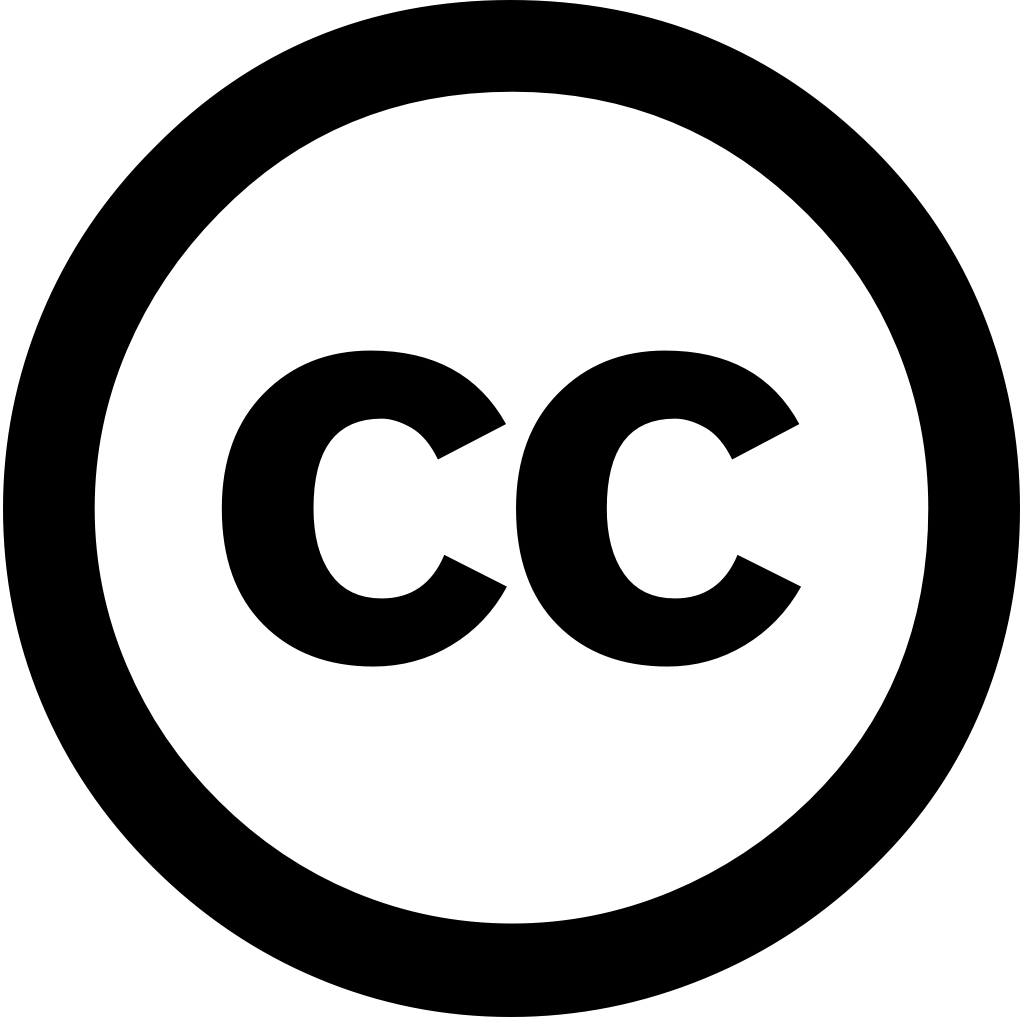
Structures, Journal Year: 2024, Volume and Issue: 71, P. 107917 - 107917
Published: Dec. 3, 2024
Language: Английский
Structures, Journal Year: 2024, Volume and Issue: 71, P. 107917 - 107917
Published: Dec. 3, 2024
Language: Английский
Journal of Building Engineering, Journal Year: 2024, Volume and Issue: 88, P. 109184 - 109184
Published: April 3, 2024
Language: Английский
Citations
23Scientific Reports, Journal Year: 2023, Volume and Issue: 13(1)
Published: Feb. 17, 2023
Abstract The ability of machine learning (ML) techniques to forecast the shear strength corroded reinforced concrete beams (CRCBs) is examined in present study. These ML include artificial neural networks (ANN), adaptive-neuro fuzzy inference systems (ANFIS), decision tree (DT) and extreme gradient boosting (XGBoost). A thorough databank with 140 data points about capacity CRCBs various degrees corrosion was compiled after a review literature. inputs parameters implemented models are width beam, effective depth compressive (CS), yield reinforcement, percentage longitudinal transversal reinforcement (stirrups), stirrups, stirrups spacing, span-to-depth ratio ( a/d ), degree main stirrups. coefficient determination ANN, ANFIS, DT, XGBoost 0.9811, 0.9866, 0.9799, 0.9998, respectively. MAPE model 99.39%, 99.16%, 99.28% lower than DT models. According results sensitivity examination, most affected by . graphical displays Taylor graph, violin plot, multi-histogram plot additionally support model's dependability precision. In addition, this demonstrated good experimental fit when compared other analytical Accurate prediction using approach confirmed that capable handling wide range can be used as predict higher accuracy. effectiveness developed existing terms precision, economic considerations, safety, indicated comparative
Language: Английский
Citations
23Journal of Building Engineering, Journal Year: 2024, Volume and Issue: 90, P. 109418 - 109418
Published: April 23, 2024
Using fiber-reinforced polymer (FRP) in reinforced concrete (RC) structures can mitigate the colossal repair costs due to reinforcing steel corrosion. Hence, FRP rod/bar is gaining wider applications diverse RC as a partial or full replacement for rebar. The rod-to-concrete interfacial bond pivotal transferring stresses from rods. This study develops novel prediction model estimate near surface mounted strength well failure type using five machine learning (ML) algorithms, namely, linear regression, decision tree, gradient boosting random forest, and extreme (XGB). performance of developed models was compared with that four design guidelines one analytical model. A database comprising 416 experimental datasets constructed used training validation. Based on statistical metrics, precision XGB algorithm superior other ML models, guidelines, Feature importance analysis based SHapley Additive exPlanations theory dependence plot performed. results show length had most significant influence strength, followed by tensile composite, diameter rod, compressive concrete, elastic modulus composite. graphical user interface offers friendly, free access, simple tool estimating rods concrete.
