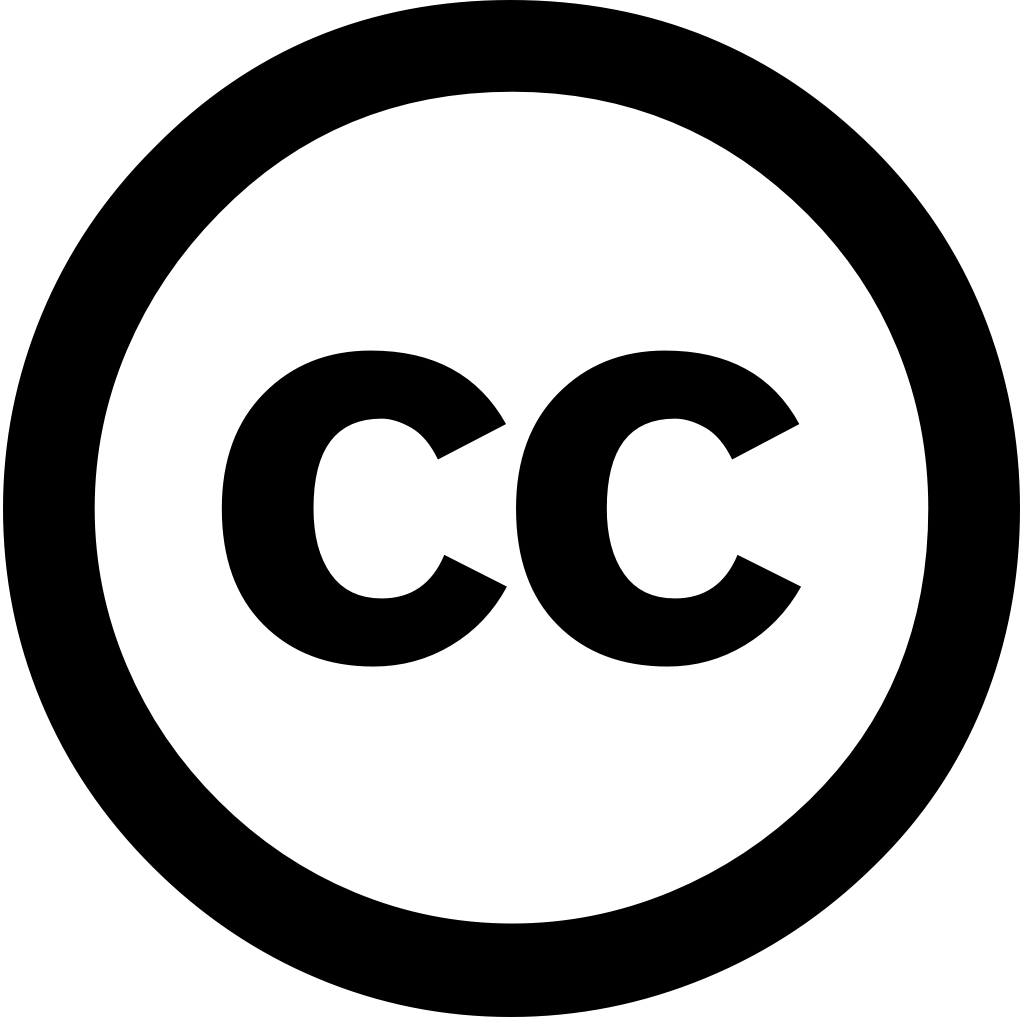
Statistics and Computing, Journal Year: 2025, Volume and Issue: 35(3)
Published: March 13, 2025
Abstract Surrogate models are statistical or conceptual approximations for more complex simulation models. In this context, it is crucial to propagate the uncertainty induced by limited budget and surrogate approximation error predictions, inference, subsequent decision-relevant quantities. However, quantifying then propagating of surrogates usually special analytic cases otherwise computationally very expensive. paper, we propose a framework enabling scalable, Bayesian approach modeling with thorough quantification, propagation, validation. Specifically, present three methods inference given measurement data. This task where propagation especially relevant, because failing account may lead biased and/or overconfident estimates parameters interest. We showcase our in detailed case studies linear nonlinear real-world scenarios. Uncertainty enables reliable safe expensive simulators will therefore be useful various fields applications.
Language: Английский