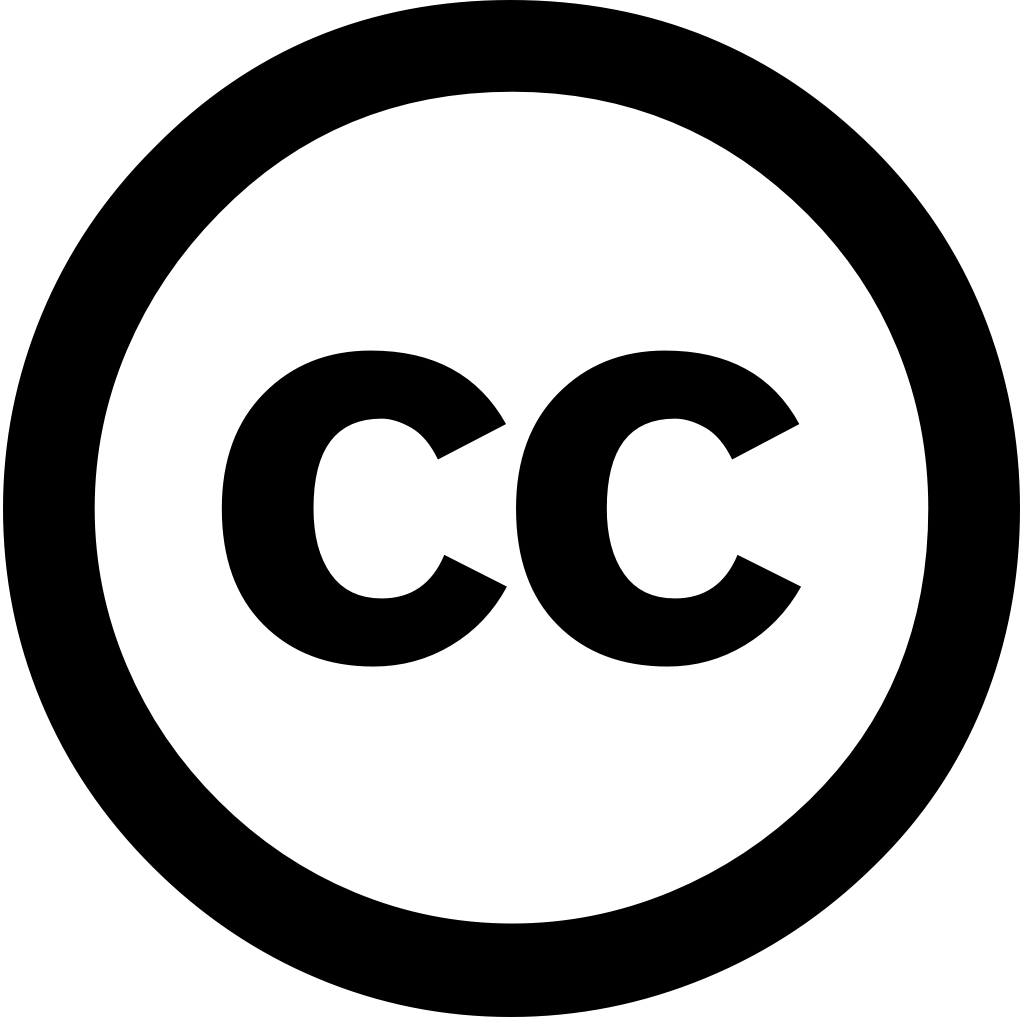
Remote Sensing, Journal Year: 2024, Volume and Issue: 16(21), P. 4086 - 4086
Published: Nov. 1, 2024
An accurate estimation of seasonal spatiotemporal dynamics forage phosphorus (P) content in alpine grassland is crucial for effective and livestock management. In this study, we integrated Sentinel-2 multispectral imagery (MSI) with computational hyperspectral features (CHSFs) developed a novel symbolic regression algorithm based on deep reinforcement learning genetic programming (DRL-GP) to estimate P grasslands. Using 243 field observations collected during the regreening, grass-bearing, yellowing periods 2023 from Shaliu River Basin, generated 10 CHSF images (CHSFIs) varying spectral dispersions (1–10 nm). Our results demonstrated following: (1) The DRL-GP-based model identified optimal dispersion each growing season, significantly enhancing accuracy. (2) Forage estimations using combined outperformed traditional methods. Compared original features, R2 improved by 99.5%, 57.4%, 86.2% periods, corresponding MSE reductions 84.8%, 41.5%, 75.8% MAE decreases 70.7%, 57.5%, 50.4%. Across these seasons, increased 322.2%, 68.2%, 639.8% compared MLR, 128.9%, 97.4%, 469.2% RF, 485.1%, 65.3%, 231.3% DNN. decreased 31%, 82.9%, 52.4% 39.9%, 42.4%, 31.4% 84.5%, 73.4%, 81.9% 32.6%, 67%, 44.2% 42.6%, 47.6%, 37.9% 60.2%, 50%, 56.3% (3) Proximity water system notably influenced variation, highest increases observed within 1–2 km sources. These findings provide critical insights optimizing management improving productivity.
Language: Английский