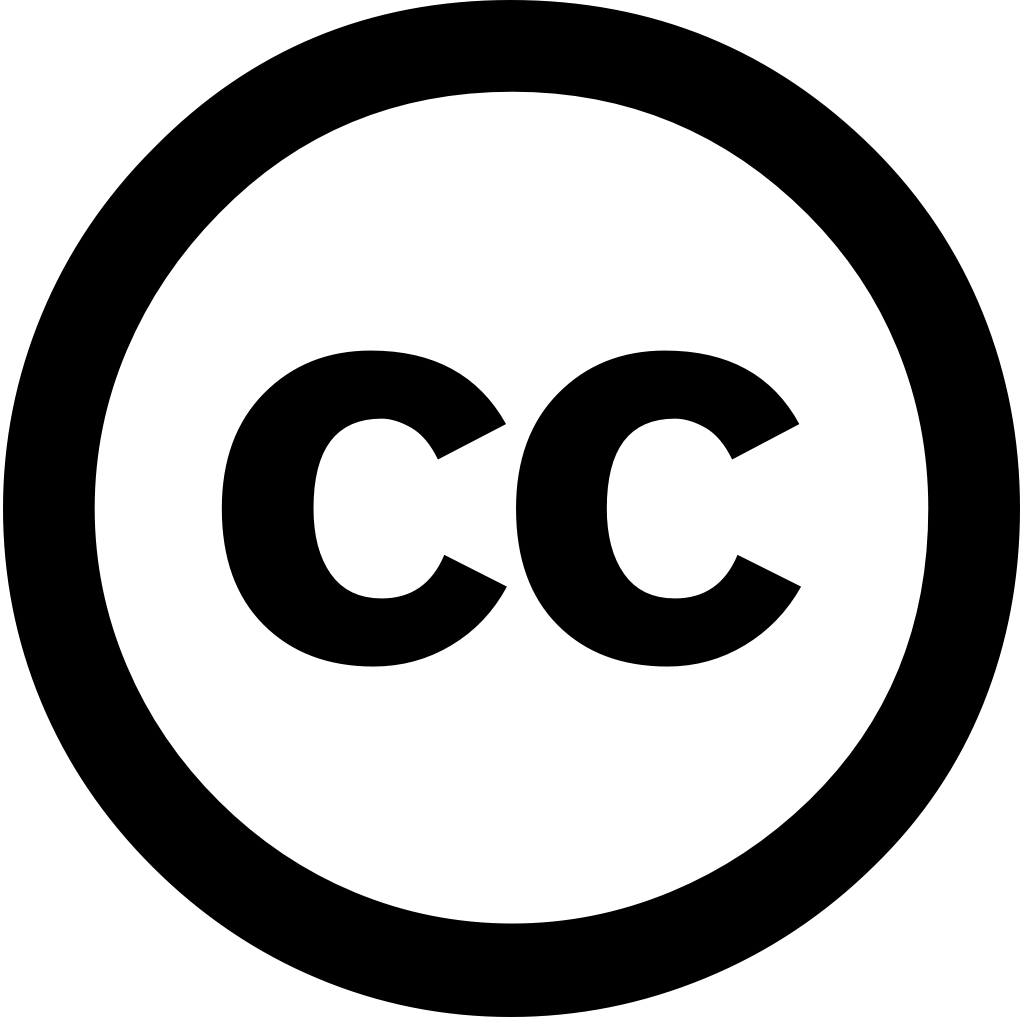
Teaching and Teacher Education, Journal Year: 2024, Volume and Issue: 148, P. 104717 - 104717
Published: July 24, 2024
Language: Английский
Teaching and Teacher Education, Journal Year: 2024, Volume and Issue: 148, P. 104717 - 104717
Published: July 24, 2024
Language: Английский
Advanced Materials, Journal Year: 2023, Volume and Issue: 35(45)
Published: June 19, 2023
Abstract Mechanical metamaterials are meticulously designed structures with exceptional mechanical properties determined by their microstructures and constituent materials. Tailoring material geometric distribution unlocks the potential to achieve unprecedented bulk functions. However, current metamaterial design considerably relies on experienced designers' inspiration through trial error, while investigating responses entails time‐consuming testing or computationally expensive simulations. Nevertheless, recent advancements in deep learning have revolutionized process of metamaterials, enabling property prediction geometry generation without prior knowledge. Furthermore, generative models can transform conventional forward into inverse design. Many studies implementation highly specialized, pros cons may not be immediately evident. This critical review provides a comprehensive overview capabilities prediction, generation, metamaterials. Additionally, this highlights leveraging create universally applicable datasets, intelligently intelligence. article is expected valuable only researchers working but also those field materials informatics.
