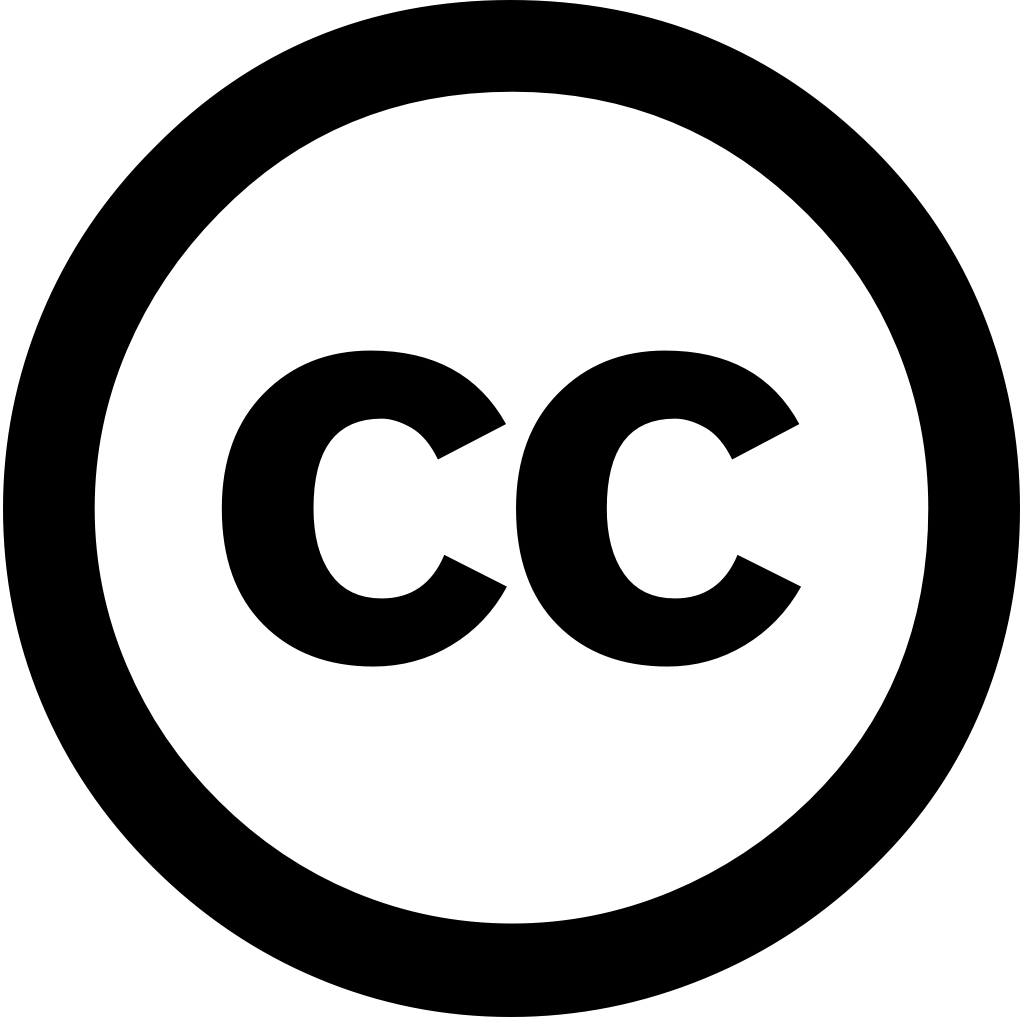
IEEE Open Journal of the Computer Society, Journal Year: 2024, Volume and Issue: 5, P. 705 - 723
Published: Jan. 1, 2024
Language: Английский
IEEE Open Journal of the Computer Society, Journal Year: 2024, Volume and Issue: 5, P. 705 - 723
Published: Jan. 1, 2024
Language: Английский
Information, Journal Year: 2025, Volume and Issue: 16(3), P. 195 - 195
Published: March 3, 2025
Deep convolutional neural networks (CNNs) have revolutionized medical image analysis by enabling the automated learning of hierarchical features from complex imaging datasets. This review provides a focused CNN evolution and architectures as applied to analysis, highlighting their application performance in different fields, including oncology, neurology, cardiology, pulmonology, ophthalmology, dermatology, orthopedics. The paper also explores challenges specific outlines trends future research directions. aims serve valuable resource for researchers practitioners healthcare artificial intelligence.
