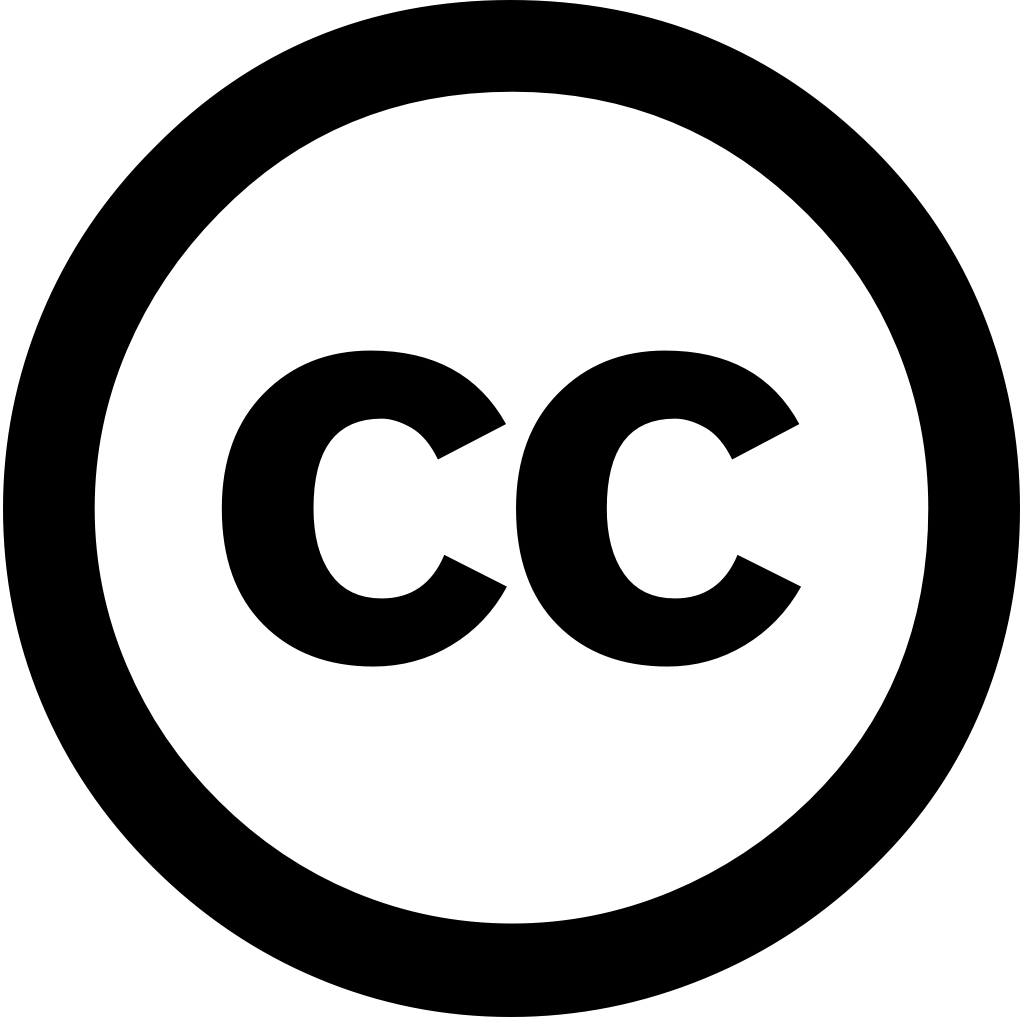
Frontiers in Oncology, Journal Year: 2024, Volume and Issue: 14
Published: Dec. 3, 2024
We used habitat radiomics as an innovative tumor biomarker to predict the outcome of neoadjuvant therapy for esophageal cancer.
Language: Английский
Frontiers in Oncology, Journal Year: 2024, Volume and Issue: 14
Published: Dec. 3, 2024
We used habitat radiomics as an innovative tumor biomarker to predict the outcome of neoadjuvant therapy for esophageal cancer.
Language: Английский
BMC Cancer, Journal Year: 2025, Volume and Issue: 25(1)
Published: Jan. 9, 2025
This study aims to quantify intratumoral heterogeneity (ITH) using preoperative CT image and evaluate its ability predict pathological high-grade patterns, specifically micropapillary and/or solid components (MP/S), in patients diagnosed with clinical stage I lung adenocarcinoma (LADC). In this retrospective study, we enrolled 457 who were postoperatively LADC from two medical centers, assigning them either a training set (n = 304) or test 153). Sub-regions within the tumor identified K-means method. Both ecological diversity features (hereafter referred as ITH) conventional radiomics C-radiomics) extracted generate ITH scores C-radiomics scores. Next, univariate multivariate logistic regression analyses employed identify clinical-radiological (Clin-Rad) associated MP/S (+) group for constructing Clin-Rad classification. Subsequently, hybrid model which presented nomogram was developed, integrating classification score. The performance of models assessed receiver operating characteristic (ROC) curves, area under curve (AUC), accuracy, sensitivity, specificity determined. score outperformed both classification, evidenced by higher AUC values (0.820 versus 0.810 0.700, p 0.049 0.031, respectively) (0.805 0.771 0.732, 0.041 0.025, respectively). Finally, consistently demonstrated robust predictive capabilities identifying presence components, achieving 0.830 0.849 (all < 0.05). derived sub-region has been shown be reliable predictor LADC.
