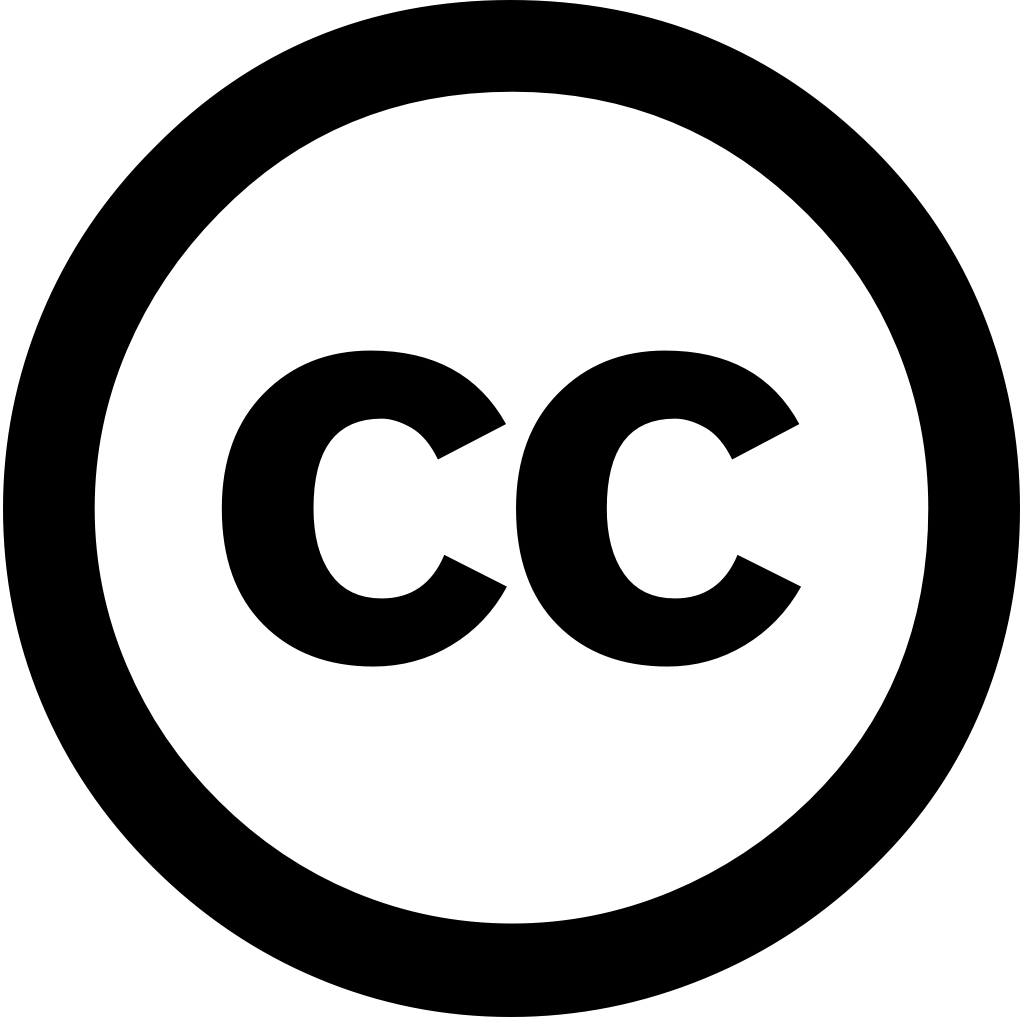
AgriEngineering, Journal Year: 2025, Volume and Issue: 7(4), P. 103 - 103
Published: April 3, 2025
Accurate weed segmentation in Unmanned Aerial Vehicle (UAV) imagery remains a significant challenge precision agriculture due to environmental variability, weak contextual representation, and inaccurate boundary detection. To address these limitations, we propose the Multi-Scale Edge-Aware Network (MSEA-Net), lightweight efficient deep learning framework designed enhance accuracy while maintaining computational efficiency. Specifically, introduce Spatial-Channel Attention (MSCA) module recalibrate spatial channel dependencies, improving local–global feature fusion reducing redundant computations. Additionally, Edge-Enhanced Bottleneck (EEBA) integrates Sobel-based edge detection refine delineation, ensuring sharper object separation dense vegetation environments. Extensive evaluations on publicly available datasets demonstrate effectiveness of MSEA-Net, achieving mean Intersection over Union (IoU) 87.42% Motion-Blurred UAV Images Sorghum Fields dataset 71.35% CoFly-WeedDB dataset, outperforming benchmark models. MSEA-Net also maintains compact architecture with only 6.74 M parameters model size 25.74 MB, making it suitable for UAV-based real-time segmentation. These results highlight potential automated efficiency deployment.
Language: Английский