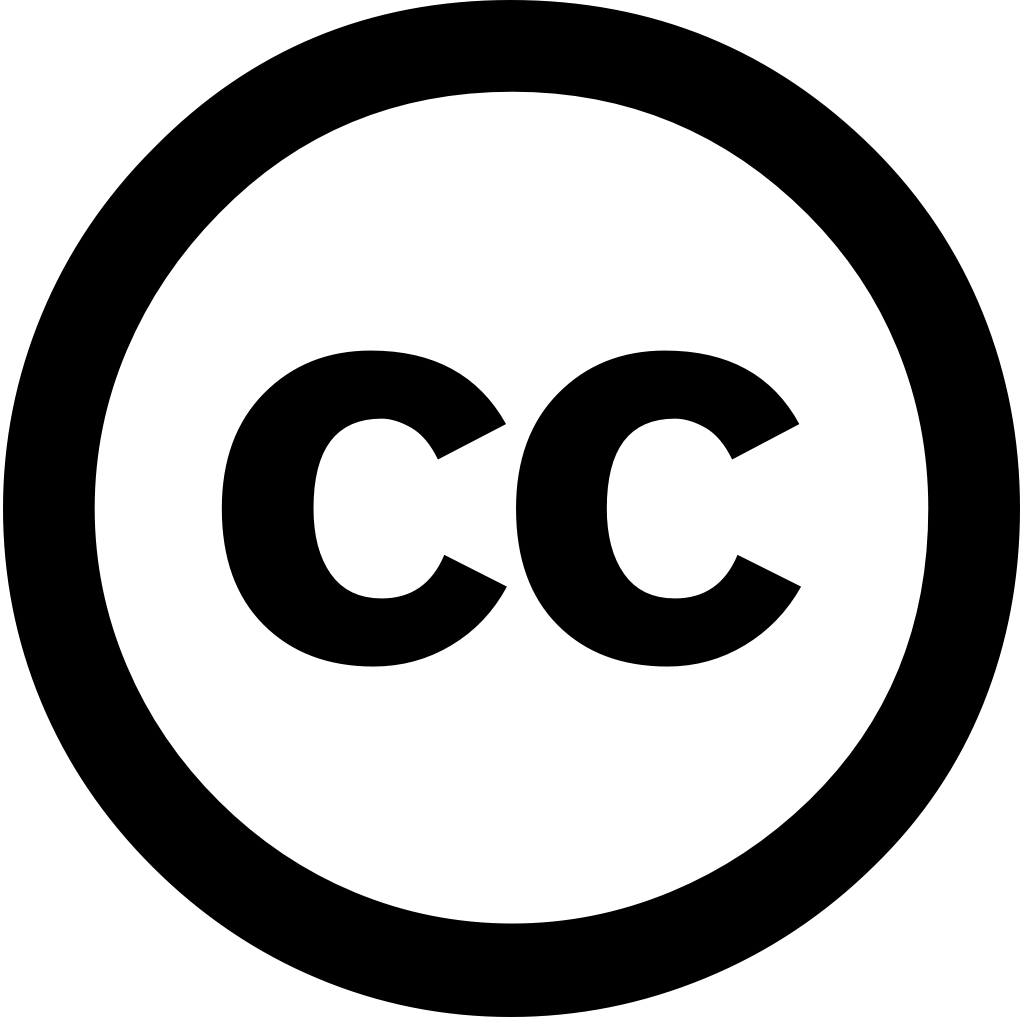
AIP Advances, Journal Year: 2025, Volume and Issue: 15(4)
Published: April 1, 2025
This study introduces a novel approach for predicting the mechanical properties of 3D-printed polylactic acid wood composites using gene expression programming (GEP) and artificial neural networks (ANN) modeling methods. Addressing challenge determining optimal process parameters in fused deposition natural fiber composites, experiments were designed Taguchi’s L27 orthogonal array. Five key analyzed: layer thickness (100–300 μm), printing speed (40–90 mm/s), raster angle (0°–90°), infill density (35%–95%), nozzle temperature (200–220 °C). ANOVA results identified as most influential factor, contributing 38.36% 26% to tensile compressive strengths, respectively. Subsequently, comparative statistical analysis evaluated predictive accuracy GEP ANN. The model exhibited superior performance, achieving validation errors between 0.04% 0.82%, outperforming ANN (0.34%–5.31%). These findings provide robust framework enhancing performance sustainable enabling more efficient reliable production processes additive manufacturing.
Language: Английский