Language: Английский
Citations
1Clinical Breast Cancer, Journal Year: 2025, Volume and Issue: unknown
Published: Feb. 1, 2025
Language: Английский
Citations
0Published: Jan. 1, 2025
Language: Английский
Citations
0Cancer Management and Research, Journal Year: 2025, Volume and Issue: Volume 17, P. 731 - 741
Published: April 1, 2025
Abstract: Radiomics received a lot of attention because its potential to provide personalized medicine in non-invasive manner, usually focusing on the analysis entire lesion. A new method called habitat can identify subregional phenotypic changes within lesion, thereby improving ability distinguish heterogeneity. The clustering be applied multiple measurement parameters separate different tumor habitats by segmentation. data-driven repeatable voxel subregions reflecting live tumors will valuable for clinical diagnosis and further treatment. In this review, we aim briefly summarize widely used cluster algorithms subregion segmentation application imaging. By analyzing many literatures, commonly K-means algorithm other such as hierarchical consensus are summarized. identifying intratumoral heterogeneity, key findings oncology described, differentiation, grading, gene expression status. latest progress innovations predicting therapeutic effects prognosis using reviewed, including multimodal imaging data fusion, integration with artificial intelligence technologies, diagnostic methods. limitations challenges also discussed, dependence image quality techniques, insufficient automation standardization, difficulties biological interpretation, lack validation. Finally, future directions increasing level standardization improve accuracy efficiency reduce reliance expert intervention proposed. Habitat represents significant advancement radiomics, offering nuanced understanding leveraging sophisticated integrating data, has transform decision-making, enabling more precise diagnostics treatment strategies, ultimately advancing field precision medicine. Keywords: habitat, analysis, imaging, K-means, radiomics
Language: Английский
Citations
0Diagnostics, Journal Year: 2025, Volume and Issue: 15(8), P. 953 - 953
Published: April 9, 2025
Background: Breast cancer is the second leading cause of cancer-related mortality among women, accounting for 12% cases. Early diagnosis, based on identification radiological features, such as masses and microcalcifications in mammograms, crucial reducing rates. However, manual interpretation by radiologists complex subject to variability, emphasizing need automated diagnostic tools enhance accuracy efficiency. This study compares a radiomics workflow machine learning (ML) with deep (DL) approach classifying breast lesions benign or malignant. Methods: matRadiomics was used extract features from mammographic images 1219 patients CBIS-DDSM public database, including 581 cases 638 masses. Among ML models, linear discriminant analysis (LDA) demonstrated best performance both lesion types. External validation conducted private dataset 222 evaluate generalizability an independent cohort. Additionally, EfficientNetB6 model employed comparison. Results: The LDA achieved mean AUC 68.28% 61.53% In external validation, values 66.9% 61.5% were obtained, respectively. contrast, superior performance, achieving 81.52% 76.24% masses, highlighting potential DL improved accuracy. Conclusions: underscores limitations ML-based diagnosis. Deep proves be more effective approach, offering enhanced supporting clinicians improving patient management.
Language: Английский
Citations
0Molecular Carcinogenesis, Journal Year: 2025, Volume and Issue: unknown
Published: Jan. 21, 2025
The standard therapy for locally unresectable advanced non-small cell lung cancer (NSCLC) is comprised of chemoradiotherapy (CRT) before immunotherapy (IO) consolidation. However, how to predict treatment outcomes and recognize patients that will benefit from IO remain unclear. This study aimed identify prognostic biomarkers by integrating computed tomography (CT)-based radiomics genomics. Specifically, our research involved 165 suffering Stage III NSCLC. Cohort 1 (IO following CRT) was divided into D1 (n = 74), D2 32), D3 26) sets, the remaining 33 treated with CRT alone were grouped in D4. According CT images primary tumor regions, radiomic features analyzed through least absolute shrinkage selection operator (LASSO) regression. Rad-score figured out forecast progression-free survival (PFS). Rad-score, high low risk groups. Next-generation sequencing implemented on peripheral blood tissue samples D4 cohorts. maximum somatic allele frequency (MSAF) about circulating DNA levels assessed. Mismatch repair switching/sucrose non-fermenting signaling pathways significantly enriched low-risk group compared high-risk (p < 0.05). Moreover, MSAF ≥ 1% those showing a decrease after benefited IO. developed model predicting PFS NSCLC constructed radio-genomic map underlying biomarkers, supplying valuable insights biology.
