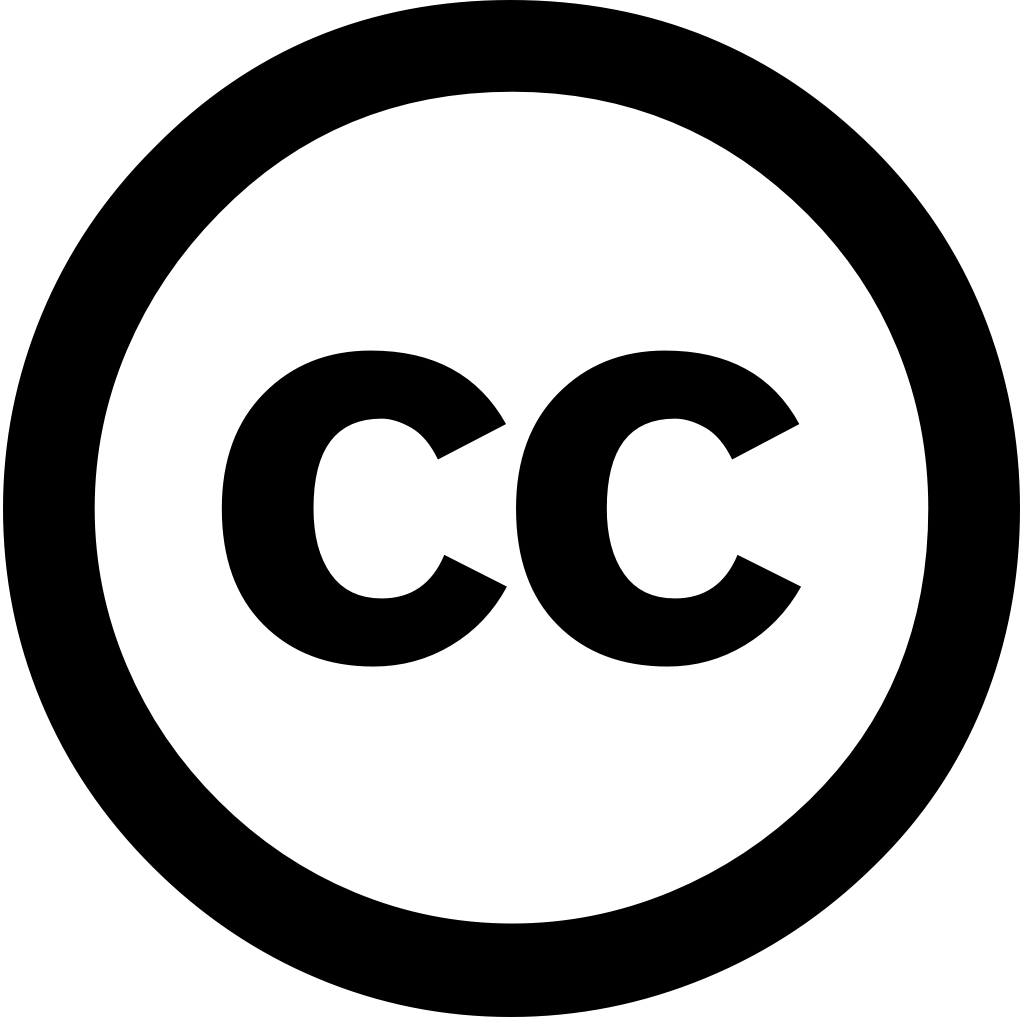
Alexandria Engineering Journal, Journal Year: 2024, Volume and Issue: 114, P. 1 - 11
Published: Nov. 26, 2024
Language: Английский
Alexandria Engineering Journal, Journal Year: 2024, Volume and Issue: 114, P. 1 - 11
Published: Nov. 26, 2024
Language: Английский
Signal Image and Video Processing, Journal Year: 2025, Volume and Issue: 19(3)
Published: Jan. 28, 2025
Language: Английский
Citations
1Engineering Applications of Artificial Intelligence, Journal Year: 2025, Volume and Issue: 147, P. 110343 - 110343
Published: Feb. 26, 2025
Language: Английский
Citations
1Engineering Research Express, Journal Year: 2025, Volume and Issue: 7(1), P. 015218 - 015218
Published: Jan. 7, 2025
Abstract Imaging in marine environment is a challenging task due to several reasons, including light variations, color distortions, noise, and limited datasets, which causes inaccurate target classification problems. This paper presents methodology for image biology environmental research. We proposed the Attention-Driven DenseNet-LSTM Network (ADL-Net), aiming improve accuracy of underwater imagery. Initially, feature extraction performed using multi-levels DenseNet201, excels hierarchical extraction, offering stability rapid convergence. In next stage, two modified attention mechanisms are designed: squeeze excitation operations used refine channel-specific responses, while convolutional block module refines both channels spatial dimensions. These help model highlight important features minimize distractions. Additionally, Bi-directional Long Short-Term Memory layer integrated capture dependencies robustness. Various training strategies find best parameter tuning. Our method demonstrates excellent performance when tested on fish datasets: LifeCLEF 2015 Fish4Knowledge, achieving accuracies 98.02%, 99.52%, respectively. findings underscore ADL-Net’s potential enhancing automated classification, advancing research ecology.
