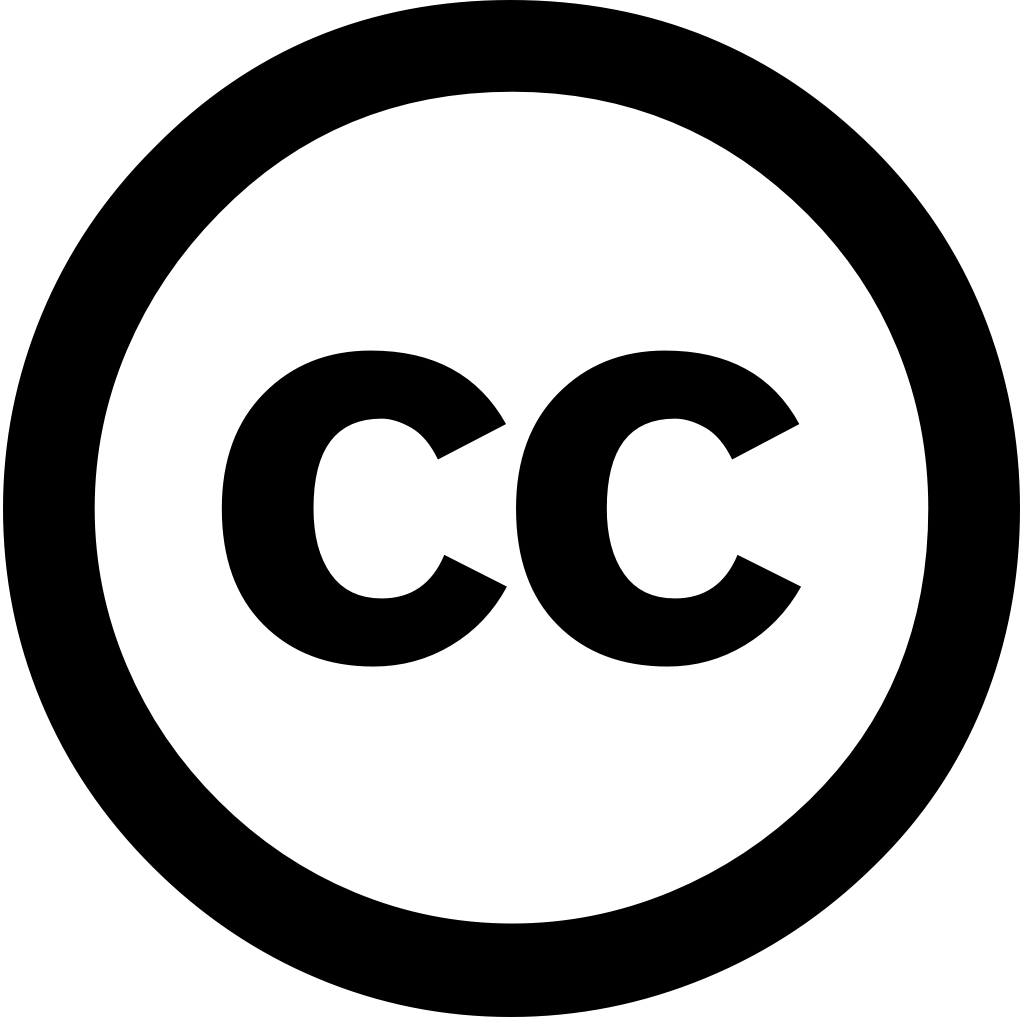
Radiation Oncology, Journal Year: 2025, Volume and Issue: 20(1)
Published: June 5, 2025
Language: Английский
Radiation Oncology, Journal Year: 2025, Volume and Issue: 20(1)
Published: June 5, 2025
Language: Английский
Artificial Intelligence Review, Journal Year: 2024, Volume and Issue: 57(2)
Published: Jan. 29, 2024
Abstract In biomedical image analysis, the applicability of deep learning methods is directly impacted by quantity data available. This due to models requiring large datasets provide high-level performance. Generative Adversarial Networks (GANs) have been widely utilized address limitations through generation synthetic images. GANs consist two models. The generator, a model that learns how produce images based on feedback it receives. discriminator, classifies an as or real and provides generator. Throughout training process, GAN can experience several technical challenges impede suitable imagery. First, mode collapse problem whereby generator either produces identical uniform from distinct input features. Second, non-convergence gradient descent optimizer fails reach Nash equilibrium. Thirdly, vanishing unstable behavior occurs discriminator achieving optimal classification performance resulting in no meaningful being provided These problems result production imagery blurry, unrealistic, less diverse. To date, there has survey article outlining impact these context domain. work presents review taxonomy solutions imaging highlights important outlines future research directions about domain
Language: Английский
Citations
29Electronics, Journal Year: 2022, Volume and Issue: 11(21), P. 3457 - 3457
Published: Oct. 25, 2022
Brain tumor (BTs) is considered one of the deadly, destructive, and belligerent disease, that shortens average life span patients. Patients with misdiagnosed insufficient medical treatment BTs have less chance survival. For analysis, magnetic resonance imaging (MRI) often utilized. However, due to vast data produced by MRI, manual segmentation in a reasonable period time difficult, which limits application standard criteria clinical practice. So, efficient automated techniques are required. The accurate early detection difficult challenging task biomedical imaging. Automated an issue because considerable temporal anatomical variability brain tumors. Early therefore essential. To detect cancers or tumors, different classical machine learning (ML) algorithms been main difficulty these models manually extracted features. This research provides deep hybrid (DeepTumorNetwork) model binary classification overcomes above-mentioned problems. proposed method GoogLeNet architecture CNN eliminating 5 layers adding 14 extracts features automatically. On same Kaggle (Br35H) dataset, key performance indicator was compared transfer (TL) (ResNet, VGG-16, SqeezNet, AlexNet, MobileNet V2) ML/DL. Furthermore, approach outperformed based on (Acc, Recall, Precision, F1-Score) classification. Additionally, methods exhibited high measures, Accuracy (99.51%), Precision (99%), Recall (98.90%), F1-Score (98.50%). approaches show its superiority recent sibling for current using MRI images.
