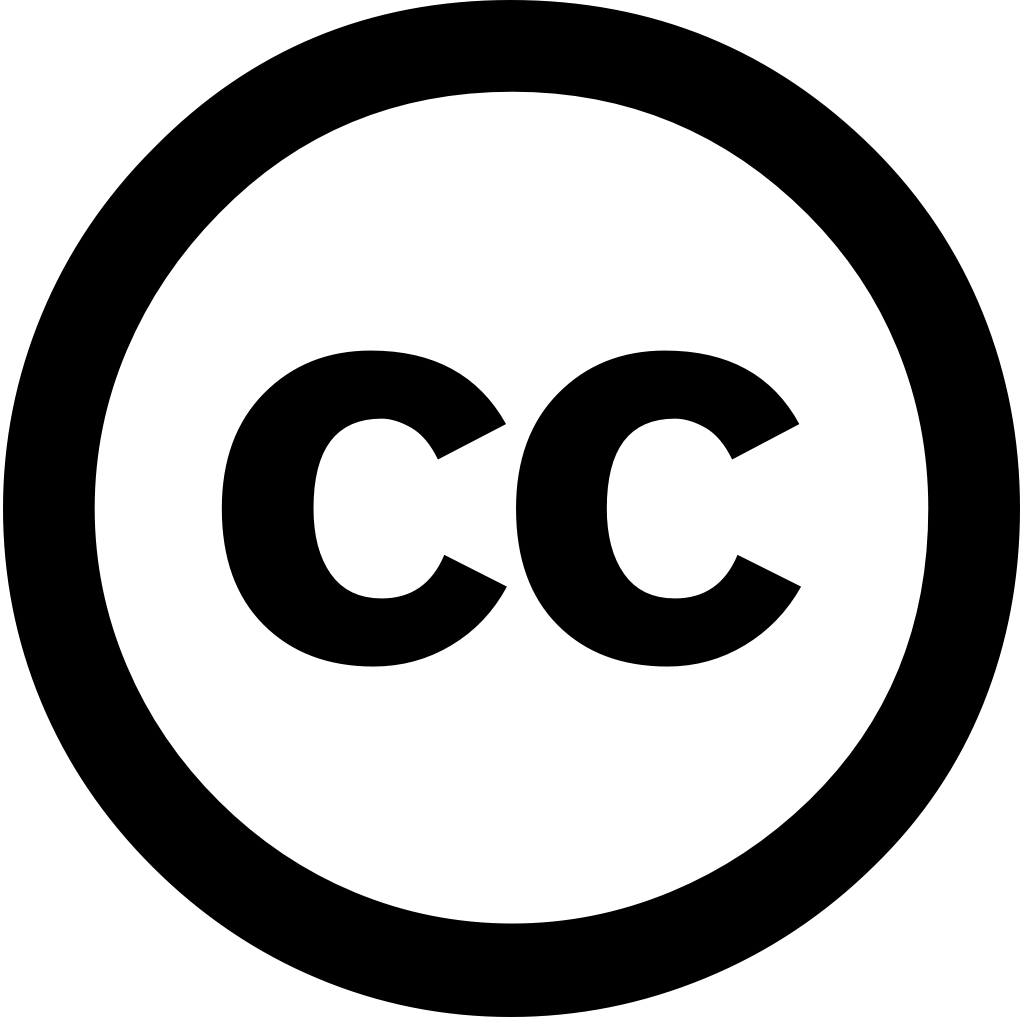
Journal of Imaging, Journal Year: 2025, Volume and Issue: 11(4), P. 101 - 101
Published: March 28, 2025
Generative adversarial networks (GANs) prioritize pixel-level attributes over capturing the entire image distribution, which is critical in synthesis. To address this challenge, we propose a dual-stream contrastive latent projection generative network (DSCLPGAN) for robust augmentation of MRI images. The generator our architecture incorporates two specialized processing pathways: one dedicated to local feature variation modeling, while other captures global structural transformations, ensuring more comprehensive synthesis medical We used transformer-based encoder-decoder framework contextual coherence and learning (CLP) module integrates loss into space generating diverse samples. generated images undergo refinement using an ensemble discriminators, where discriminator 1 (D1) ensures classification consistency with real images, 2 (D2) produces probability map localized variations, 3 (D3) preserves consistency. For validation, utilized publicly available dataset contains 3064 T1-weighted contrast-enhanced three types brain tumors: meningioma (708 slices), glioma (1426 pituitary tumor (930 slices). experimental results demonstrate state-of-the-art performance, achieving SSIM 0.99, accuracy 99.4% diversity level 5, PSNR 34.6 dB. Our approach has potential high-fidelity augmentations reliable AI-driven clinical decision support systems.
Language: Английский