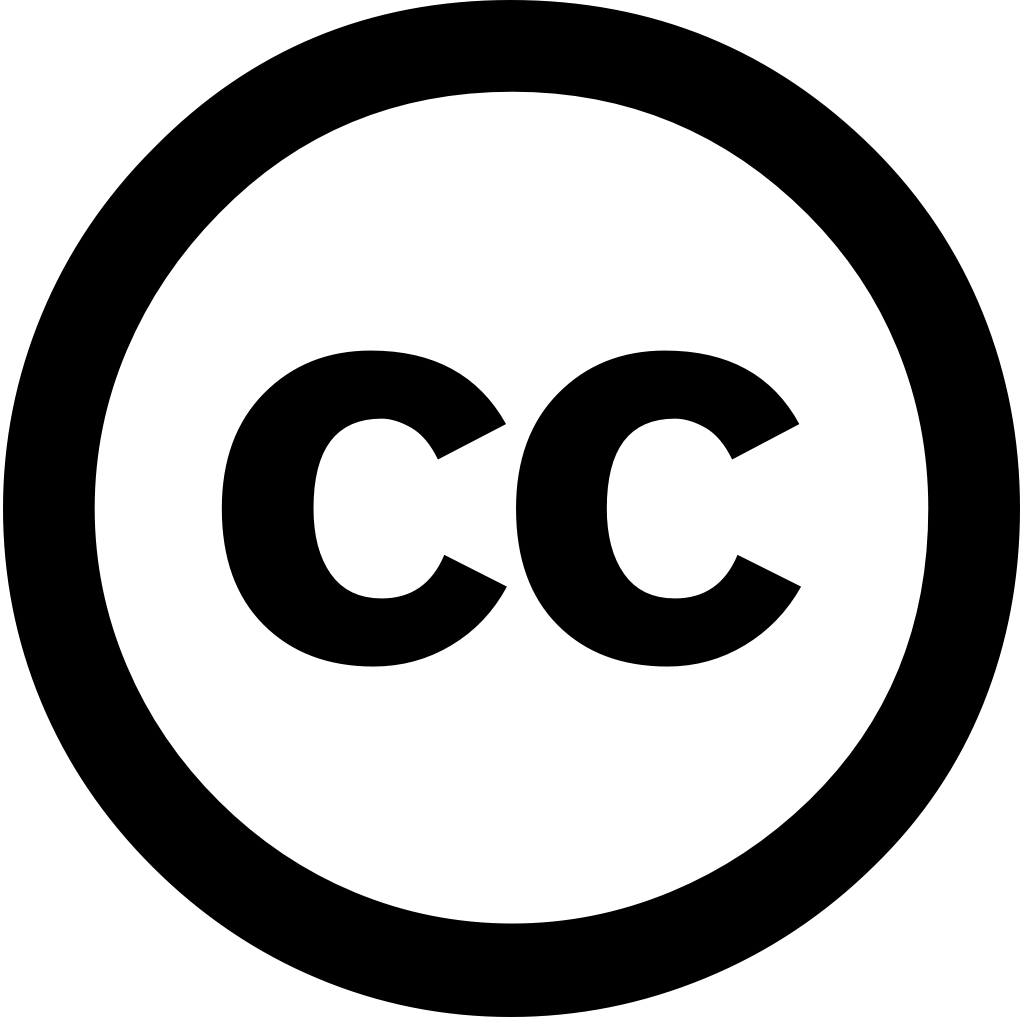
Research Square (Research Square), Journal Year: 2024, Volume and Issue: unknown
Published: Nov. 26, 2024
Language: Английский
Research Square (Research Square), Journal Year: 2024, Volume and Issue: unknown
Published: Nov. 26, 2024
Language: Английский
Strahlentherapie und Onkologie, Journal Year: 2024, Volume and Issue: unknown
Published: Nov. 6, 2024
Abstract Background Promptable foundation auto-segmentation models like Segment Anything (SA, Meta AI, New York, USA) represent a novel class of universal deep learning that could be employed for interactive tumor auto-contouring in RT treatment planning. Methods was evaluated an point-to-mask task glioma brain 16,744 transverse slices from 369 MRI datasets (BraTS 2020 dataset). Up to nine point prompts were automatically placed per slice. Tumor boundaries auto-segmented on contrast-enhanced T1w sequences. Out the three auto-contours predicted by SA, accuracy contour with highest calculated IoU (Intersection over Union, “oracle mask,” simulating model use selection best contour) and confidence (“suggested mask”). Results Mean (mbIoU) using (oracle mask) full 0.762 (IQR 0.713–0.917). The 2D mask achieved after mean 6.6 5–9). Segmentation significantly better high- compared low-grade cases (mbIoU 0.789 vs. 0.668). Accuracy worse suggested (0.572). Stacking segmentations slices, 3D Dice score 0.872, which improved 0.919 combining axial, sagittal, coronal contours. Conclusion segmentation can achieve high datasets. results suggest facilitate planning when properly integrated clinical application.
