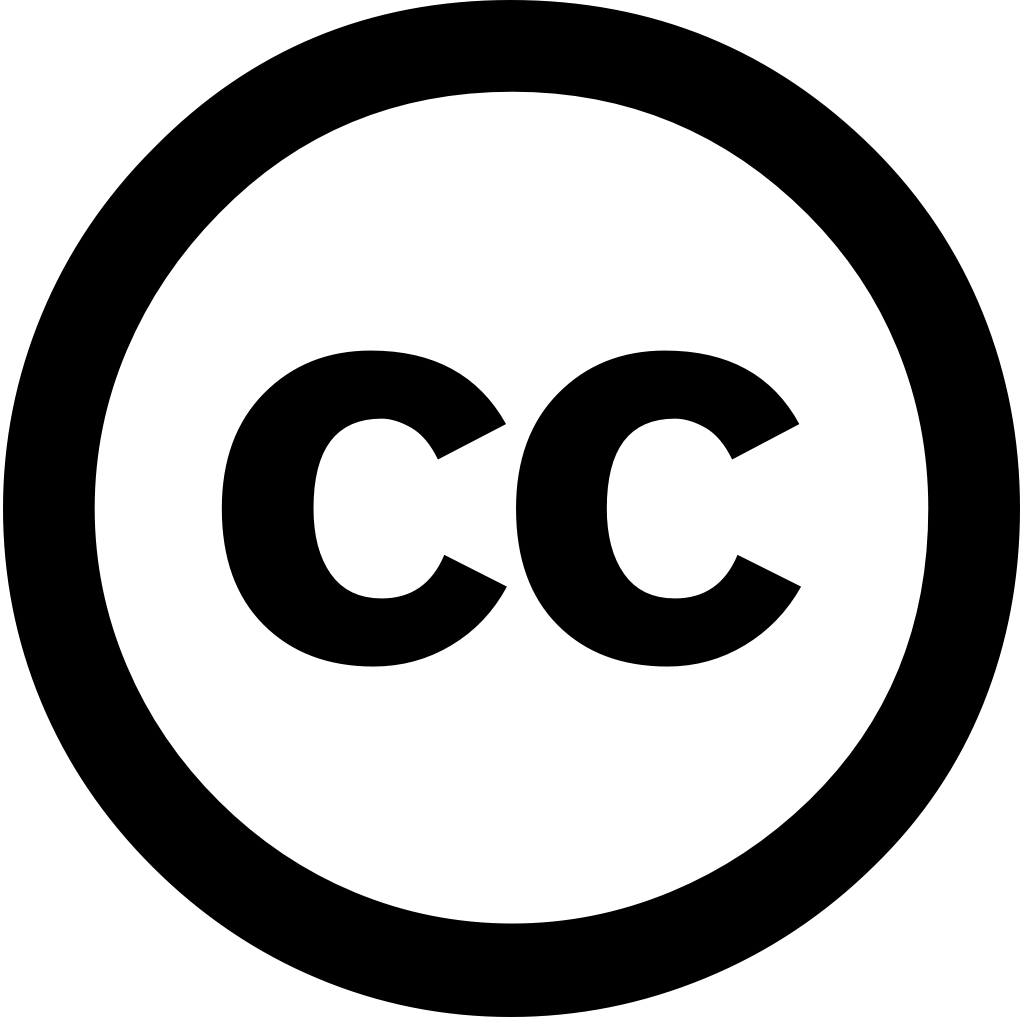
Materials Genome Engineering Advances, Journal Year: 2024, Volume and Issue: unknown
Published: Dec. 8, 2024
Abstract Although the power conversion efficiency of organic solar cells (OSCs) has been rapidly improved, there is still a lot room for designing and developing new materials their combinations to approach limit. In this work, we establish database ∼100 bulk heterojunction OSCs composed representative donors acceptors reported in literature, train machine learning models identify potential donor‐acceptor combinations. We find that fully connected neural network achieves Pearson coefficient up 0.88 predicting with different acceptors. use sure independence screening sparsifying method feature analysis analyze evaluate performance OSCs. To prove reliability viability predictive model, introduce theoretical limits confidence tests into process, which provides simple but reliable solution quickly OSC material
Language: Английский