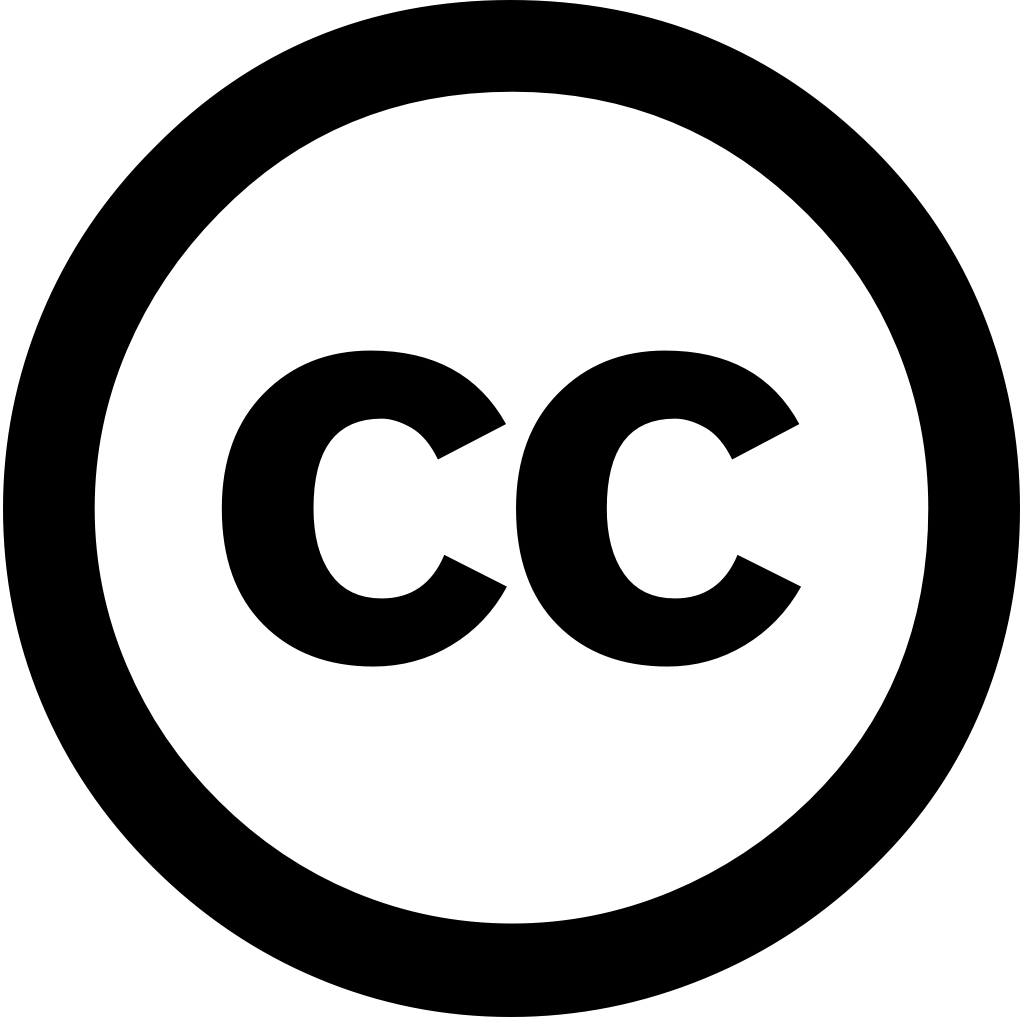
Research Square (Research Square), Journal Year: 2024, Volume and Issue: unknown
Published: Nov. 18, 2024
Language: Английский
Research Square (Research Square), Journal Year: 2024, Volume and Issue: unknown
Published: Nov. 18, 2024
Language: Английский
Academic Radiology, Journal Year: 2025, Volume and Issue: unknown
Published: Feb. 1, 2025
Language: Английский
Citations
0BMC Medical Imaging, Journal Year: 2025, Volume and Issue: 25(1)
Published: Feb. 26, 2025
To develop a predictive nomogram for breast cancer lympho-vascular invasion (LVI), based on digital tomography (DBT) data obtained from intra- and peri-tumoral regions. One hundred ninety-two patients were enrolled in this retrospective study 2 institutions, which Institution 1 served as the basis training (n = 113) testing 49) sets, while external validation set 30). Tumor regions of interest (ROI) manually-delineated DBT images, ROI was defined mm around intra-tumoral ROI. Radiomics features extracted, logistic regression used to construct intra-, peri-, + radiomics models. Patient clinical analyzed by both uni- multi-variable analyses identify independent risk factors non-radiomics imaging model, combination most optimal models comprised comprehensive model. The best-performing model out 3 types (radiomics, imaging, comprehensive) identified using receiver operating characteristic (ROC) curve analysis, nomogram. LVI, maximum tumor diameter (odds ratio [OR] 1.486, 95% confidence interval [CI] 1.082–2.041, P 0.014), suspicious malignant calcification (OR 2.898, CI 1.232 ~ 6.815, 0.015), axillary lymph node (ALN) metastasis 3.615, 1.642–7.962, < 0.001) Furthermore, accurate predicting LVI occurrence, with areas under (AUCs) 0.889, 0.916, 0.862, for, respectively, training, compared (0.858, 0.849, 0.844) (0.743, 0.759, 0.732). resulting nomogram, incorporating well diameter, calcification, ALN metastasis, had great correspondence actual diagnoses calibration curve, high utility decision analysis. derived features, highly identifying future occurrence cancer, demonstrating its potential an assistive tool clinicians devise individualized treatment regimes.
