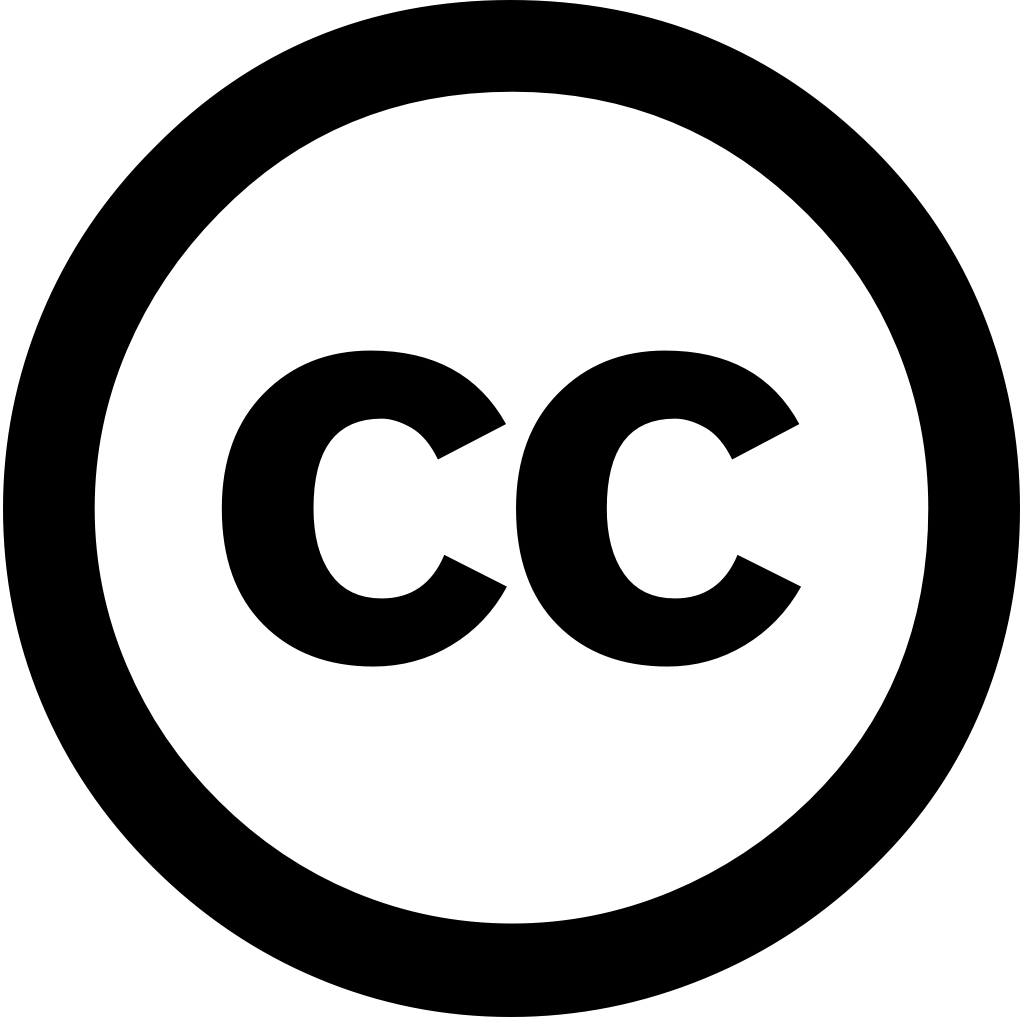
Molecular Biology and Evolution, Journal Year: 2024, Volume and Issue: 42(1)
Published: Dec. 27, 2024
Determining the impact of mutations on thermodynamic stability proteins is essential for a wide range applications such as rational protein design and genetic variant interpretation.Since major driver evolution, evolutionary data are often used to guide predictions. Many state-of-the-art predictors extract information from multiple sequence alignments (MSA) homologous query protein, leverage it predict effects stability. To evaluate power limitations methods, we massive amount recently obtained by deep mutational scanning study how best construct MSAs optimally them. We tested different models found that, unexpectedly, independent-site achieve similar accuracy more complex epistatic models. A detailed analysis latter suggests that their inference results in noisy couplings, which do not appear add predictive over contribution, at least context prediction. Interestingly, combining any features with simple structural feature, relative solvent accessibility mutated residue, achieved prediction supervised, machine learning-based, change predictors. Our provide new insights into relationship between evolution stability, show can be exploited improve performance
Language: Английский