Language: Английский
Citations
51IEEE Access, Journal Year: 2023, Volume and Issue: 11, P. 60279 - 60291
Published: Jan. 1, 2023
Lung cancer is the most common cause of deaths worldwide. Early detection crucial for successful treatment and increasing patient survival rates. Artificial intelligence techniques can play a significant role in early lung cancer. Various methods based on machine learning deep approaches are used to detect This research works aims develop automated accurately identify classify CT scans by using computational techniques. The process typically involves lobe segmentation, extracting candidate nodules, classifying nodules as either or non-cancer. proposed classification uses modified U-Net segmentation nodule model consisting three phases. first phase segments slice predicted mask architecture second extracts label employing architecture. Finally, third AlexNet, support vector applied into experimental results methodology extraction, have shown promising publicly available LUAN16 dataset. AlexNet-SVM achieves 97.98% accuracy, 98.84% sensitivity, 97.47% specificity, 97.53% precision, 97.70% F1
Language: Английский
Citations
35Sensors, Journal Year: 2023, Volume and Issue: 23(20), P. 8589 - 8589
Published: Oct. 20, 2023
Medical image segmentation is crucial for medical processing and the development of computer-aided diagnostics. In recent years, deep Convolutional Neural Networks (CNNs) have been widely adopted achieved significant success. UNet, which based on CNNs, mainstream method used segmentation. However, its performance suffers owing to inability capture long-range dependencies. Transformers were initially designed Natural Language Processing (NLP), sequence-to-sequence applications demonstrated ability their abilities acquire local information are limited. Hybrid architectures CNNs Transformer, such as TransUNet, proposed benefit from Transformer's dependencies CNNs' low-level details. Nevertheless, automatic remains a challenging task due factors blurred boundaries, low-contrast tissue environment, in context ultrasound, issues like speckle noise attenuation. this paper, we propose new model that combines strengths both with network architectural improvements enrich feature representation captured by skip connections decoder. To end, devised attention module called Three-Level Attention (TLA). This composed an Gate (AG), channel attention, spatial normalization mechanism. The AG preserves structural information, whereas helps interdependencies between channels. Spatial employs coefficient Transformer improve akin TransNorm. further connection reduce semantic gap, encoder decoder redesigned manner similar UNet++ dense connection. Moreover, supervision using side-output was introduced, analogous BASNet, originally saliency predictions. Two datasets different modalities, CT scan dataset ultrasound dataset, evaluate UNet architecture. experimental results showed our consistently improved prediction across datasets.