Language: Английский
Citations
0Computers in Biology and Medicine, Journal Year: 2024, Volume and Issue: 179, P. 108910 - 108910
Published: July 19, 2024
Language: Английский
Citations
2Food Chemistry, Journal Year: 2024, Volume and Issue: 460, P. 140795 - 140795
Published: Aug. 8, 2024
Language: Английский
Citations
2Multimedia Tools and Applications, Journal Year: 2024, Volume and Issue: unknown
Published: Oct. 22, 2024
Language: Английский
Citations
1Neural Computing and Applications, Journal Year: 2024, Volume and Issue: unknown
Published: Dec. 26, 2024
Language: Английский
Citations
1Tuijin Jishu/Journal of Propulsion Technology, Journal Year: 2023, Volume and Issue: 44(3), P. 3296 - 3321
Published: Sept. 11, 2023
Brain tumor is generally structured by the subtle summation of anomalous cells because it categorized as a mass tissue, and very crucial to categorize brain tumors from Magnetic Resonance Imaging (MRI) for diagnosis purpose. The investigation humans routine process detecting classifying MRI tumors. Due low contrast images, poor boundaries noises, image classification techniques provide performance in medical images. Hence, new model needed solve critical challenges existing approaches. This decreases human intervention while making decisions about Therefore, investigated approaches with deep learning. proposed framework consists two main different phases (i) normal abnormal images (b) Segmentation severity first step comprised pre-processing classification. Firstly, required are gathered benchmark databases. These collected undergone pre-processing, which done filtering methods Contrast Limited Adaptive Histogram Equalization (CLAHE). Subsequently, pre-processed fed into Transformer-based Multi-scale Residual Attention Network (TMRAN), where obtained. While second case, classified considered input segmentation process. segmented obtained Convolutional Neural (CNN), Modified UNet UNet, turn parameters optimized using Controlling Parameters-based African Vultures Optimization Algorithm (MCP-AVOA), termed UNet. At final stage, these applied novel TMRAN model, hyper tuned optimally improved MCP-AVOA order acquire optimal results. validated diverse measures compared over other conventional methodologies. Thus, findings elucidate that attains higher
Language: Английский
Citations
1International Journal For Multidisciplinary Research, Journal Year: 2024, Volume and Issue: 6(3)
Published: June 17, 2024
Sign language is used as a way of communication by deaf and mute individuals. However, due to the limited number people who understand sign language, integrating them into society challenging. Approximately 6.9% Bangladesh's population 5% world’s suffer from speech impediments. Individuals with this condition cannot hear what others are saying or communicate verbally, thus must be relied upon. In recent years, recognition has gained attention its necessity. scarcity publicly available dynamic gesture datasets for Bangladeshi Language (BdSL) exists. Dynamic gestures, which contain both spatial temporal information, more useful in real-life applications. The classification gestures requires data than static gestures. research, 11 numeral Bengali digit signs aimed classified. Data been collected “SignBD-Word” dataset, contains extracted RGB human body pose keypoints skeleton data. When compared other requirements training deep neural networks per class dataset found insufficient. A hybrid model architecture proposed research recognize using lightweight 3DCNN bidirectional LSTM layers classifying motion patterns after experimenting on various models. It observed that combining pre-trained DenseNet-201 BiLSTM increases real-time accuracy 4.54%. To best our knowledge, first approach combine action recognition. Also, one earliest investigations BdSL hand Additionally, different models base feature extractors have evaluated.
Language: Английский
Citations
0Mugla Journal of Science and Technology, Journal Year: 2024, Volume and Issue: unknown
Published: Nov. 17, 2024
Sign language is a vital communication tool for hearing-impaired individuals to express their thoughts and emotions. Turkish Language (TSL) based on hand gestures, facial expressions, body movements. In this study, deep learning models were developed recognize 41 commonly used TSL expressions. An original dataset was created using the Media Pipe Holistic framework capture 3D landmarks of hand, face, The study trained evaluated GRU, LSTM, Bi-LSTM models, as well hybrid architectures such CNN+GRU, GRU+LSTM, GRU+Bi-LSTM. training hold-out validation method used. 80% allocated 20% testing. Additionally, data validation. Among Deep Learning CNN+GRU model achieved highest accuracy rate 96.72%, outperforming similar studies in literature. Our results demonstrate that techniques can effectively classify with combination showing particularly high performance. Future work will focus expanding developing real-time recognition systems incorporate both skeleton images landmarks.
Language: Английский
Citations
0