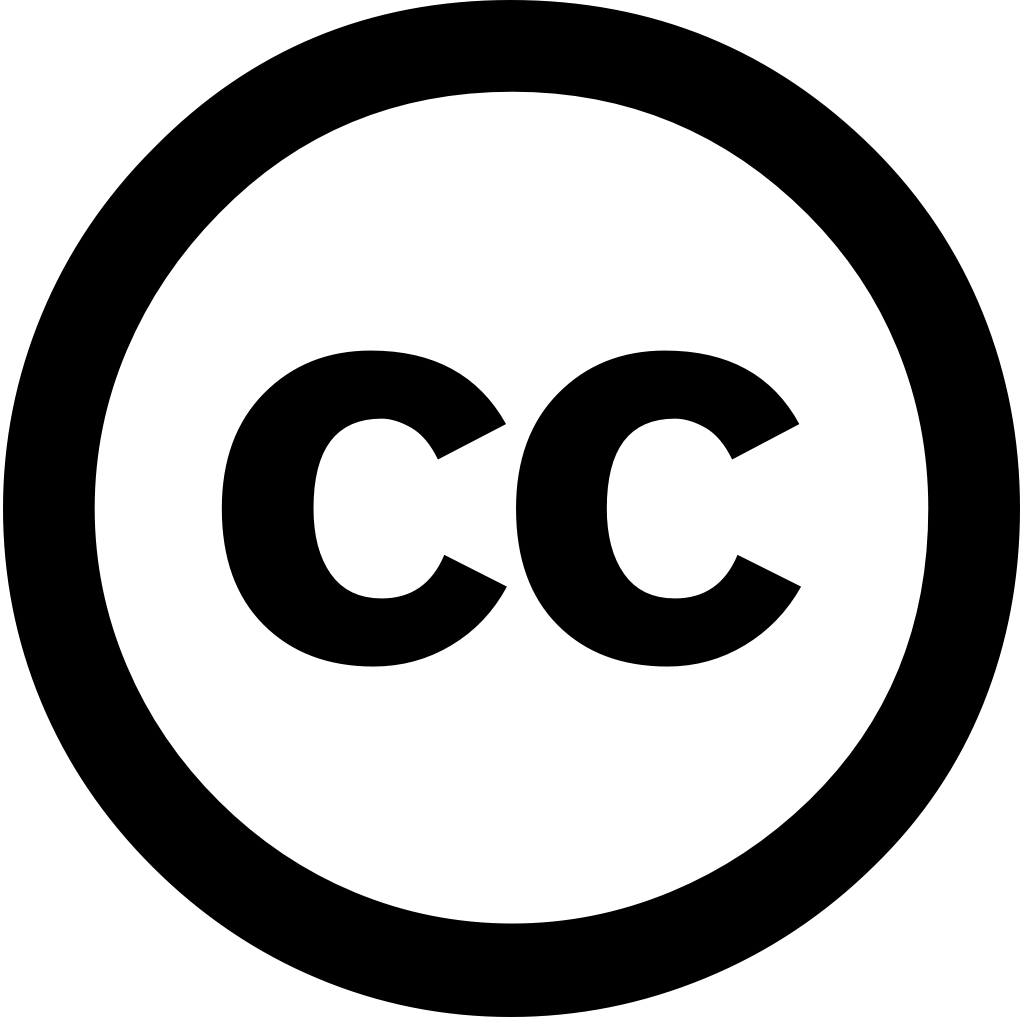
NeuroImage, Journal Year: 2024, Volume and Issue: unknown, P. 120911 - 120911
Published: Oct. 1, 2024
Language: Английский
NeuroImage, Journal Year: 2024, Volume and Issue: unknown, P. 120911 - 120911
Published: Oct. 1, 2024
Language: Английский
Journal of Affective Disorders, Journal Year: 2022, Volume and Issue: 323, P. 631 - 639
Published: Dec. 12, 2022
Language: Английский
Citations
26Neuropsychopharmacology, Journal Year: 2024, Volume and Issue: 50(1), P. 103 - 113
Published: Sept. 6, 2024
Abstract Computational models have become integral to human neuroimaging research, providing both mechanistic insights and predictive tools for cognition behavior. However, concerns persist regarding the ecological validity of lab-based studies whether their spatiotemporal resolution is not sufficient capturing neural dynamics. This review aims re-examine utility computational neuroimaging, particularly in light growing prominence alternative neuroscientific methods emphasis on more naturalistic behaviors paradigms. Specifically, we will explore how modeling can enhance analysis high-dimensional imaging datasets and, conversely, conjunction with other data modalities, inform through lens neurobiological plausibility. Collectively, this evidence suggests that remains critical neuroscience when enhanced by models, serve an important role bridging levels understanding. We conclude proposing key directions future emphasizing development standardized paradigms integrative use across techniques.
Language: Английский
Citations
4Cerebral Cortex, Journal Year: 2024, Volume and Issue: 34(5)
Published: May 1, 2024
Abstract Speech comprehension in noise depends on complex interactions between peripheral sensory and central cognitive systems. Despite having normal hearing, older adults show difficulties speech comprehension. It remains unclear whether the brain’s neural responses could indicate aging. The current study examined individual brain activation during perception different listening environments predict age. We applied functional near-infrared spectroscopy to 93 normal-hearing human (20 70 years old) a sentence task, which contained quiet condition 4 signal-to-noise ratios (SNR = 10, 5, 0, −5 dB) noisy conditions. A data-driven approach, region-based brain-age predictive modeling was adopted. observed significant behavioral decrease with age under conditions, but not condition. Brain activations SNR 10 dB successfully individual’s Moreover, we found that bilateral visual cortex, left dorsal pathway, cerebellum, right temporal–parietal junction area, homolog Wernicke’s middle temporal gyrus contributed most prediction performance. These results demonstrate of regions about sensory-motor mapping sound, especially be sensitive measures for than external behavior measures.