Language: Английский
Citations
8Frontiers in Artificial Intelligence, Journal Year: 2025, Volume and Issue: 7
Published: Jan. 14, 2025
Introduction Generating physician letters is a time-consuming task in daily clinical practice. Methods This study investigates local fine-tuning of large language models (LLMs), specifically LLaMA models, for letter generation privacy-preserving manner within the field radiation oncology. Results Our findings demonstrate that base without fine-tuning, are inadequate effectively generating letters. The QLoRA algorithm provides an efficient method intra-institutional LLMs with limited computational resources (i.e., single 48 GB GPU workstation hospital). fine-tuned LLM successfully learns oncology-specific information and generates institution-specific style. ROUGE scores generated summary reports highlight superiority 8B LLaMA-3 model over 13B LLaMA-2 model. Further multidimensional evaluations 10 cases reveal that, although has capacity to generate content beyond provided input data, it salutations, diagnoses treatment histories, recommendations further treatment, planned schedules. Overall, benefit was rated highly by experts (average score 3.4 on 4-point scale). Discussion With careful review correction, automated LLM-based significant practical value.
Language: Английский
Citations
0Deleted Journal, Journal Year: 2025, Volume and Issue: unknown
Published: Feb. 17, 2025
Citations
0Strahlentherapie und Onkologie, Journal Year: 2025, Volume and Issue: 201(3), P. 207 - 209
Published: Feb. 19, 2025
Language: Английский
Citations
0Journal of Applied Clinical Medical Physics, Journal Year: 2025, Volume and Issue: unknown
Published: March 2, 2025
Abstract Purpose Reducing radiation dose attributed to computed tomography (CT) may compromise the accuracy of organ segmentation, an important step in 177 Lu DOTATATE therapy that affects both activity and mass estimates. This study aimed facilitate CT reduction using deep learning methods for patients undergoing serial single photon emission (SPECT)/CT imaging during therapy. Methods The patient dataset hosted Deep Blue Data was used this study. noise insertion method incorporating effect bowtie filter, automatic exposure control, electronic applied simulate images at four reduced levels. Organ segmentation carried out TotalSegmentator model, while image denoising performed with DenseNet model. impact performance on dosimetry quantified by calculating percent difference between a rate map segmented reference mask same test (PD ) spleen, right kidney, left liver. Results Before denoising, mean ± standard deviation PD all critical organs were 2.31 2.94%, 4.86 9.42%, 8.39 14.76%, 12.95 19.99% levels down 20%, 10%, 5%, 2.5% normal dose, respectively. After corresponding results 1.69 2.25%, 2.84 4.46%, 3.72 4.22%, 7.98 15.05% Conclusion As increased, gradually deteriorated, which turn deteriorated Improving quality through could enhance dosimetry, making it valuable tool support SPECT/CT treatment.