Language: Английский
Citations
0Academic Radiology, Journal Year: 2025, Volume and Issue: unknown
Published: March 1, 2025
Language: Английский
Citations
0European journal of medical research, Journal Year: 2025, Volume and Issue: 30(1)
Published: March 17, 2025
Surgery offers the potential for a radical cure and prolonged survival in individuals diagnosed with esophageal squamous cell carcinoma (ESCC). However, rates exhibit significant variability among patients. Accurately assessing surgical outcomes remains critical challenge. This study aimed to evaluate predictive value of preoperative radiomics prognostic nutritional index ESCC develop comprehensive model estimating postoperative overall (OS) these A retrospective analysis was conducted on 466 patients from two medical centers. The dataset randomly divided into training cohort (TC, hospital 1, 246 cases), an internal validation (IVC, 106 external (EVC, 2, 114 cases). Radiological features were extracted after delineating region interest, followed by application least absolute shrinkage selection operator (LASSO) regression identify optimal compute score (RS). Independent factors identified via Cox incorporated RS construct combined nomogram. performance evaluated using concordance index, time-dependent receiver operating characteristic (ROC) curves, calibration plots, decision curve analysis. model, which integrated radiomics, tumor-node-metastasis (TNM) staging estimate 3 year OS rate, achieved area under ROC (AUC) values 0.812, 0.786, 0.810 TC, IVC, EVC, respectively, demonstrating excellent accuracy. These surpassed AUCs TNM 0.717, 0.612, 0.699, respectively. model's indexes EVC 0.780, 0.760, 0.764, Calibration curves highlighted nomogram's superior clinical utility. developed combining enabling estimation ESCC. holds promise as tool risk stratification.