Language: Английский
Citations
10Scientific Reports, Journal Year: 2024, Volume and Issue: 14(1)
Published: Jan. 21, 2024
Language: Английский
Citations
9IEEE Access, Journal Year: 2022, Volume and Issue: 10, P. 121204 - 121229
Published: Jan. 1, 2022
In
this
paper,
curve-fitting
and
an
artificial
neural
network
(ANN)
model
were
developed
to
predict
R-Event.
Expected
number
of
new
infections
that
arise
in
any
event
occurring
over
a
total
time
space
is
termed
as
Real-time
data
for
the
office
environment
was
gathered
spring
2022
naturally
ventilated
room
Roorkee,
India,
under
composite
climatic
conditions.
To
ascertain
merit
proposed
ANN
models,
performances
approach
compared
against
curve
fitting
regarding
conventional
statistical
indicators,
i.e.,
correlation
coefficient,
root
mean
square
error,
absolute
Nash-Sutcliffe
efficiency
index,
percentage
a20-index.
Eleven
input
parameters
namely
indoor
temperature
(
Language: Английский
Citations
31Buildings, Journal Year: 2023, Volume and Issue: 13(4), P. 931 - 931
Published: March 31, 2023
The degradation of reinforced concrete (RC) structures has raised major concerns in the industry. demolition existing shown to be an unsustainable solution and leads many financial concerns. Alternatively, strengthening sector put forward sustainable solutions, such as retrofitting rehabilitation structural elements with fiber-reinforced polymer (FRP) composites. Over past four decades, FRP retrofits have attracted attention from scientific community, thanks their numerous advantages having less weight, being non-corrodible, etc., that help enhance axial, flexural, shear capacities RC members. This study focuses on predicting compressive strength (CS) FRP-confined cylinders using analytical models machine learning (ML) models. To achieve this, a total 1151 specimens been amassed comprehensive literature studies. ML utilized are Gaussian process regression (GPR), support vector (SVM), artificial neural network (ANN), optimized SVM, GPR input parameters used for prediction include geometrical characteristics specimens, mechanical properties composite, CS concrete. results five compared nineteen evaluated algorithms imply model found best among all other models, demonstrating higher correlation coefficient, root mean square error, absolute percentage a-20 index, Nash–Sutcliffe efficiency values 0.9960, 3.88 MPa, 3.11%, 2.17 0.9895, 0.9921, respectively. R-value is 0.37%, 0.03%, 5.14%, 2.31% than ANN, GPR, SVM respectively, whereas error value is, 81.04%, 12.5%, 471.77%, 281.45% greater model.
