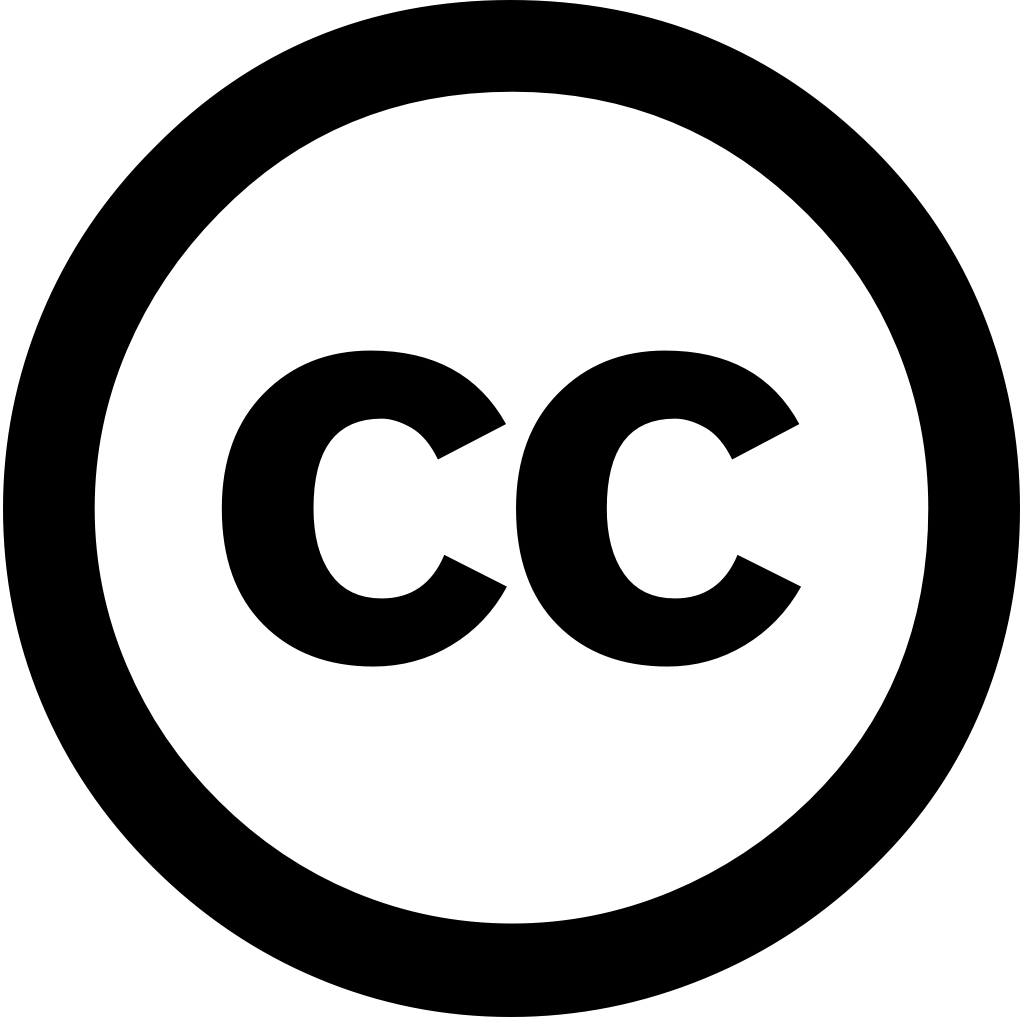
PLoS ONE, Journal Year: 2025, Volume and Issue: 20(3), P. e0316600 - e0316600
Published: March 10, 2025
Despite the advantages of additive manufacturing, its widespread adoption is still hindered by poor quality fabricated parts. Advanced machine learning techniques to predict part can improve repeatability and open manufacturing various industries. This study aims accurately relative density, surface roughness hardness AlSi10Mg samples produced selective laser melting regarding process parameters such as scan speed, layer thickness, power, hatch distance. For this purpose, data including porosity, hardness, were extracted from literature, additional measurements performed on manufactured in current work. work compares five supervised algorithms, artificial neural networks, support vector regression, kernel ridge random forest, Lasso regression. These models are evaluated based coefficient determination mean squared error. On basis computational results, network outperformed predicting roughness, hardness. Feature importance analysis compiled dataset using ANN revealed that power speed most important features affecting density (e.g., porosity) while thickness significantly impact The identified an optimal region achieves a > 99%, < 10 µm, 120 HV. results presented provide significant for potentially reducing experimentation costs identifying optimize
Language: Английский