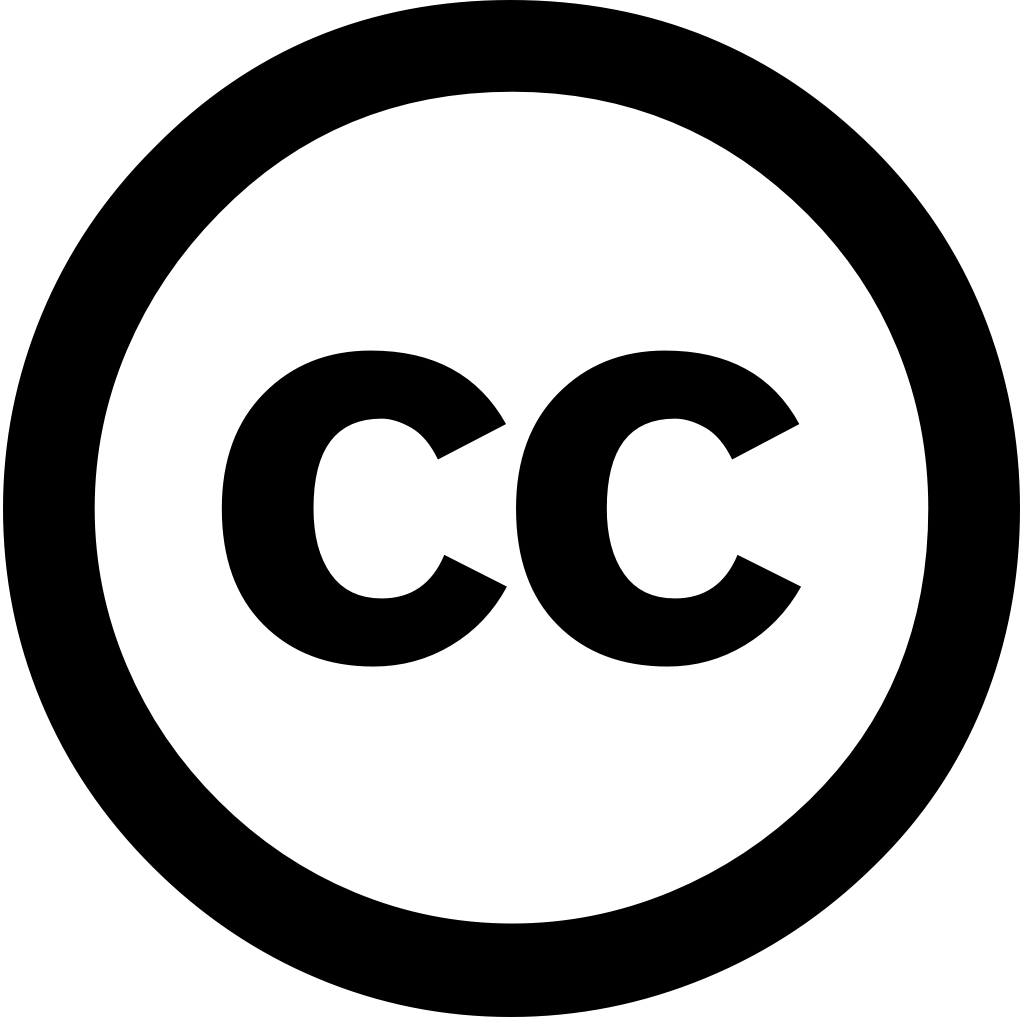
Journal of Medical Internet Research, Journal Year: 2024, Volume and Issue: unknown
Published: Nov. 14, 2024
Language: Английский
Journal of Medical Internet Research, Journal Year: 2024, Volume and Issue: unknown
Published: Nov. 14, 2024
Language: Английский
Cancers, Journal Year: 2025, Volume and Issue: 17(4), P. 621 - 621
Published: Feb. 12, 2025
Background/Objectives: Detecting lung nodules on computed tomography (CT) images is critical for diagnosing thoracic cancers. Deep learning models, particularly convolutional neural networks (CNNs), show promise in automating this process. This systematic review and meta-analysis aim to evaluate the diagnostic accuracy of these focusing lesion-wise sensitivity as primary metric. Methods: A comprehensive literature search was conducted, identifying 48 studies published up 7 November 2023. The pooled performance assessed using a random-effects model, with key outcome. Factors influencing model performance, including participant demographics, dataset privacy, data splitting methods, were analyzed. Methodological rigor maintained through Checklist Artificial Intelligence Medical Imaging (CLAIM) Quality Assessment Diagnostic Accuracy Studies-2 (QUADAS-2) tools. Trial Registration: registered PROSPERO under CRD42023479887. Results: revealed 79% (95% CI: 72-86%) independent datasets 85% 83-88%) across all datasets. Variability associated characteristics study methodologies. Conclusions: While deep models demonstrate significant potential nodule detection, findings highlight need more diverse datasets, standardized evaluation protocols, interventional enhance generalizability clinical applicability. Further research necessary validate broader patient populations.
