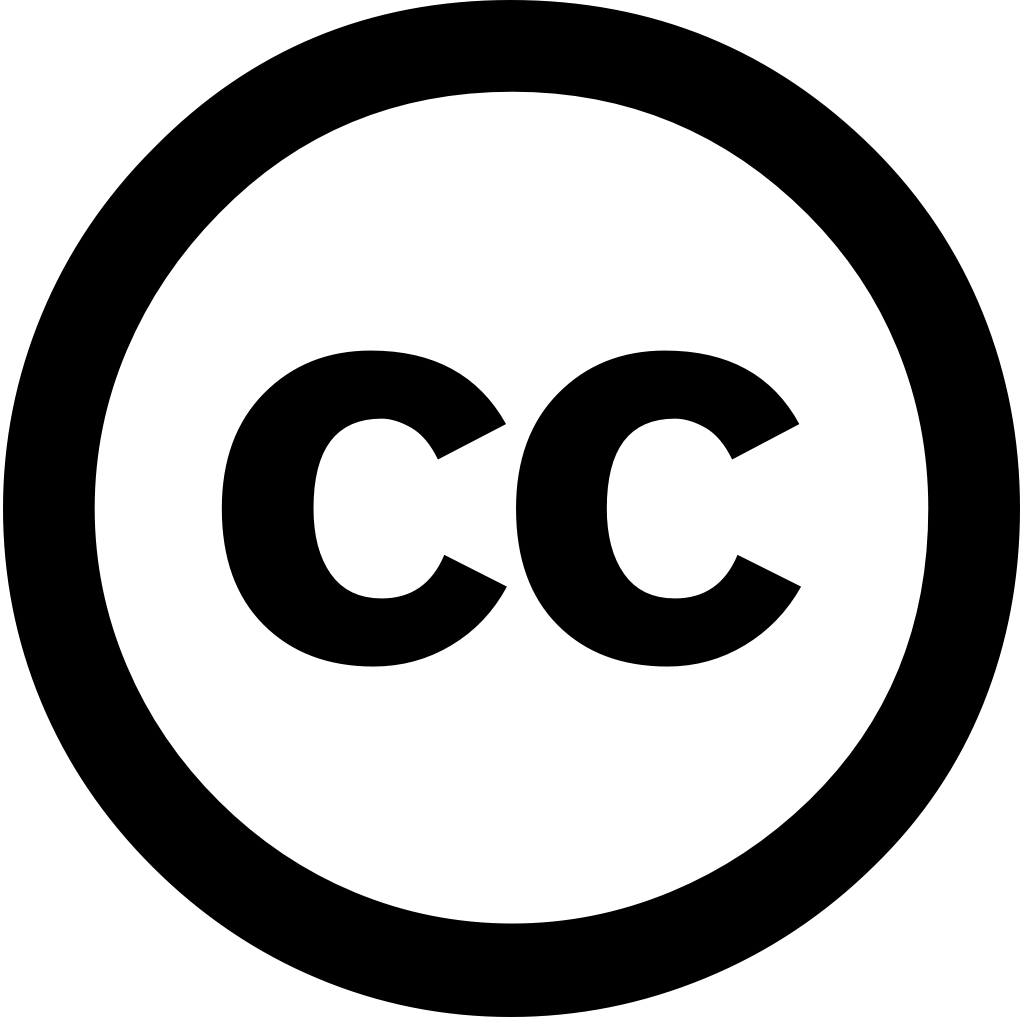
Applied Sciences, Journal Year: 2025, Volume and Issue: 15(6), P. 3071 - 3071
Published: March 12, 2025
Hearing loss significantly impacts daily communication, making accurate speech audiometry (SA) assessment essential for diagnosis and treatment. However, SA testing is time-consuming resource-intensive, limiting its accessibility in clinical practice. This study aimed to develop a multi-class classification model that predicts results using pure-tone (PTA) data, enabling more efficient automated assessment. To achieve this, we implemented compared MLP, RNN, gradient boosting, XGBoost models, evaluating their performance accuracy, F1 score, log loss, confusion matrix analysis. Experimental showed boosting achieved the highest 86.22%, while demonstrated balanced performance. The MLP 85.77% RNN 85.41%, exhibiting relatively low with showing limitations due temporal dependency of PTA data. Additionally, all models faced challenges predicting class 2 (borderline hearing levels) overlapping data distributions. These findings suggest machine learning particularly XGBoost, outperform deep prediction. Future research should focus on feature engineering, hyperparameter optimization, ensemble approaches enhance validate real-world applicability. proposed could contribute automating prediction improving efficiency patient care.
Language: Английский