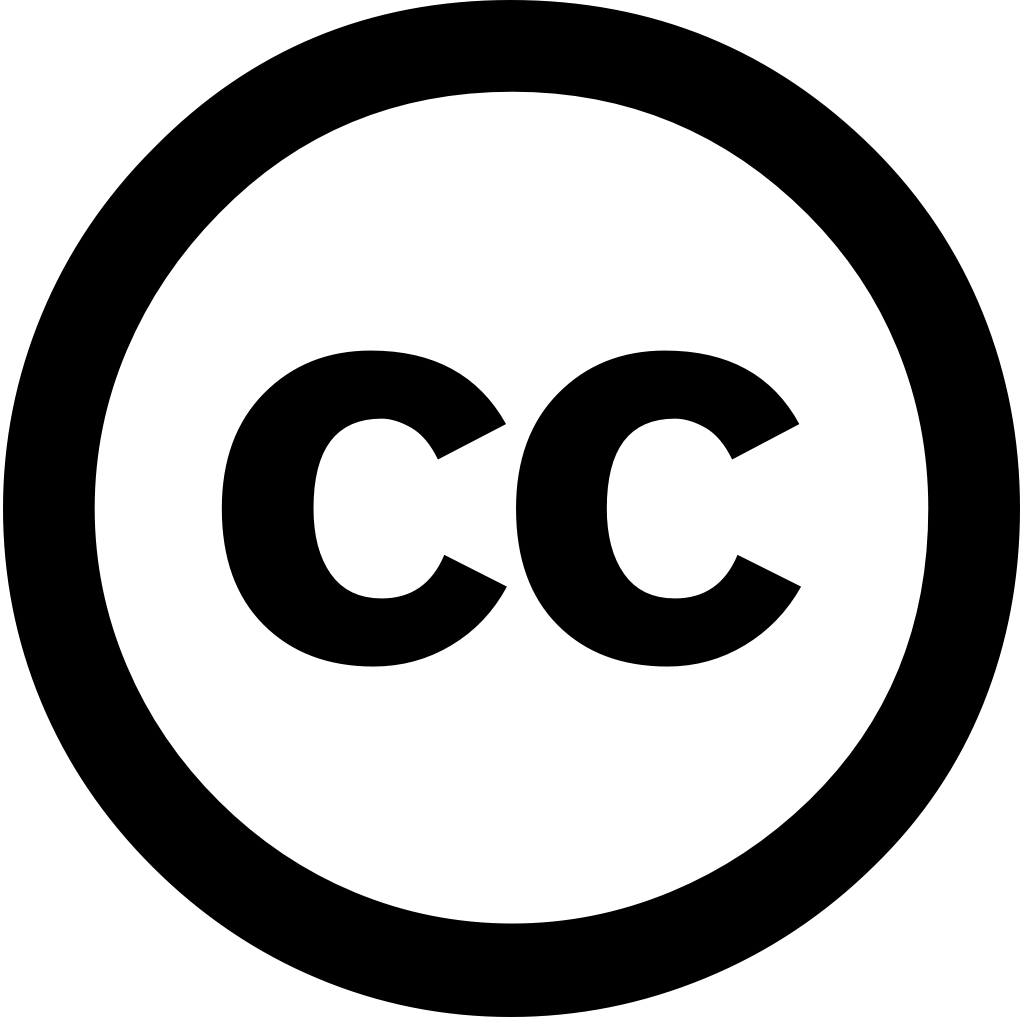
Machine Learning Science and Technology, Journal Year: 2024, Volume and Issue: 5(2), P. 025047 - 025047
Published: May 15, 2024
Abstract Precise liver tumors and associated organ segmentation hold immense value for surgical radiological intervention, enabling anatomical localization pre-operative planning intra-operative guidance. Modern deep learning models medical image have evolved from convolution neural networks to transformer architectures, significantly boosting global context understanding. However, accurate delineation especially of hepatic lesions remains an enduring challenge due models’ predominant focus solely on spatial feature extraction failing adequately characterize complex anatomies. Moreover, the relative paucity expertly annotated imaging data restricts model exposure diverse pathological presentations. In this paper, we present a three-phrased cascaded framework featuring X-Fuse that synergistically integrates frequency domain’s complementary information in dual encoders enrich latent representation. To enhance generalizability, building upon topology taking advantage additional unlabeled data, our proposed integration curriculum pseudo-labeling with Jensen–Shannon variance-based uncertainty rectification promotes optimized pseudo supervision semi-supervised learning. We further introduce tumor-focus augmentation technique including training-free copy-paste knowledge-based synthesis show efficacy simplicity, contributing substantial elevation adaptability lesional morphologies. Extensive experiments modular evaluations holdout test set demonstrate methods outperform existing state-of-the-art both supervised settings, as measured by Dice similarity coefficient, achieving superior bones (95.42%), (96.26%), (89.53%) 16.41% increase comparing V-Net supervised-only augmented-absent scenario. Our method marks significant step toward realization more reliable robust AI-assisted diagnostic tools tumor intervention. made codes publicly available [ https://github.com/lyupengju/X-Fuse ].
Language: Английский