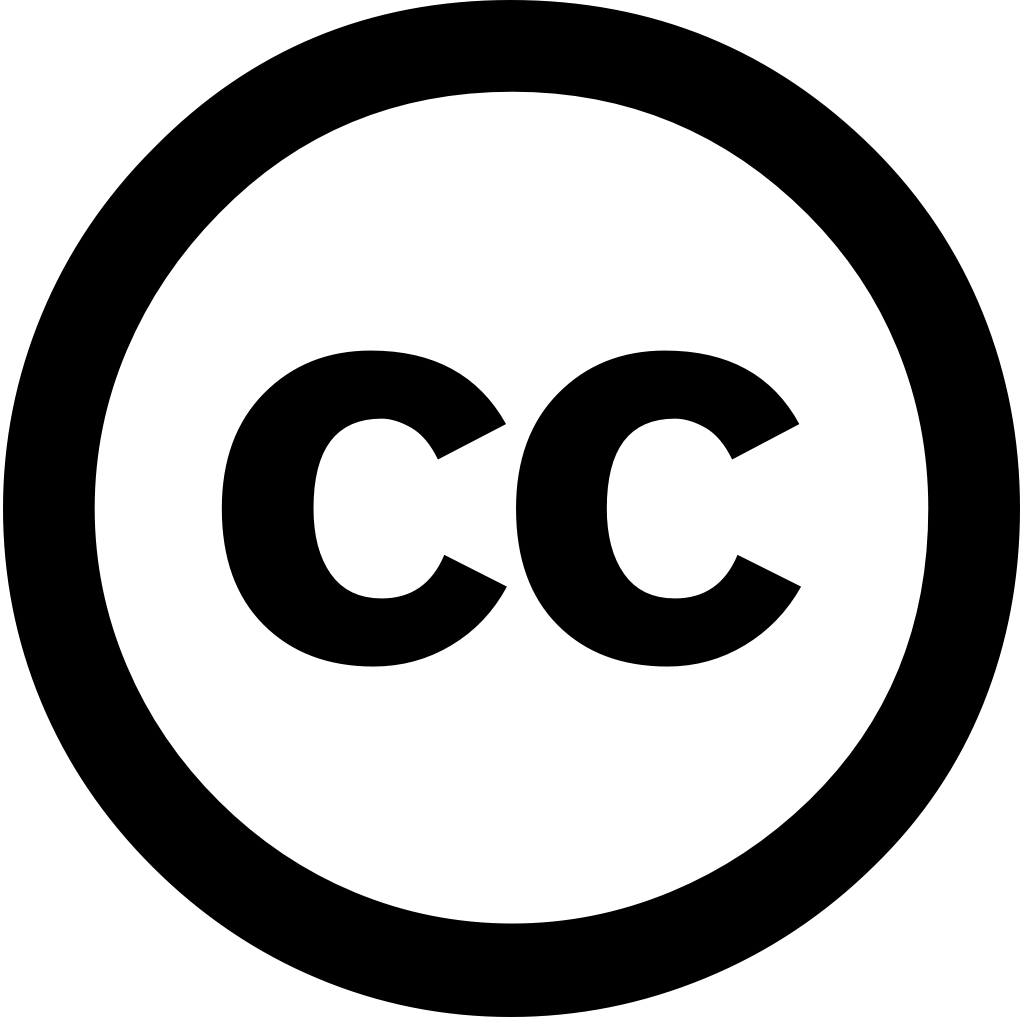
Neurocomputing, Journal Year: 2024, Volume and Issue: 599, P. 128073 - 128073
Published: June 15, 2024
In the rapidly evolving domain of machine learning, ability to adapt unforeseen circumstances and novel data types is paramount importance. The deployment Artificial Intelligence progressively aimed at more realistic open scenarios where data, tasks, conditions are variable not fully predetermined, therefore a closed set assumption cannot be hold. such environments, learning asked autonomous, continuous, adaptive, requiring effective management uncertainty unknown fulfill expectations. response, there vigorous effort develop new generation models, which characterized by enhanced autonomy broad capacity generalize, enabling them perform effectively across wide range tasks. field in environments poses many challenges also brings together different paradigms, some traditional but others emerging, overlapping confusion between makes it difficult distinguish or give necessary relevance. This work delves into frontiers methodologies that thrive these identifying common practices, limitations, connections paradigms Open-Ended Learning, Open-World Open Set Recognition, other related areas as Continual Out-of-Distribution detection, Novelty Detection, Active Learning. We seek easy understanding fields their roots, uncover problems suggest several research directions may motivate articulate future efforts towards robust autonomous systems.
Language: Английский