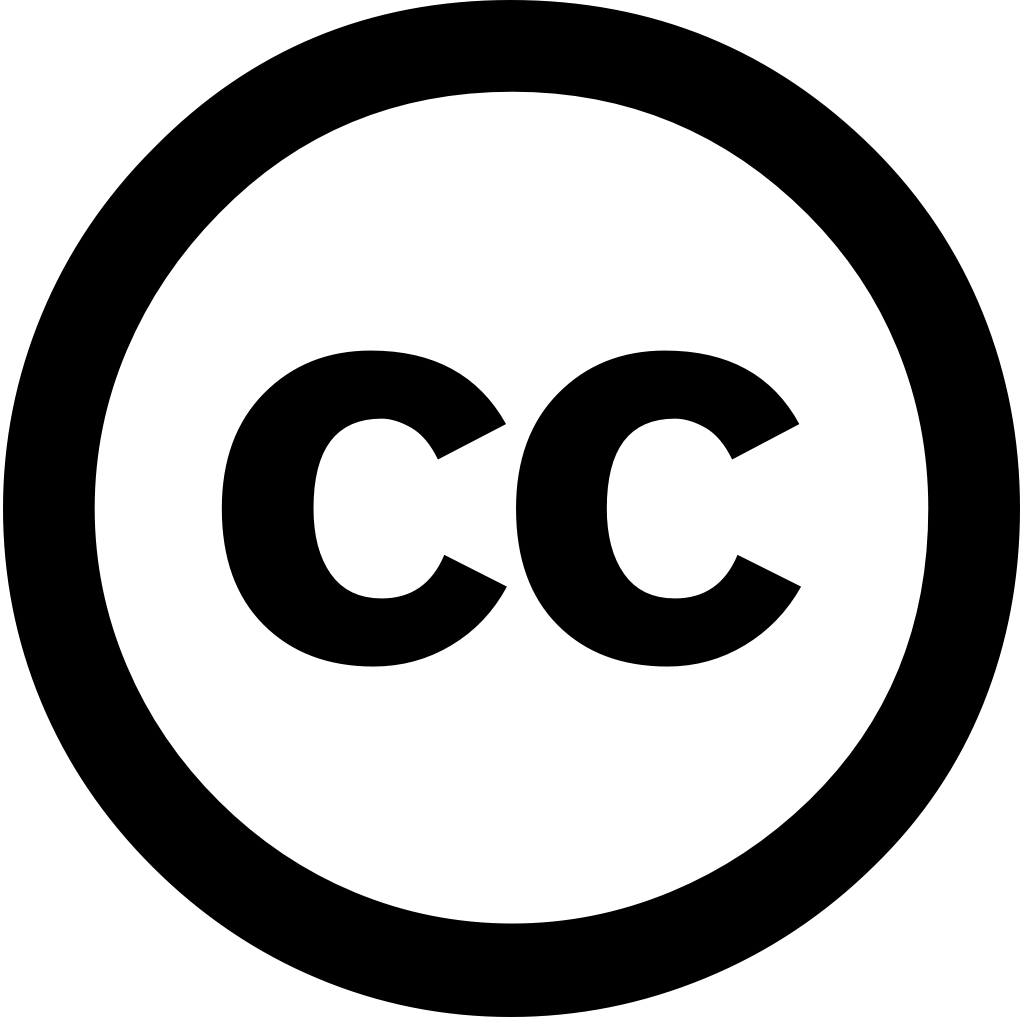
Journal of Multidisciplinary Healthcare, Journal Year: 2025, Volume and Issue: Volume 18, P. 319 - 327
Published: Jan. 1, 2025
Triple-negative breast cancer (TNBC) is a unique subtype characterized by the lack of estrogen receptor (ER), progesterone (PR), and human epidermal growth factor 2 (HER2) expression in tumor cells. TNBC represents about 15% to 20% all cancers aggressive highly malignant. Currently, diagnosis primarily depends on pathological examination, while treatment efficacy assessed through imaging, biomarker detection, evaluation, clinical symptom improvement. Among these, detection assessments are invasive, time-intensive procedures that may be difficult for patients with severe comorbidities high complication risks. Thus, there an urgent need new, supportive tools treatment. Deep learning radiomics techniques represent advanced machine methodologies also emerging outcomes medical-engineering field recent years. They extensions conventional imaging diagnostic methods have demonstrated tremendous potential image segmentation, reconstruction, recognition, classification. These hold certain application prospects TNBC, assessment response, long-term prognosis prediction. This article reviews progress deep learning, ultrasound, MRI, treatment, based research from both domestic international scholars.
Language: Английский