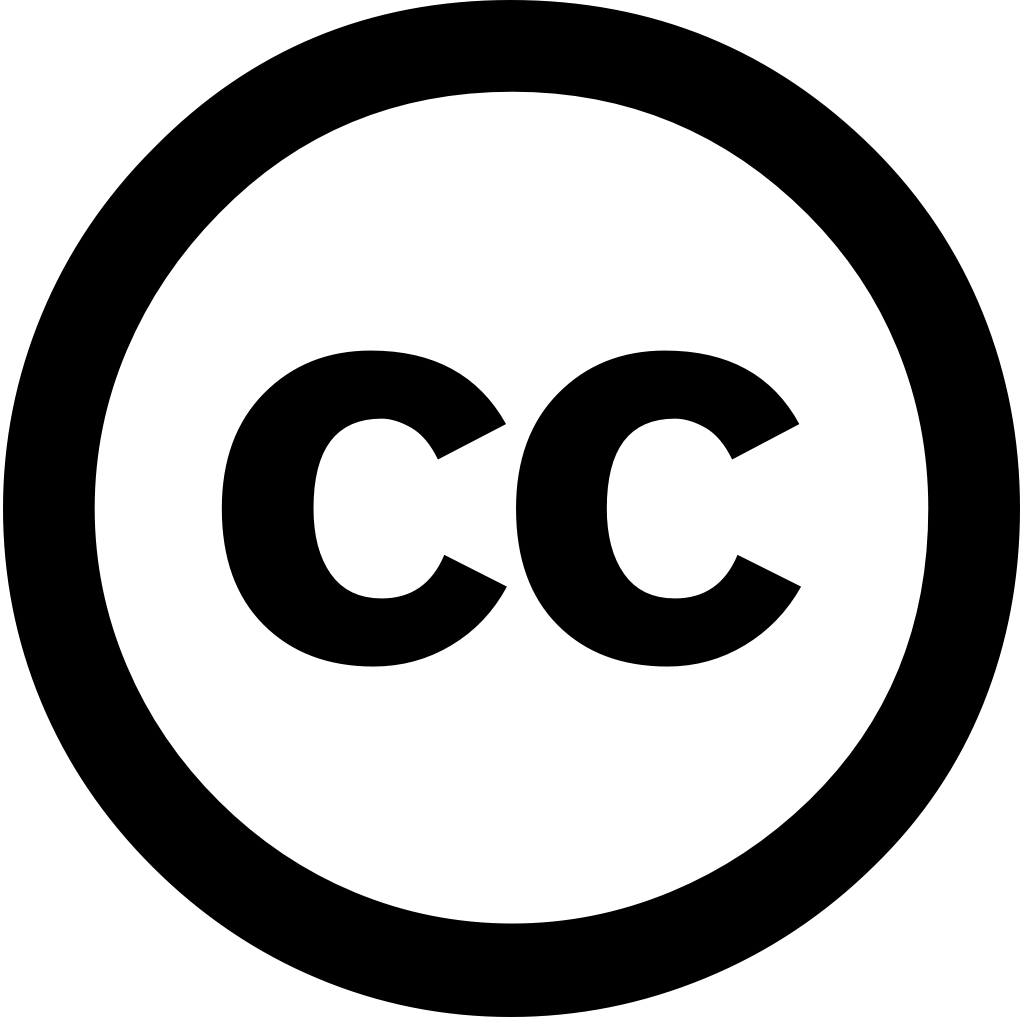
Urban Science, Journal Year: 2025, Volume and Issue: 9(5), P. 138 - 138
Published: April 23, 2025
Air pollution presents significant risks to both human health and the environment. This study uses air meteorological data develop an effective deep learning model for hourly PM2.5 concentration predictions in Tehran, Iran. evaluates efficient metaheuristic algorithms optimizing hyperparameters improve accuracy of predictions. The optimal feature set was selected using Variance Inflation Factor (VIF) Boruta-XGBoost methods, which indicated elimination NO, NO2, NOx. highlighted PM10 as most important feature. Wavelet transform then applied extract 40 features enhance prediction accuracy. Hyperparameters weights matrices Echo State Network (ESN) were determined algorithms, with Salp Swarm Algorithm (SSA) demonstrating superior performance. evaluation different criteria revealed that ESN-SSA outperformed other hybrids original ESN, LSTM, GRU models.
Language: Английский