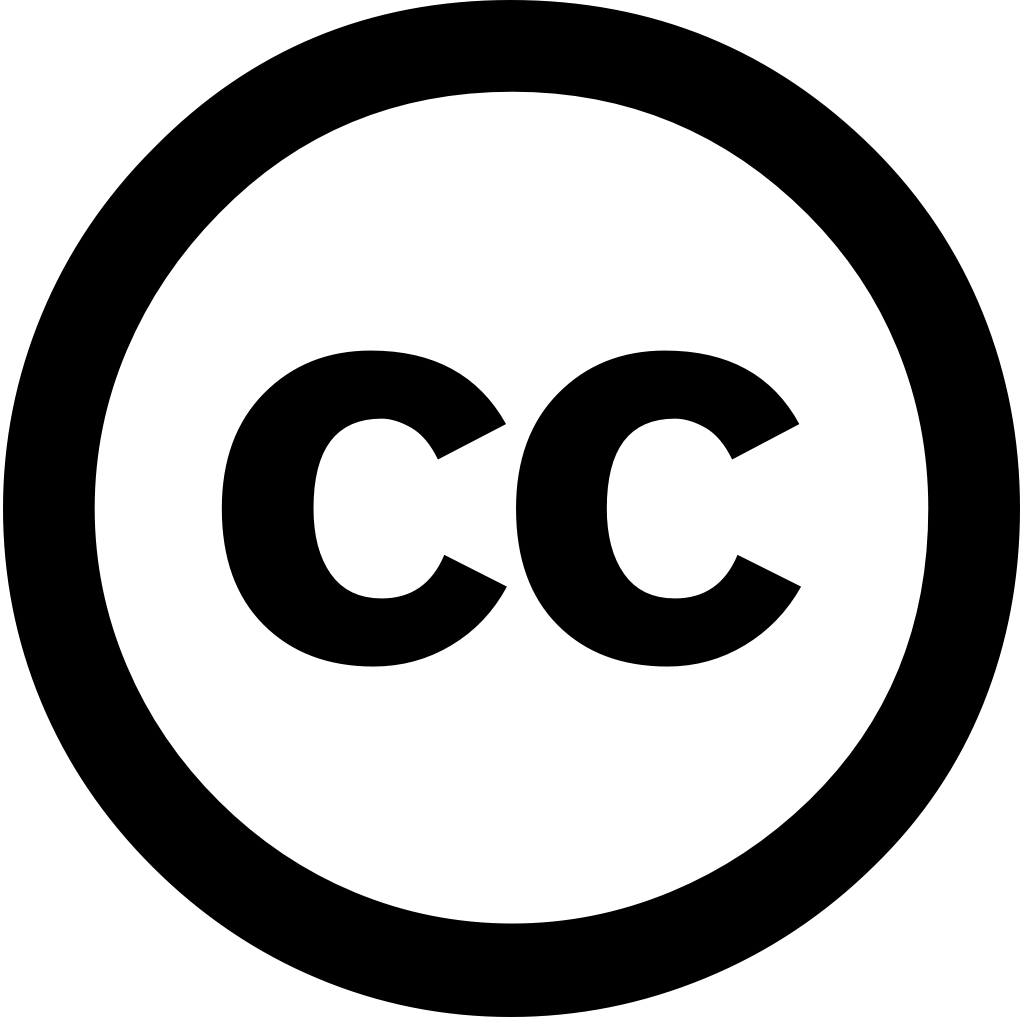
PLoS ONE, Journal Year: 2025, Volume and Issue: 20(5), P. e0320565 - e0320565
Published: May 7, 2025
The development of lightweight, corrosion-resistant metallic lattice structures has gained significant attention in aerospace, defense, and structural applications, where material durability weight optimization are critical. This study investigates the corrosion behavior Laser Powder Bed Fusion (LPBF)-fabricated A286 steel honeycomb, Body-Centered Cubic (BCC), gyroid lattices, comparing their performance against conventional materials such as Rolled Homogeneous Armor (RHA), Maraging High Strength Steel (MHA), High-Nitrogen (HNS). Corrosion testing was conducted using accelerated salt spray exposure, results were validated through computed tomography (CT)-based integrity analysis machine learning-based predictive modeling. experimental findings revealed that exhibited significantly lower rates than bulk materials, with honeycomb demonstrating highest resistance (1.218 mm/year), followed by BCC (1.311 mm/year) (1.671 mm/year). Compared to RHA, a 57.23% reduction rate, confirming its superior electrochemical stability. CT scan evaluations further highlighted differences density distribution geometric fidelity, lattices showing most uniform porosity, while displayed localized variations at nodal intersections. To enhance capabilities, various learning (ML) algorithms employed model based on weight-loss measurements topology. Bayesian Ridge regression outperformed other models, achieving an R² 0.99849 RMSE 0.00049, robustness capturing trends. Linear Regression also performed well, ensemble models Random Forest XGBoost higher error margins due dataset linearity constraints. Residual graphical interpretations stability reliability ML-based assessments, feasibility alternative traditional methods. presents comprehensive framework for integrating testing, computational modeling, CT-based defect analysis, offering scalable approach optimizing micro-lattice designs corrosion-sensitive applications. highlight potential LPBF-fabricated marine structures, enhanced resistance, reduced degradation, maintenance strategies essential long-term operational performance.
Language: Английский