Language: Английский
Citations
17Applied Sciences, Journal Year: 2024, Volume and Issue: 14(9), P. 3601 - 3601
Published: April 24, 2024
In recent years, machine learning models have become a potential approach in accurately predicting the concrete compressive strength, which is essential for real-world application of geopolymer concrete. However, precursor system known to be more heterogeneous compared Ordinary Portland Cement (OPC) concrete, adversely affecting data generated and performance models. To its advantage, enrichment through deep can effectively enhance prediction Therefore, this study investigates capability tabular generative adversarial networks (TGANs) generate on mixtures strength It assesses impact using synthetic with various models, including tree-based, support vector machines, neural networks. For purpose, 930 instances 11 variables were collected from open literature. particular, 10 content fly ash, slag, sodium silicate, hydroxide, superplasticizer, fine aggregate, coarse added water, curing temperature, specimen age are considered as inputs, while output A TGAN was employed an additional 1000 points based original dataset training new predictive These evaluated real test sets trained data. The results indicate that developed significantly improve performance, particularly networks, followed by tree-based machines. Moreover, characteristics greatly influence model both before after augmentation.
Language: Английский
Citations
7Materials, Journal Year: 2022, Volume and Issue: 15(23), P. 8295 - 8295
Published: Nov. 22, 2022
The bond strength between concrete and corroded steel reinforcement bar is one of the main responsible factors that affect ultimate load-carrying capacity reinforced (RC) structures. Therefore, prediction accurate has become an important parameter for safety measurements RC However, analytical models are not enough to estimate strength, as they built using various assumptions limited datasets. machine learning (ML) techniques named artificial neural network (ANN) support vector (SVM) have been used bar. considered input parameters in this research surface area specimen, cover, type bars, yield compressive diameter length, water/cement ratio, corrosion level bars. These were build ANN SVM models. reliability developed compared with twenty Moreover, analyzed results revealed precision efficiency higher radar plot Taylor diagrams also utilized show graphical representation best-fitted model. proposed model best model, a correlation coefficient 0.99, mean absolute error 1.091 MPa, root square 1.495 MPa. Researchers designers can apply precisely steel-to-concrete strength.
Language: Английский
Citations
26Nondestructive Testing And Evaluation, Journal Year: 2024, Volume and Issue: unknown, P. 1 - 28
Published: April 16, 2024
The flyash-based geopolymer concrete has been extensively utilized to minimize carbon emissions for over 15 years. compressive strength (CS), split tensile (STS), and flexural (FS) of determine its mechanical characteristics. Laboratory testing analytical methods is costly time-consuming, which struggle predict complicated combination CS, STS, FS. ML models accurately To the FS GPC concrete, five techniques (LR, SVM, GPR, EL, ANN) were adopted. Taylor diagrams error graphs adopted represents model performances. GPR outperforms other in CS predictions by having lowest MAE, RMSE, MAPE, R-values. continues outperform STS predictions, with low as well high SVM excellent since it a MAE RMSE. ANN optimisations keep them competitive. consistently gets MAPE values across all three tests, indicating that strong contender. created can forecast characteristics ease better reproducibility.
Language: Английский
Citations
5Buildings, Journal Year: 2022, Volume and Issue: 12(12), P. 2137 - 2137
Published: Dec. 5, 2022
Due to the corrosion problem in reinforced concrete structures, use of fiber-reinforced polymer (FRP) bars may be preferred place traditional reinforcing steel. FRP are used constructions boost strength structural elements and retain their longevity. In this study, axial load carrying capacity (ALCC) FRP-reinforced columns has been evaluated using analytical, as well machine learning, models. A total fourteen popular analytical models one proposed learning-based model were estimate ALCC columns. The learning is based on an artificial neural network (ANN) method. performance ANN, models, assessed six different indices. R-value developed ANN 0.9758, followed by NS value 0.9513. It found that mean absolute percentage error best-fitted 328.71% higher than model, root-mean-square 211.97% model. data demonstrate performs better other quick easy-to-use
Language: Английский
Citations
20