Language: Английский
Citations
1IEEE Access, Journal Year: 2024, Volume and Issue: 12, P. 115411 - 115447
Published: Jan. 1, 2024
The rapid advancement of technology has set higher standards for the next generation wireless communication networks, known as 6G. These networks go beyond simple task connecting devices and aim to establish a self-sustaining system within society. One key factors in achieving this goal is integration AI services apps through Internet Things (IoT), which will be made possible with support 6G technology. artificial intelligence (AI) play crucial role enhancing protocols, architectures, operations networks. To achieve collaborative IoT applications, Federated Learning (FL) emerged popular method. FL enables training without need data sharing, ensuring privacy security. However, also faces challenges, such presence malicious risk single-point failure. address these concerns, blockchain (BCT) offers secure efficient solution. By leveraging blockchain, issues can effectively tackled, providing reliable framework implementing FL-IoT applications.
Language: Английский
Citations
7Future Internet, Journal Year: 2025, Volume and Issue: 17(4), P. 140 - 140
Published: March 25, 2025
The rapid expansion of IoT devices has introduced major challenges in ensuring data interoperability, enabling real-time processing, and achieving scalability, especially decentralized edge computing environments. In this paper, an advanced framework FedProx with ontology-driven standardization is proposed, which can meet such comprehensively. On the one hand, it guarantee semantic consistency across different kinds using unified ontology, so that from multiple sources could be seamlessly integrated; on other solves non-IID issues limited resources servers by FedProx. Experimental findings indicate outperforms FedAvg, a remarkable accuracy level 89.4%, having higher convergence rates, attaining 30% saving communication overhead through gradient compression. addition, ontology alignment procedure yielded 95% success rate, thereby uniform preprocessing domains, including traffic monitoring parking management. model demonstrates outstanding scalability flexibility to new devices, while maintaining high performance during evolution. These highlight its great potential for deployment smart cities, environmental monitoring, IoT-based ecosystems, creation more efficient integrated solutions these areas.
Language: Английский
Citations
0Lecture notes in computer science, Journal Year: 2025, Volume and Issue: unknown, P. 205 - 216
Published: Jan. 1, 2025
Language: Английский
Citations
0Artificial Intelligence Review, Journal Year: 2025, Volume and Issue: 58(8)
Published: May 3, 2025
Language: Английский
Citations
0ACM Transactions on Intelligent Systems and Technology, Journal Year: 2024, Volume and Issue: unknown
Published: Sept. 2, 2024
Despite achieving remarkable performance, Federated Learning (FL) encounters two important problems, i.e., low training efficiency and limited computational resources. In this paper, we propose a new FL framework, FedDUMAP, with three original contributions, to leverage the shared insensitive data on server in addition distributed edge devices so as efficiently train global model. First, simple dynamic update algorithm, which takes advantage of while dynamically adjusting steps order speed up convergence improve accuracy. Second, an adaptive optimization method algorithm exploit momentum each local device for superior Third, develop layer-adaptive model pruning carry out specific operations, is adapted diverse features layer attain excellent trade-off between effectiveness efficiency. Our proposed model, combines techniques has significantly better performance compared baseline approaches terms (up 16.9 times faster), accuracy 20.4% higher), cost 62.6% smaller).
Language: Английский
Citations
3Knowledge and Information Systems, Journal Year: 2024, Volume and Issue: unknown
Published: Nov. 26, 2024
Language: Английский
Citations
2IEEE Open Journal of the Computer Society, Journal Year: 2024, Volume and Issue: 5, P. 705 - 723
Published: Jan. 1, 2024
Language: Английский
Citations
0