Language: Английский
Citations
2PLoS ONE, Journal Year: 2024, Volume and Issue: 19(5), P. e0300720 - e0300720
Published: May 30, 2024
Alterations in the brain’s connectivity or interactions among brain regions have been studied with aid of resting state (rs)fMRI data attained from large numbers healthy subjects various demographics. This has instrumental providing insight into how a phenotype as fundamental age affects brain. Although machine learning (ML) techniques already deployed such studies, novel questions are investigated this work. We study whether young brains develop properties that progressively resemble those aged brains, and if aging dynamics older provide information about trajectory subjects. The degree prospective monotonic relationship will be quantified, hypotheses trajectories tested via ML. Furthermore, functional across spectrum three datasets compared at population level sexes. findings scrutinize similarities differences male female greater detail than previously performed.
Language: Английский
Citations
1Medical Physics, Journal Year: 2023, Volume and Issue: 50(6), P. 3873 - 3884
Published: April 5, 2023
The lack of analysis brain networks in individuals with end-stage renal disease (ESRD) is an obstacle to detecting and preventing neurological complications ESRD.This study aims explore the correlation between activity ESRD based on a quantitative dynamic functional connectivity (dFC) networks. It provides insights into differences healthy patients identify activities regions most relevant ESRD.Differences were analyzed quantitatively evaluated this study. Blood oxygen level-dependent (BOLD) signals obtained through resting-state magnetic resonance imaging (rs-fMRI) used as information carriers. First, matrix dFC was constructed for each subject using Pearson correlation. Then high-order built by applying "correlation's correlation" method. Second, sparsification performed graphical least absolute shrinkage selection operator (gLASSO) model. discriminative features sparse extracted sifted central moments t-tests, respectively. Finally, feature classification conducted support vector machine (SVM).The experiment showed that reduced some degree certain patients. sensorimotor, visual, cerebellum subnetworks had highest numbers abnormal connectivities. inferred these three likely have direct relationship ESRD.The low-order can positions where damage occurs In contrast individuals, damaged disruption not limited specific regions. This indicates has severe impact function. Abnormal mainly associated responsible visual processing, emotional, motor control. findings presented here potential use detection, prevention, prognostic evaluation ESRD.
Language: Английский
Citations
2Brain Science Advances, Journal Year: 2023, Volume and Issue: 9(2), P. 78 - 94
Published: June 1, 2023
Schizophrenia (SZ) and bipolar disorder (BD) are two of the most frequent mental disorders. These disorders exhibit similar psychotic symptoms, making diagnosis challenging leading to misdiagnosis. Yet, network complexity changes driving spontaneous brain activity in SZ BD patients still unknown. Functional entropy (FE) is a novel way measuring dispersion (or spread) functional connectivities inside brain. The FE was utilized this study examine resting-state fMRI data at three levels, including global, modules, nodes. At considerably lower than that normal control (NC). intra-module level, substantially higher cingulo-opercular network. Moreover, strong negative association between clinical measures discovered patient groups. Finally, we classified using features attained an accuracy 66.7% (BD vs. NC) 75.0% (SZ BD). findings proposed connectivity’s analyses can provide important insights for illness.
Language: Английский
Citations
2bioRxiv (Cold Spring Harbor Laboratory), Journal Year: 2024, Volume and Issue: unknown
Published: March 31, 2024
Abstract Recent efforts in cognitive neuroscience have focused on leveraging resting-state functional connectivity (RSFC) data from fMRI to predict human traits and behaviors more accurately. Traditional methods typically analyze RSFC by correlating averaged time-series between regions of interest (ROIs) or networks, a process that may overlook critical spatial signal patterns. To address this limitation, we introduced novel linear regression technique estimates predicting brain activity patterns target ROI those seed ROI. We applied both traditional our estimation large-scale dataset the Human Connectome Project, analyzing sex, age, personality traits, psychological task performance. Additionally, developed an ensemble learner integrates these using weighted average approach enhance prediction accuracy. Our findings revealed hierarchical clustering method displays distinct whole-brain grouping compared approach. Importantly, model outperformed behaviors. Notably, predictions showed relatively low similarity, indicating captures unique previously undetected information about through fine-grained local neural activation. These results highlight potential combining innovative analysis techniques enrich understanding basis
Language: Английский
Citations
0Quantitative Imaging in Medicine and Surgery, Journal Year: 2024, Volume and Issue: 14(5), P. 3350 - 3365
Published: April 11, 2024
Yulong Wang, Yande Ren, Yuzhen Bi, Feng Zhao, Xingzhen Bai, Liangzhou Wei, Wanting Liu, Hancheng Ma, Peirui Bai
Language: Английский
Citations
0NeuroImage, Journal Year: 2024, Volume and Issue: unknown, P. 120911 - 120911
Published: Oct. 1, 2024
Language: Английский
Citations
0