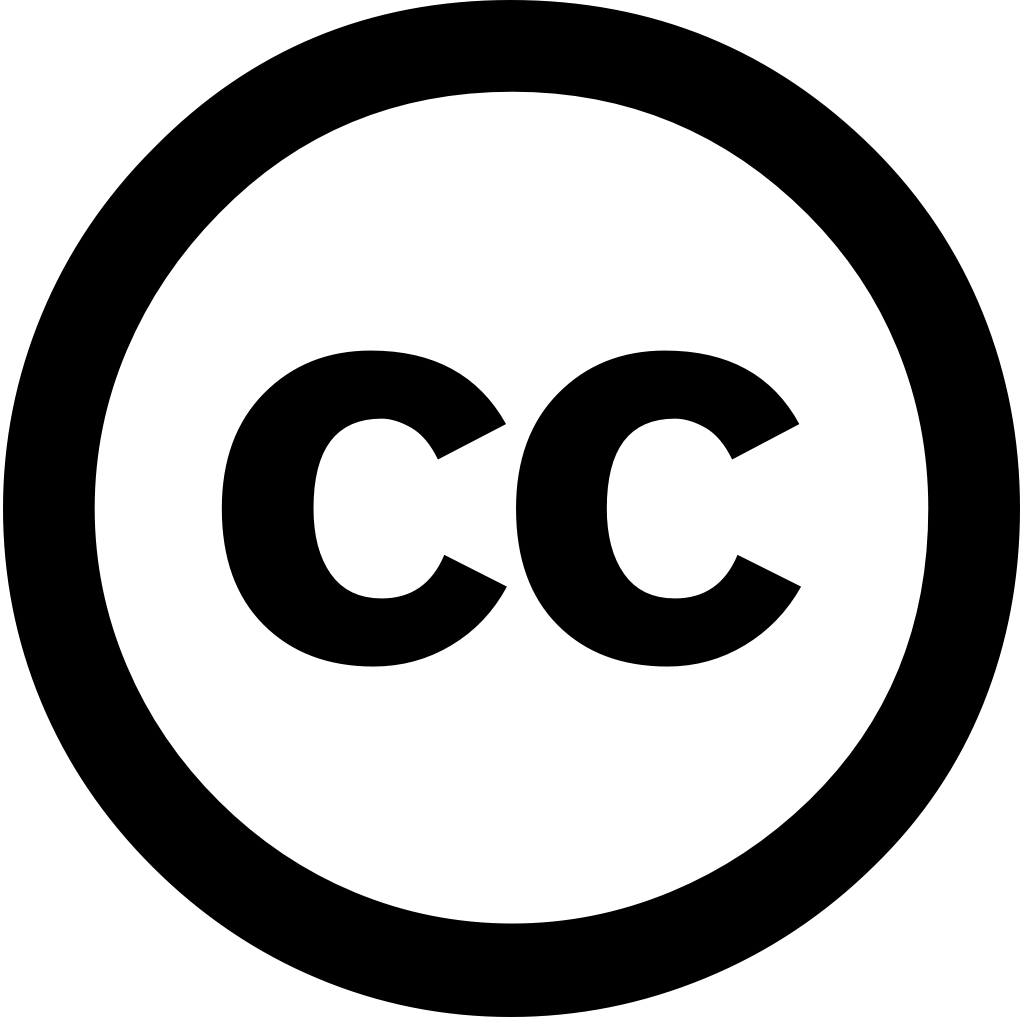
JMIR AI, Journal Year: 2024, Volume and Issue: unknown
Published: Sept. 23, 2024
Language: Английский
JMIR AI, Journal Year: 2024, Volume and Issue: unknown
Published: Sept. 23, 2024
Language: Английский
Frontiers in Medicine, Journal Year: 2025, Volume and Issue: 12
Published: Jan. 23, 2025
Large Language Models (LLMs) are transforming patient education in medication management by providing accessible information to support healthcare decision-making. Building on our recent scoping review of LLMs education, this perspective examines their specific role guidance. These artificial intelligence (AI)-driven tools can generate comprehensive responses about drug interactions, side effects, and emergency care protocols, potentially enhancing autonomy decisions. However, significant challenges exist, including the risk misinformation complexity accurate without access individual data. Safety concerns particularly acute when patients rely solely AI-generated advice for self-medication This analyzes current capabilities, critical limitations, raises questions regarding possible integration We emphasize need regulatory oversight ensure these serve as supplements to, rather than replacements for, professional
Language: Английский
Citations
1medRxiv (Cold Spring Harbor Laboratory), Journal Year: 2024, Volume and Issue: unknown
Published: Aug. 12, 2024
Background: Generative Large language models (LLMs) represent a significant advancement in natural processing, achieving state-of-the-art performance across various tasks. However, their application clinical settings using real electronic health records (EHRs) is still rare and presents numerous challenges. Objective: This study aims to systematically review the use of generative LLMs, effectiveness relevant techniques patient care-related topics involving EHRs, summarize challenges faced, suggest future directions. Methods: A Boolean search for peer-reviewed articles was conducted on May 19th, 2024 PubMed Web Science include research published since 2023, which one month after release ChatGPT. The results were deduplicated. Multiple reviewers, including biomedical informaticians, computer scientists, physician, screened publications eligibility data extraction. Only studies utilizing LLMs analyze EHR included. We summarized prompt engineering, fine-tuning, multimodal data, evaluation matrices. Additionally, we identified current applying as reported by included proposed Results: initial 6,328 unique studies, with 76 screening. Of these, 67 (88.2%) employed zero-shot prompting, five them 100% accuracy specific Nine used advanced prompting strategies; four tested these strategies experimentally, finding that engineering improved performance, noting non-linear relationship between number examples improvement. Eight explored fine-tuning all improvements tasks, but three noted potential degradation certain two utilized LLM-based decision-making enabled accurate disease diagnosis prognosis. 55 different metrics 22 purposes, such correctness, completeness, conciseness. Two investigated LLM bias, detecting no bias other male patients received more appropriate suggestions. Six hallucinations, fabricating names structured thyroid ultrasound reports. Additional not limited impersonal tone consultations, made uncomfortable, difficulty had understanding responses. Conclusion: Our indicates few have computational enhance performance. diverse highlight need standardization. currently cannot replace physicians due
