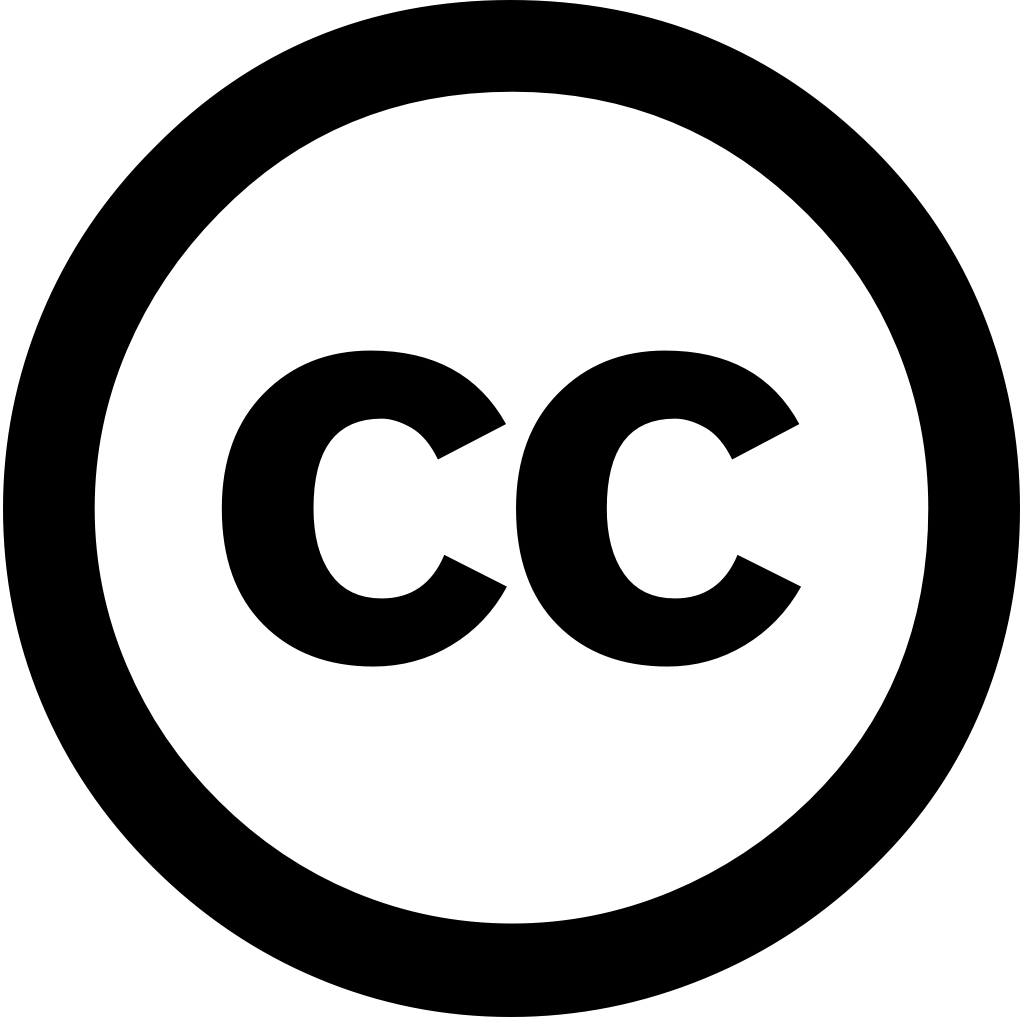
Mehran University Research Journal of Engineering and Technology, Journal Year: 2025, Volume and Issue: 44(2), P. 35 - 46
Published: April 9, 2025
Travel dynamics significantly impact commuter stress, influenced by traffic behavior, road conditions, travel modes, distance, and socio-demographic characteristics. Previous research on stress often exhibits limitations, including narrow scopes focusing specific routes, vehicle types, or demographics. This study addresses these constraints employing a comprehensive approach to analyze the influence of various attributes levels. An interview-based dataset was collected capture multifaceted experiences users. Five tree-based machine learning models–Decision Tree (DT), Random Forests (RF), Extra Trees (ET), Extreme Gradient Boosting (XGBoost), k-Nearest Neighbor (k-NN)–were deployed for imbalanced multi-class classification. XGBoost demonstrated superior performance with highest accuracy (73.33%) precision (75.63%) standard deviation ±5.9. A novel double hyperparameter optimization technique enhanced prediction across all models, notably increasing k-NN classifier’s 19.99%. The SHAP (SHapley Additive exPlanations) method utilized model interpretability, revealing distance traveled per day as most influential factor levels, followed mode transport, gender, age low, medium, high-stress categories, respectively. also examines features individual levels through random instance selection. provides valuable insights into complex interplay between paving way development effective mitigation strategies improved
Language: Английский