Language: Английский
Citations
0Information, Journal Year: 2025, Volume and Issue: 16(3), P. 215 - 215
Published: March 11, 2025
As yet, there is no systematic review focusing on benefits and issues of commercial deep learning-based auto-segmentation (DLAS) software for prostate cancer (PCa) radiation therapy (RT) planning despite that NRG Oncology has underscored such necessity. This article’s purpose to systematically DLAS product performances PCa RT their associated evaluation methodology. A literature search was performed with the use electronic databases 7 November 2024. Thirty-two articles were included as per selection criteria. They evaluated 12 products (Carina Medical LLC INTContour (Lexington, KY, USA), Elekta AB ADMIRE (Stockholm, Sweden), Limbus AI Inc. Contour (Regina, SK, Canada), Manteia Technologies Co. AccuContour (Jian Sheng, China), MIM Software ProtégéAI (Cleveland, OH, Mirada Ltd. DLCExpert (Oxford, UK), MVision.ai Contour+ (Helsinki, Finland), Radformation AutoContour (New York, NY, RaySearch Laboratories RayStation Siemens Healthineers AG AI-Rad Companion Organs RT, syngo.via Image Suite DirectORGANS (Erlangen, Germany), Therapanacea Annotate (Paris, France), Varian Systems, Ethos (Palo Alto, CA, USA)). Their results illustrate can delineate organs at risk (abdominopelvic cavity, anal canal, bladder, body, cauda equina, left (L) right (R) femurs, L R pelvis, proximal sacrum) four clinical target volumes (prostate, lymph nodes, bed, seminal vesicle bed) clinically acceptable outcomes, resulting in delineation time reduction, 5.7–81.1%. Although recommended each centre perform its own prior implementation, seems more important due methodological respective single studies, e.g., small dataset used, etc.
Language: Английский
Citations
0JCO Oncology Practice, Journal Year: 2025, Volume and Issue: unknown
Published: March 13, 2025
Much work has been published on artificial intelligence (AI) and oncology, with many focusing an algorithm perspective. However, very few perspective articles have explicitly discussed the role of AI in oncology from perspectives stakeholders—the clinicians patients. In this article, we delve into opportunities clinician's patient's lens. From perspective, discuss reducing burnout, enhancing decision making, leveraging vast data sets to provide evidence-based recommendations, eventually affecting diagnostic accuracy treatment planning. virtual concierge, which could improve cancer care journey by facilitating patient education, mental health support, personalized lifestyle wellness recommendations promoting a holistic approach care. We aim highlight stakeholders' unmet needs guide institutions create innovative solutions oncology. By addressing these perspectives, our article aims bridge gap between technological research advancements their real-world AI-focused clinical applications Understanding prioritizing stakeholders will foster development impactful tools intentional utilization such technology, for implementation integration workflows.
Language: Английский
Citations
0Radiation Oncology, Journal Year: 2025, Volume and Issue: 20(1)
Published: March 14, 2025
Abstract Objective To investigate the feasibility of active heart sparing (AHS) planning in patients with locally advanced and centrally located NSCLC receiving standard definitive radiotherapy (RT), while maintaining or improving appropriate lung, esophagus, spinal cord constraints target volume (PTV) coverage intent. Methods materials A total 27 stage IIIA/B treated curative intent RT were selected for this analysis. All existing radiation plans revised further new equivalent calculated using AHS same cohort patients. Primary end-point was substructures. The secondary end point to calculate difference terms dosimetric parameters substructures principal OARs as well PTV-coverage within current patient group. Results feasible entire group An optimal obtained all mandatory have been met. median value mean dose (MHD) 8.18 Gy 6.71 AHS-group, respectively ( p = 0.000). Other such V 5Gy (40.57% vs. 27.7%; 0.000) 30Gy (5.39% 3.86%; significantly worse following relevant regarding found be compared AHS-group: base (16.97 6.37 Gy, 0.000), maximum (18.64 6.05 15Gy (11.11% 0% LAD; dose; (9.55% 0.94%, 23Gy (0.00% 0.00% 45.68% 6.57%, 0.002 left ventricle. Conclusion Our analysis showed an improvement affected by optimization. This approach could lead a possible reduction events prolonged survival. New clinical studies should include cardiologic evaluations biomarkers contouring cardiac
Language: Английский
Citations
0Strahlentherapie und Onkologie, Journal Year: 2025, Volume and Issue: unknown
Published: April 28, 2025
Language: Английский
Citations
0Journal of Neuro-Oncology, Journal Year: 2025, Volume and Issue: unknown
Published: May 1, 2025
Language: Английский
Citations
0