Language: Английский
Citations
0Frontiers in Oncology, Journal Year: 2025, Volume and Issue: 15
Published: May 12, 2025
Purpose To develop a radiomics model based on ultrasound images for predicting risk of recurrence in breast cancer patients. Methods In this retrospective study, 420 patients with pathologically confirmed were included, randomly divided into training (70%) and test (30%) sets, an independent external validation cohort 90 According to St. Gallen criteria, categorized two groups, low-medium-risk high-risk. Radiomics features extracted from analysis set using Pyradiomics. The informative screened the minimum redundancy maximum relevance (mRMR) least absolute shrinkage selection operator (LASSO) algorithms. Subsequently, models constructed eight machine learning Three distinct nomogram created selected through multivariate logistic regression, including Clinic-Ultrasound (Clin-US), Clinic-Radiomics (Clin-Rad), Clinic-Ultrasound-Radiomics (Clin-US-Rad) models. receiver operating characteristic (ROC), calibration, decision curve (DCA) curves used evaluate model’s clinical applicability predictive performance. Results A total 12 screened, which wavelet.LHL first order Mean weighed more tended have high recurrence. higher recurrence, score (Rad-score) all three sets (training, test, set, p < 0.05). Rad-score is equally applicable four different subtypes cancer. Clin-US-Rad achieved highest AUC values (AUC = 0.817 0.851, respectively). calibration DCA also demonstrated good utility combined model. Conclusion learning-based useful nomograms show promising potential assessing This non-invasive approach offers crucial guidance diagnosis treatment condition.
Language: Английский
Citations
0Insights into Imaging, Journal Year: 2025, Volume and Issue: 16(1)
Published: May 18, 2025
Lymphovascular invasion significantly impacts the prognosis of urothelial carcinoma bladder. Traditional lymphovascular detection methods are time-consuming and costly. This study aims to develop a deep learning-based model preoperatively predict status in bladder using CT images. Data images 577 patients across four medical centers were retrospectively collected. The largest tumor slices from transverse, coronal, sagittal planes selected used train CNN models (InceptionV3, DenseNet121, ResNet18, ResNet34, ResNet50, VGG11). Deep learning features extracted visualized Grad-CAM. Principal Component Analysis reduced 64. Using features, Decision Tree, XGBoost, LightGBM trained with 5-fold cross-validation ensembled stacking model. Clinical risk factors identified through logistic regression analyses combined DL scores enhance prediction accuracy. ResNet50-based achieved an AUC 0.818 validation set 0.708 testing set. showed 0.794 0.767 set, demonstrating robust performance diverse data. We developed radiomics based on offers non-invasive, cost-effective tool assist clinicians personalized treatment planning. feature-based CT. Max cross sections three dimensions image made comparisons six networks, including ResNet50.
Language: Английский
Citations
0Clinical Breast Cancer, Journal Year: 2024, Volume and Issue: 24(6), P. e485 - e494.e1
Published: Aug. 1, 2024
Language: Английский
Citations
2Academic Radiology, Journal Year: 2024, Volume and Issue: 31(10), P. 3917 - 3928
Published: April 23, 2024
Language: Английский
Citations
2Journal of Radiation Research and Applied Sciences, Journal Year: 2024, Volume and Issue: 17(4), P. 101174 - 101174
Published: Nov. 3, 2024
Language: Английский
Citations
2Diagnostics, Journal Year: 2023, Volume and Issue: 13(20), P. 3280 - 3280
Published: Oct. 23, 2023
Background: Carpal tunnel syndrome (CTS) is the most common entrapment neuropathy for which ultrasound imaging has recently emerged as a valuable diagnostic tool. This meta-analysis aims to investigate role of radiomics in diagnosis CTS and compare it with other approaches. Methods: We conducted comprehensive search electronic databases from inception September 2023. The included studies were assessed quality using Quality Assessment Tool Diagnostic Accuracy Studies. primary outcome was performance compared radiologist evaluation diagnosing CTS. Results: Our five observational comprising 840 participants. In context evaluation, combined statistics sensitivity, specificity, odds ratio 0.78 (95% confidence interval (CI), 0.71 0.83), 0.72 CI, 0.59 0.81), 9 5 15), respectively. contrast, training mode yielded sensitivity 0.88 0.85 0.91), specificity 0.84 0.92), 58 38 87). Similarly, testing demonstrated an aggregated 0.89), 0.80 0.73 0.85), 22 12 41). Conclusions: contrast assessments by radiologists, exhibited superior detecting Furthermore, there minimal variability accuracy between sets radiomics, highlighting its potential robust tool
Language: Английский
Citations
5