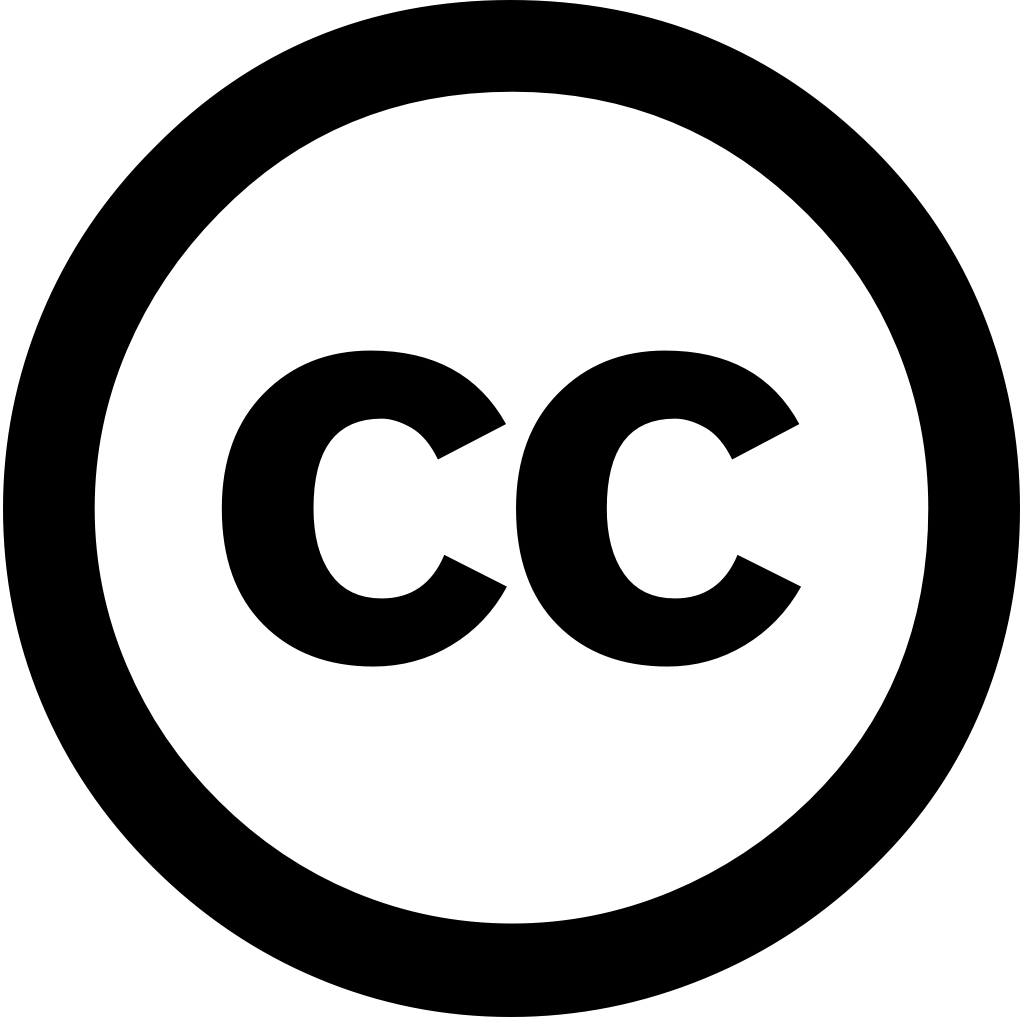
bioRxiv (Cold Spring Harbor Laboratory), Journal Year: 2025, Volume and Issue: unknown
Published: Feb. 4, 2025
Abstract Neural circuits must balance plasticity and stability to enable continual learning without catastrophic forgetting, a pervasive feature of artificial neural networks trained using end-to-end (e.g. backpropagation). Here, we apply an alternative, hierarchical algorithm the cognitive task boundary detection in video clips. In contrast backpropagation, training converges network executing fixed schema generates firing statistics consistent with single-neuron recordings from human subjects performing same task. The hierarchically network’s circuit remains invariant following on sparse data, additional data serving refine upstream representation.
Language: Английский