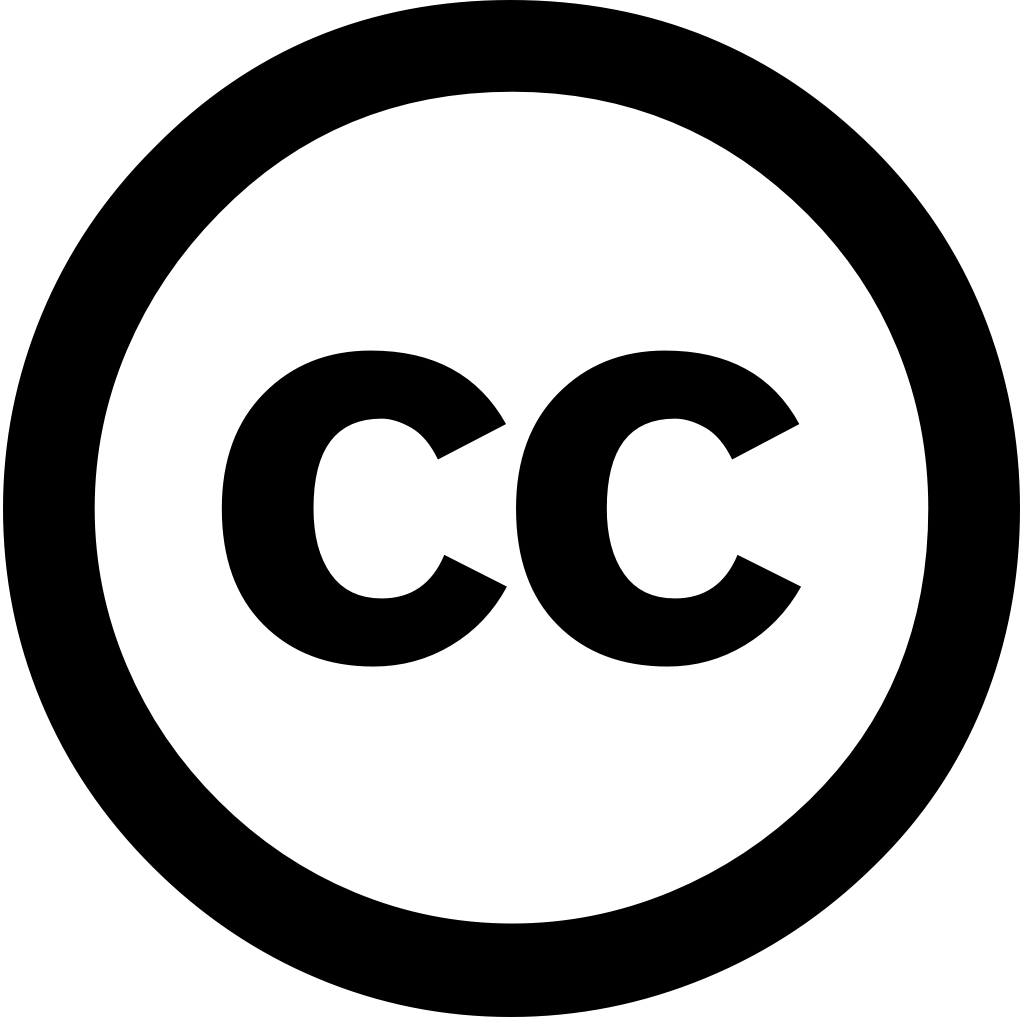
BMC Health Services Research, Journal Year: 2025, Volume and Issue: 25(1)
Published: March 27, 2025
The time a patient spends in the hospital from admission to discharge is known as length of stay (LOS). Predicting LOS crucial for enhancing care, managing resources, and optimizing use beds. Therefore, this study aimed predict patients hospitalized various clinics using different artificial intelligence (AI) models. analyzed 162,140 aged 18 older at university northern Türkiye 2012 2020. Three soft computing methods—Artificial Neural Networks (ANN), Adaptive Neuro-Fuzzy Inference Systems (ANFIS), Multiple Linear Regression Analysis (MLR)—were employed estimate inputs such medical imaging services (number CT, USG, ECG, hemogram tests, biochemistry, number direct x-rays), demographic, diagnostic data (patients' age, sex, season hospitalization, type diagnosis, second diagnosis). predictions utilized single double-hidden layer ANNs with training algorithms (Levenberg-Marquardt-LM, Bayesian Regularization-BR Scaled Conjugate Gradient-SCG) activation functions (tangent-sigmoid, purelin), ANFIS Grid Partitioning (ANFIS-GP), MLR. Model performance was evaluated Coefficient Determination (R²), Root Mean Square Error (RMSE) Absolute (MAE). Of patients, 54% were male 43.5% treated surgical clinics. mean age 55.1 years, 32.9% participants 65 years or older. Hospital stays 2–7 days 39.7% over 7 30.9%, 1 day 29.4%. Neoplasm-related diagnoses (ICD codes) accounted 25.1% admissions. Variables influencing identified through feature selection wards. most significant factors affecting include computerized tomography scans (CT), ultrasonography (USG), X-rays. Utilizing these factors, 12 models varied input variables developed analyzed. double hidden ANN model Levenberg-Marquardt (LM) algorithm outperformed others, achieving R² values 0.854 0.807 test dataset, RMSE 2.397 2.774 MAE 1.787 1.994 days, respectively. Following ANN-LM, best results obtained ANFIS-GP, while MLR exhibited lowest performance. Various AI can effectively units. Accurate help health managers allocate resources more equitably across
Language: Английский