Language: Английский
Citations
0Lecture notes in computer science, Journal Year: 2025, Volume and Issue: unknown, P. 209 - 220
Published: Jan. 1, 2025
Language: Английский
Citations
0The Ewha Medical Journal, Journal Year: 2024, Volume and Issue: 47(4)
Published: Sept. 12, 2024
Artificial intelligence (AI) is rapidly transforming various medical fields, including radiation oncology. This review explores the integration of AI into oncology, highlighting both challenges and opportunities. can improve precision, efficiency, outcomes therapy by optimizing treatment planning, enhancing image analysis, facilitating adaptive therapy, enabling predictive analytics. Through analysis large datasets to identify optimal parameters, automate complex tasks, reduce planning time, accuracy. In AI-driven techniques enhance tumor detection segmentation processing data from CT, MRI, PET scans enable precise delineation. beneficial because it allows real-time adjustments plans based on changes in patient anatomy size, thereby improving accuracy effectiveness. Predictive analytics using historical predict potential complications, guiding clinical decision-making more personalized strategies. Challenges adoption oncology include ensuring quality quantity, achieving interoperability standardization, addressing regulatory ethical considerations, overcoming resistance implementation. Collaboration among researchers, clinicians, scientists, industry stakeholders crucial these obstacles. By challenges, drive advancements care operational efficiencies. presents an overview current state insights future directions for research practice.
Language: Английский
Citations
2Published: Nov. 14, 2024
Language: Английский
Citations
0Cancers, Journal Year: 2024, Volume and Issue: 17(1), P. 69 - 69
Published: Dec. 29, 2024
Background: Positron emission tomography (PET) is a valuable tool for the assessment of lymphoma, while artificial intelligence (AI) holds promise as reliable resource analysis medical images. In this context, we systematically reviewed applications deep learning (DL) interpretation lymphoma PET Methods: We searched PubMed until 11 September 2024 studies developing DL models evaluation images patients with lymphoma. The risk bias and applicability concerns were assessed using prediction model (PROBAST). articles included categorized presented based on task performed by proposed models. Our study was registered international prospective register systematic reviews, PROSPERO, CRD42024600026. Results: From 71 papers initially retrieved, 21 total 9402 participants ultimately in our review. achieved promising performance diverse tasks, namely, detection histological classification lesions, differential diagnosis from other conditions, quantification metabolic tumor volume, treatment response survival areas under curve, F1-scores, R2 values up to 0.963, 87.49%, 0.94, respectively. Discussion: primary limitations several small number absence external validation. conclusion, can reliably be aided models, which are not designed replace physicians but assist them managing large volumes scans through rapid accurate calculations, alleviate their workload, provide decision support tools precise care improved outcomes.
Language: Английский
Citations
0Journal of Medical Internet Research, Journal Year: 2024, Volume and Issue: unknown
Published: Nov. 14, 2024
Language: Английский
Citations
0