Language: Английский
Citations
124Virtual and Physical Prototyping, Journal Year: 2023, Volume and Issue: 18(1)
Published: March 9, 2023
Metal additive manufacturing is gaining immense research attention. Some of these efforts are associated with physics, statistical, or artificial intelligence-driven process modelling and optimisation, structure–property characterisation, structural design equipment enhancements for cost reduction faster throughputs. In this review, the focus drawn on utilisation topology optimisation in metal manufacturing. First, symbiotic relationship between aerospace, medical, automotive, other industries investigated. Second, support structure by thermal-based powder-bed processes discussed. Third, introduction capabilities to limit constraints generate porous features examined. Fourth, emerging adopt intelligence models Finally, some open-source commercial software explored. This study considers challenges faced while providing perceptions future directions.
Language: Английский
Citations
86Advanced Materials, Journal Year: 2023, Volume and Issue: 36(8)
Published: Dec. 5, 2023
Abstract Metamaterials are artificial materials designed to exhibit effective material parameters that go beyond those found in nature. Composed of unit cells with rich designability assembled into multiscale systems, they hold great promise for realizing next‐generation devices exceptional, often exotic, functionalities. However, the vast design space and intricate structure–property relationships pose significant challenges their design. A compelling paradigm could bring full potential metamaterials fruition is emerging: data‐driven This review provides a holistic overview this rapidly evolving field, emphasizing general methodology instead specific domains deployment contexts. Existing research organized modules, encompassing data acquisition, machine learning‐based cell design, optimization. The approaches further categorized within each module based on shared principles, analyze compare strengths applicability, explore connections between different identify open questions opportunities.
Language: Английский
Citations
76Progress in Materials Science, Journal Year: 2023, Volume and Issue: 138, P. 101129 - 101129
Published: April 23, 2023
Additive manufacturing (AM) processes have proven to be a perfect match for topology optimization (TO), as they are able realize sophisticated geometries in unique layer-by-layer manner. From viewpoint, however, there is significant likelihood of process-related defects within complex geometrical features designed by TO. This because TO seldomly accounts process constraints and conditions typically perceived purely design tool. On the other hand, advanced AM simulations shown their potential reliable tools capable predicting various hence serving second-to-none material tool achieving targeted properties. Thus far, these two geometry been traditionally viewed entirely separate paradigms, whereas one must conceive them holistic computational instead. More specifically, models provide input physics-based TO, where consequently, not only component will function optimally, but also near-to-minimum defects. In this regard, we aim at giving thorough overview concepts applied AM. The paper arranged following way: first, literature on performance reviewed then most recent developments techniques related covered. Process play pivotal role latter type serve additional top primary end-user objectives. As natural consequence this, comprehensive detailed review non-metallic metallic additive performed, divided into micro-scale deposition-scale simulations. Material multi-scaling which central process-structure-property relationships, next followed subsection reduced-order versions incorporable due lower requirements. Finally concluded suggestions further research paths discussed.
Language: Английский
Citations
66Materials Science and Engineering R Reports, Journal Year: 2023, Volume and Issue: 156, P. 100755 - 100755
Published: Oct. 7, 2023
Language: Английский
Citations
56Chemosphere, Journal Year: 2024, Volume and Issue: 356, P. 141770 - 141770
Published: March 28, 2024
Language: Английский
Citations
41Computational Mechanics, Journal Year: 2024, Volume and Issue: 74(2), P. 281 - 331
Published: Jan. 13, 2024
Abstract The rapid growth of deep learning research, including within the field computational mechanics, has resulted in an extensive and diverse body literature. To help researchers identify key concepts promising methodologies this field, we provide overview deterministic mechanics. Five main categories are identified explored: simulation substitution, enhancement, discretizations as neural networks, generative approaches, reinforcement learning. This review focuses on methods rather than applications for thereby enabling to explore more effectively. As such, is not necessarily aimed at with knowledge learning—instead, primary audience verge entering or those attempting gain discussed are, therefore, explained simple possible.
Language: Английский
Citations
28Computer Methods in Applied Mechanics and Engineering, Journal Year: 2024, Volume and Issue: 421, P. 116766 - 116766
Published: Jan. 17, 2024
Language: Английский
Citations
24Structural and Multidisciplinary Optimization, Journal Year: 2022, Volume and Issue: 65(11)
Published: Oct. 25, 2022
Language: Английский
Citations
53Computer Methods in Applied Mechanics and Engineering, Journal Year: 2023, Volume and Issue: 413, P. 116126 - 116126
Published: June 2, 2023
Language: Английский
Citations
43