Language: Английский
Citations
0Frontiers in Immunology, Journal Year: 2025, Volume and Issue: 16
Published: March 12, 2025
Background Construction and validation of an automated breast volume ultrasound (ABVS)-based nomogram for assessing axillary lymph node (ALNs) metastasis in (AUS)-negative early cancer. Methods A retrospective study 174 patients with AUS-negative early-stage cancer was divided into a training test ratio 7:3. Radiomics features were extracted by combining images intra-tumor peri-tumor ABVS. Select the best classifier from 3 machine learning techniques to build Model 1and radiomics-score (RS). Differences ER, PR, Her-2, Ki-67 expression analyzed intra-tumoral peri-tumoral habitat radiomics features. 2 (based on sonogram features) RS constructed multivariate logistic regression. Efficiency models evaluated area under curve (AUC). Plotting evaluating its treatment ALN≥3 according 3. Result Intratumoral peritumoral 5 mm screened using least absolute shrinkage selection operator (LASSO), regression used as best-performing 1. Using unsupervised cluster analysis, intratumoral 5mm classified habitats, they differed PR Her-2 expression. (combining diameter microcalcification) created achieves highest AUC both (0.827) (0.768) sets. The Nomo-score calculated based nomogram-model2 nomogram-model3, revealing positive correlation between ALN burden Nomo-score. Combined optimal thresholds, 54.6%-100% ≥3 nomogram-model3 81.8%-100% ≥3. Conclusion ABVS-based is effective tool metastasis, it can provide preoperative basis individualized
Language: Английский
Citations
0Published: Jan. 1, 2025
Language: Английский
Citations
0MedComm, Journal Year: 2025, Volume and Issue: 6(5)
Published: May 1, 2025
ABSTRACT Organoid technology, as an emerging field within biotechnology, has demonstrated transformative potential in advancing precision medicine. This review systematically outlines the translational trajectory of organoids from bench to bedside, emphasizing their construction methodologies, key regulatory factors, and multifaceted applications personalized healthcare. By recapitulating physiological architectures disease phenotypes through three‐dimensional culture systems, leverage natural synthetic scaffolds, stem cell sources, spatiotemporal cytokine regulation model tissue‐specific microenvironments. Diverse organoid types—including skin, intestinal, lung, tumor organoids—have facilitated breakthroughs modeling tissue development, drug efficacy toxicity screening, pathogenesis studies, patient‐tailored diagnostics. For instance, patient‐derived preserve heterogeneity genomic profiles, serving predictive platforms for individualized chemotherapy responses. In medicine, organoid‐guided multiomics analyses identify actionable biomarkers resistance mechanisms, while clustered regularly interspaced short palindromic repeats‐based functional screens optimize therapeutic targeting. Despite preclinical successes, challenges persist standardization, vascularization, ethical considerations. Future integration artificial intelligence, microfluidics, spatial transcriptomics will enhance scalability, reproducibility, clinical relevance. bridging molecular insights with patient‐specific therapies, are poised revolutionize offering dynamic regenerative strategies, treatment paradigms.
Language: Английский
Citations
0Cancer Imaging, Journal Year: 2025, Volume and Issue: 25(1)
Published: May 3, 2025
Abstract Objectives The World Health Organization/International Society of Urological Pathology (WHO/ISUP) grading clear cell renal carcinoma (ccRCC) is crucial for prognosis and treatment planning. This study aims to predict the grade using intratumoral peritumoral subregional CT radiomics analysis better clinical interventions. Methods Data from two hospitals included 513 ccRCC patients, who were divided into training (70%), validation (30%), an external set (testing) 67 patients. Using ITK-SNAP, radiologists annotated tumor regions interest (ROI) extended surrounding areas by 1 mm, 3 5 mm. K-means clustering algorithm region three sub-regions, Least Absolute Shrinkage Selection Operator (LASSO) regression identified most predictive features. Various machine learning models established, including models, based on heterogeneity (ITH) score, comprehensive models. Predictive ability was evaluated receiver operating characteristic (ROC) curves, area under curve (AUC) values, DeLong tests, calibration decision curves. Results combined model showed strong power with AUC 0.852 (95% CI: 0.725–0.979) test data, outperforming individual ITH score highly precise, AUCs 0.891 0.854–0.927) in training, 0.877 0.814–0.941) validation, 0.847 0.725–0.969) testing, proving its superior across datasets. Conclusion A combining Habitat, Peri1mm, salient features significantly more accurate predicting pathologic grading. Key points Question : Characterize non-invasively WHO/ISUP pathological preoperatively. Findings An integrated subregion characterization, characteristics, can Clinical relevance Subregion characterization outperforms single-entity approach. model, compared boosts prognostic accuracy targeted actions.
Language: Английский
Citations
0