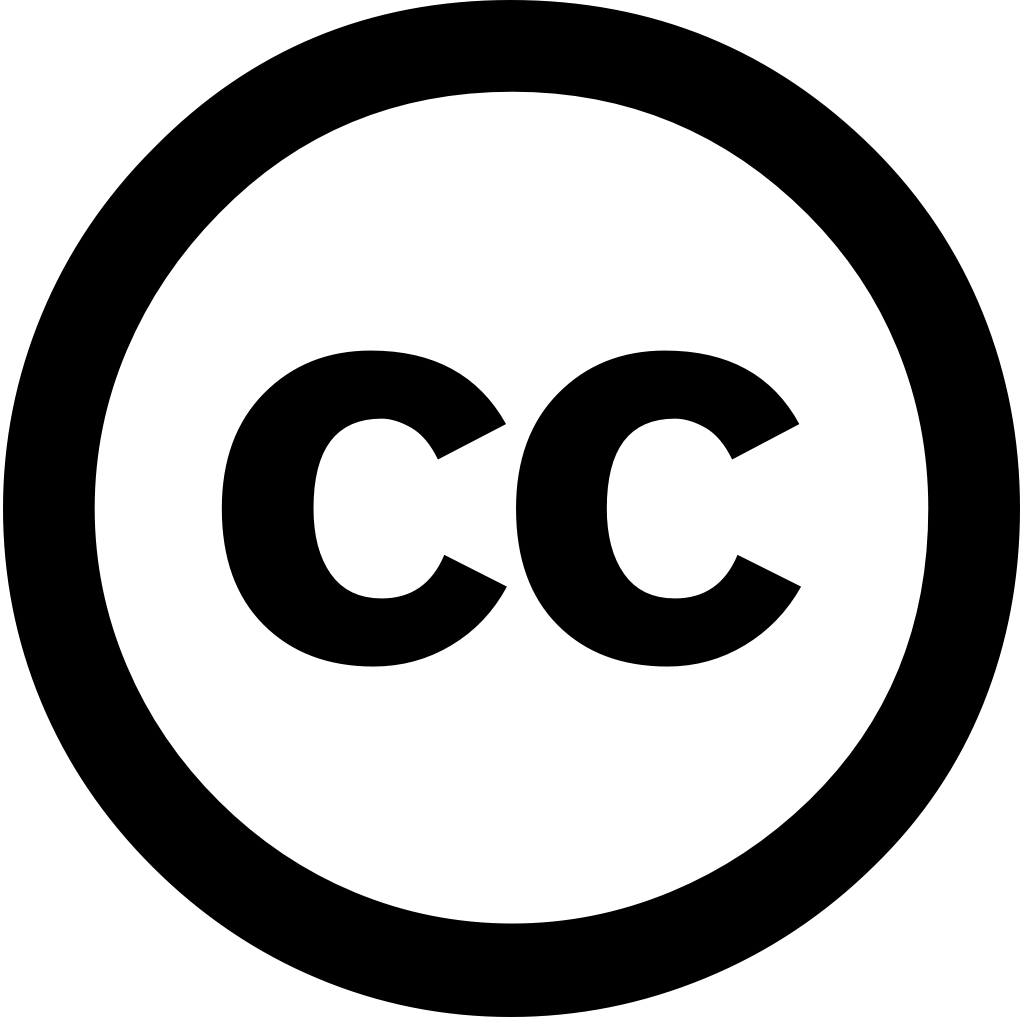
Sensors, Journal Year: 2024, Volume and Issue: 24(22), P. 7318 - 7318
Published: Nov. 16, 2024
The efficient separation of coal and gangue remains a critical challenge in modern mining, directly impacting energy efficiency, environmental protection, sustainable development. Current machine vision-based sorting methods face significant challenges dense scenes, where label rewriting problems severely affect model performance, particularly when are closely distributed conveyor belt images. This paper introduces CGDet (Coal Gangue Detection), novel compact convolutional neural network that addresses these through two key innovations. First, we proposed an Object Distribution Density Measurement (ODDM) method to quantitatively analyze the distribution density gangue, enabling optimal selection input feature map resolutions mitigate issues. Second, developed Relative Resolution Scale (RROSM) assess object scales, guiding design streamlined fusion structure eliminates redundant components while maintaining detection accuracy. Experimental results demonstrate effectiveness our approach; achieved superior performance with AP50 AR50 scores 96.7% 99.2% respectively, reducing parameters by 46.76%, computational cost 47.94%, inference time 31.50% compared traditional models. These improvements make suitable for real-time underground mining environments, resources limited but high accuracy is essential. Our work provides new perspective on designing yet high-performance networks scene applications.
Language: Английский