Language: Английский
Citations
4Information, Journal Year: 2025, Volume and Issue: 16(4), P. 284 - 284
Published: April 1, 2025
Electronic Medical Records (EMRs) are central to the modern healthcare system. Recent advances in artificial intelligence (AI), particularly generative (GenAI), have opened new opportunities for advancement of EMRs. This scoping review aims explore current real-world applications GenAI within EMRs support an understanding AI healthcare. A literature search was conducted following PRISMA-ScR guidelines. The using Ovid MEDLINE, up 28 October 2024, a peer-reviewed strategy. Overall, 55 studies were included. list five themes generated by human reviewers based on review: data manipulation (24), patient communication (9), clinical decision making (8), prediction summarization (4), and other (2). majority originated from United States (35). Both proprietary commercially available models tested, with ChatGPT being most commonly referenced LLM. As these continue be developed, their diverse use cases potential improve outcomes, enhance access medical data, streamline hospital workflows, reduce physician workload. However, continued problems surrounding privacy, trust, bias, model hallucinations, need robust evaluation remain. Further research considering ethical, medical, societal implications is essential validate findings address existing limitations advancement.
Language: Английский
Citations
0European Journal of Clinical Pharmacology, Journal Year: 2024, Volume and Issue: unknown
Published: Sept. 10, 2024
Language: Английский
Citations
0Published: Sept. 23, 2024
Language: Английский
Citations
0Scientia Pharmaceutica, Journal Year: 2024, Volume and Issue: 92(4), P. 58 - 58
Published: Oct. 21, 2024
ChatGPT has emerged as a promising tool for enhancing clinical practice. However, its implementation raises critical questions about impact on this field. In scoping review, we explored the utility of in pharmacy A search was conducted five databases up to 23 May 2024. Studies analyzing use with direct or potential applications practice were included. total 839 records identified, which 14 studies included: six tested version 3.5, three 4.0, both versions, one used 3.0, and did not specify version. Only half evaluated real-world scenarios. reasonable number papers analyzed practice, highlighting benefits limitations. The indicated that is fully prepared due significant there great application context near future, following further improvements tool. Further exploration required, along proposing conscious appropriate utilization.
Language: Английский
Citations
0JMIR AI, Journal Year: 2024, Volume and Issue: unknown
Published: Sept. 23, 2024
Language: Английский
Citations
0