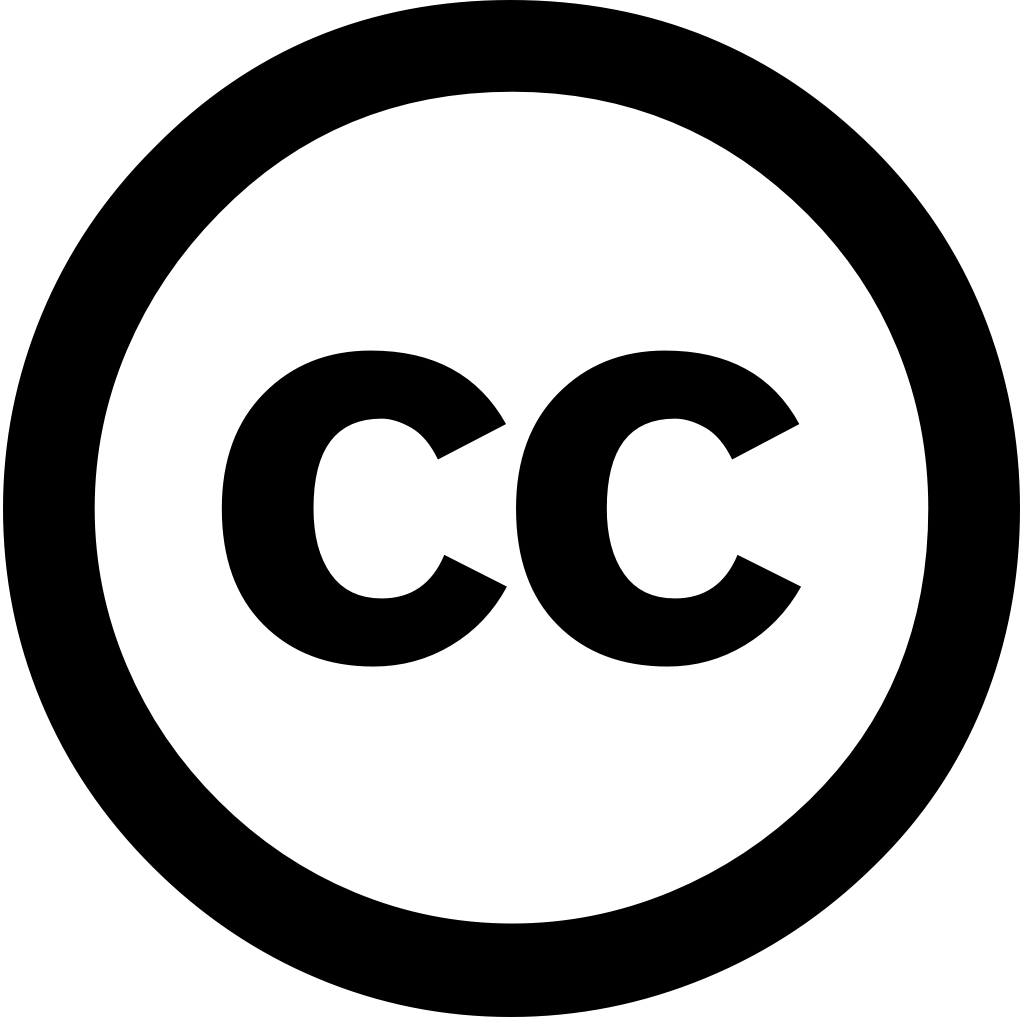
Research Square (Research Square), Journal Year: 2024, Volume and Issue: unknown
Published: Nov. 28, 2024
Language: Английский
Research Square (Research Square), Journal Year: 2024, Volume and Issue: unknown
Published: Nov. 28, 2024
Language: Английский
Artificial Intelligence Review, Journal Year: 2025, Volume and Issue: 58(5)
Published: Feb. 24, 2025
Language: Английский
Citations
0Journal of Clinical Medicine, Journal Year: 2025, Volume and Issue: 14(7), P. 2442 - 2442
Published: April 3, 2025
Obsessive-compulsive disorder (OCD) is a complex psychiatric condition characterized by significant heterogeneity in symptomatology and treatment response. Advances neuroimaging, EEG, other multimodal datasets have created opportunities to identify biomarkers predict outcomes, yet traditional statistical methods often fall short analyzing such high-dimensional data. Deep learning (DL) offers powerful tools for addressing these challenges leveraging architectures capable of classification, prediction, data generation. This brief review provides an overview five key DL architectures-feedforward neural networks, convolutional recurrent generative adversarial transformers-and their applications OCD research clinical practice. We highlight how models been used the predictors response, diagnose classify OCD, advance precision psychiatry. conclude discussing implementation DL, summarizing its advances promises underscoring field.
Language: Английский
Citations
0Research Square (Research Square), Journal Year: 2024, Volume and Issue: unknown
Published: Nov. 28, 2024
Language: Английский
Citations
0