Language: Английский
Citations
31Computer Methods and Programs in Biomedicine, Journal Year: 2023, Volume and Issue: 243, P. 107885 - 107885
Published: Oct. 27, 2023
Language: Английский
Citations
31IEEE Transactions on Circuits & Systems II Express Briefs, Journal Year: 2022, Volume and Issue: 69(11), P. 4593 - 4597
Published: June 8, 2022
Deep learning techniques, particularly convolutional neural networks, have shown great potential in computer vision and medical imaging applications. However, deep models are computationally demanding as they require enormous computational power specialized processing hardware for model training. To make these portable compatible prototyping, their implementation on low-power devices is imperative. In this work, we present the of Modified U-Net Intel Movidius Neural Compute Stick 2 (NCS-2) segmentation images. We selected because, image segmentation, a prominent that provides improved performance even if dataset size small. The modified evaluated terms dice score. Experiments reported task three datasets: BraTs brain MRI, heart MRI dataset, Ziehl-Neelsen sputum smear microscopy (ZNSDB) dataset. For proposed model, reduced number parameters from 30 million to 0.49 architecture. Experimental results show comparable while requiring significantly lower resources inference NCS-2. maximum scores recorded 0.96 0.94 0.74 ZNSDB
Language: Английский
Citations
32Neural Computing and Applications, Journal Year: 2024, Volume and Issue: 36(11), P. 5799 - 5825
Published: Jan. 13, 2024
Abstract Cell nucleus segmentation is an important method that widely used in the diagnosis and treatment of many diseases, as well counting identifying cell nucleus. The main challenges when using this are heterogeneous image intensities image, overlapping nuclei, noise. In order to overcome these difficulties, a hybrid model with attention block, CellSegUNet, proposed, inspired by advantageous points UNet++ Residual UNet models. With proposed mechanism, semantic gaps may occur prevented evaluating both horizontal vertical features together. serial parallel connection convolutional blocks residual modules CellSegUNet prevents data loss. Thus, stronger representation ability were obtained. output layer, which is, especially for model, calculated differences between each layer input layer. value obtained from level where lowest comes constitutes whole system. At same depth level, versus ResUNet models compared on Data Science Bowl (DSB), Sartorius Instance Segmentation (SCIS), Blood (BCS) datasets. accuracy, dice, jaccard metrics 0.980, 0.970, 0.959 DSB dataset, 0.931, 0.957, 0.829 SCIS dataset 0.976, 0.971, 0.927 BCS respectively. As result, it predicted can provide solutions different problems.
Language: Английский
Citations
9IEEE Transactions on Evolutionary Computation, Journal Year: 2024, Volume and Issue: 28(3), P. 597 - 607
Published: Jan. 11, 2024
Convolutional neural networks (CNNs) have shown a competitive performance in medical imaging applications, such as image segmentation. However, choosing an existing architecture capable of adapting to specific dataset is challenging and requires design expertise. Neural search (NAS) employed overcome these limitations. NAS uses techniques the Networks architecture. Typically, models' weights optimization carried out using continuous loss function, unlike model topology optimization, which highly influenced by problem. Genetic programming (GP) evolutionary algorithm (EA) problem CNNs considering attributes its representation. A tree representation can express complex connectivity apply variation operations. This article presents tree-based GP for evolving based on well-known U-Net producing compact flexible models segmentation across multiple domains. proposal called / (NASGP-Net). NASGP-Net cell-based encoding backbone construct hierarchical arrangement primitive Our experiments indicate that our approach produce remarkable results with fewer parameters regarding fixed architectures. Moreover, against methods. Finally, we observed notable improvements several evaluation metrics, including dice similarity coefficient (DSC), intersection over union (IoU), Hausdorff distance (HD).
Language: Английский
Citations
8Frontiers in Neuroscience, Journal Year: 2024, Volume and Issue: 18
Published: April 12, 2024
Introduction In neurological diagnostics, accurate detection and segmentation of brain lesions is crucial. Identifying these challenging due to its complex morphology, especially when using traditional methods. Conventional methods are either computationally demanding with a marginal impact/enhancement or sacrifice fine details for computational efficiency. Therefore, balancing performance precision in compute-intensive medical imaging remains hot research topic. Methods We introduce novel encoder-decoder network architecture named the Adaptive Feature Medical Segmentation Network (AFMS-Net) two encoder variants: Single Encoder Block (SAEB) Dual (DAEB). A squeeze-and-excite mechanism employed SAEB identify significant data while disregarding peripheral details. This approach best suited scenarios requiring quick efficient segmentation, an emphasis on identifying key lesion areas. contrast, DAEB utilizes advanced channel spatial attention strategy fine-grained delineation multiple-class classifications. Additionally, both architectures incorporate Path (SegPath) module between decoder, refining enhancing feature extraction, improving model stability. Results AFMS-Net demonstrates exceptional across several notable datasets, including BRATs 2021, ATLAS ISLES 2022. Its design aims construct lightweight capable handling challenges high precision. Discussion The proposed addresses critical balance issue efficiency lesions. By introducing tailored variants, adapts varying requirements speed feature. not only advances state-of-the-art but also provides scalable framework future image processing.
Language: Английский
Citations
8Computers in Biology and Medicine, Journal Year: 2024, Volume and Issue: 170, P. 108009 - 108009
Published: Jan. 15, 2024
Language: Английский
Citations
7