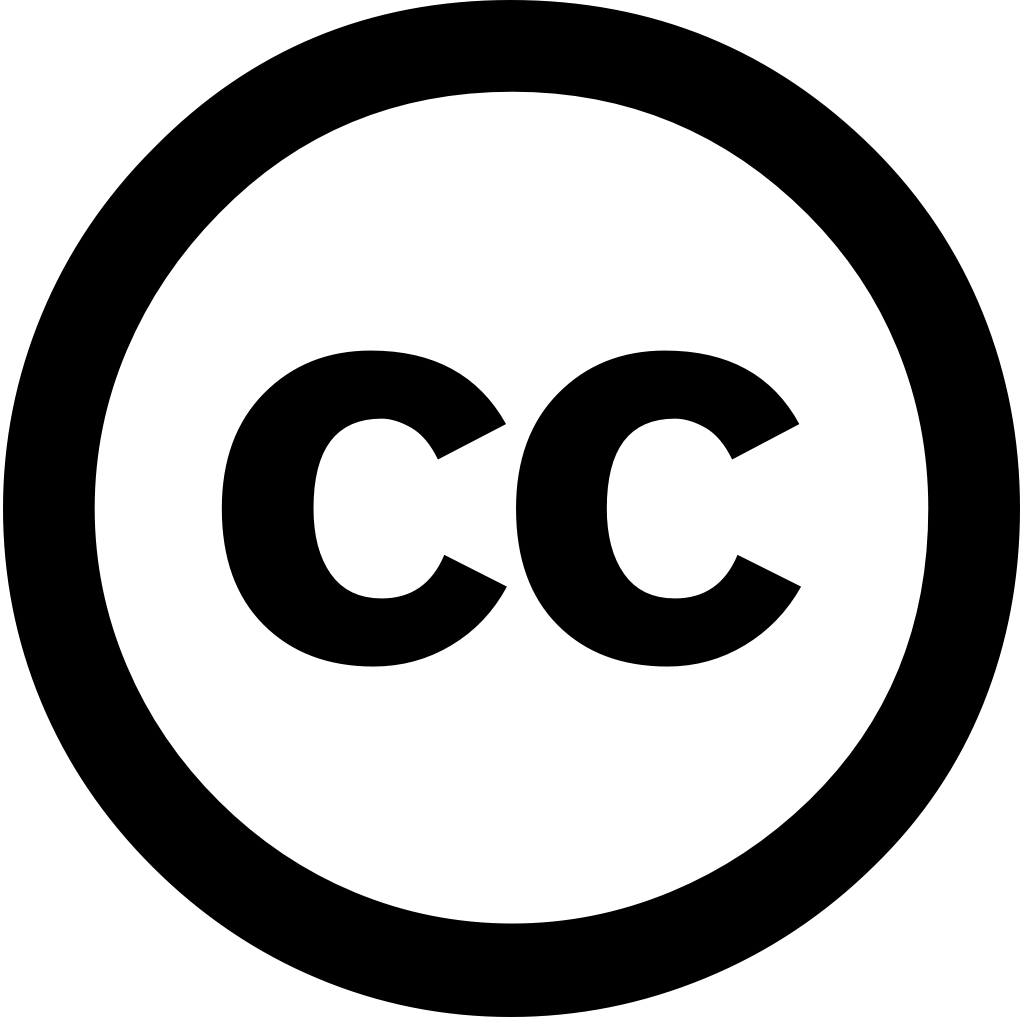
Micromachines, Journal Year: 2025, Volume and Issue: 16(5), P. 504 - 504
Published: April 26, 2025
Machining processes often face challenges such as elevated temperatures and wear, which traditional cutting fluids are insufficient to address. As a result, solutions involving nanoparticle additives being explored enhance cooling lubrication performance. This study investigates the effect of thermal conductivity, an important property influenced by densities mono hybrid nanofluids. To this end, various nanofluids were prepared incorporating hexagonal boron nitride (hBN), zinc oxide (ZnO), multi-walled carbon nanotubes (MWCNTs), titanium dioxide (TiO2), aluminum (Al2O3) nanoparticles into sunflower oil base fluid. Hybrid created combining two nanoparticles, including ZnO + MWCNT, hBN ZnO, TiO2, Al2O3, TiO2 Al2O3. A dataset consisting 180 data points was generated measuring conductivity density at (30–70 °C) in laboratory setting. Conducting measurements across different temperature ranges presents significant challenges, requiring considerable time resources, resulting high costs potential inaccuracies. address these issues, feedforward artificial neural network (FFANN) method proposed predict conductivity. Our multilayer FFANN model takes input experimental environment where measurement is made, measured relevant nanoparticle, relative nanoparticle. The predicts value linearly output. demonstrated predictive accuracy, with reliability R = 0.99628 coefficient determination (R2) 0.9999. average mean absolute error (MAE) for all 0.001, squared (MSE) 1.76 × 10−6. provides State-of-the-Art approach predicting offering valuable insights selecting optimal based on values density.
Language: Английский