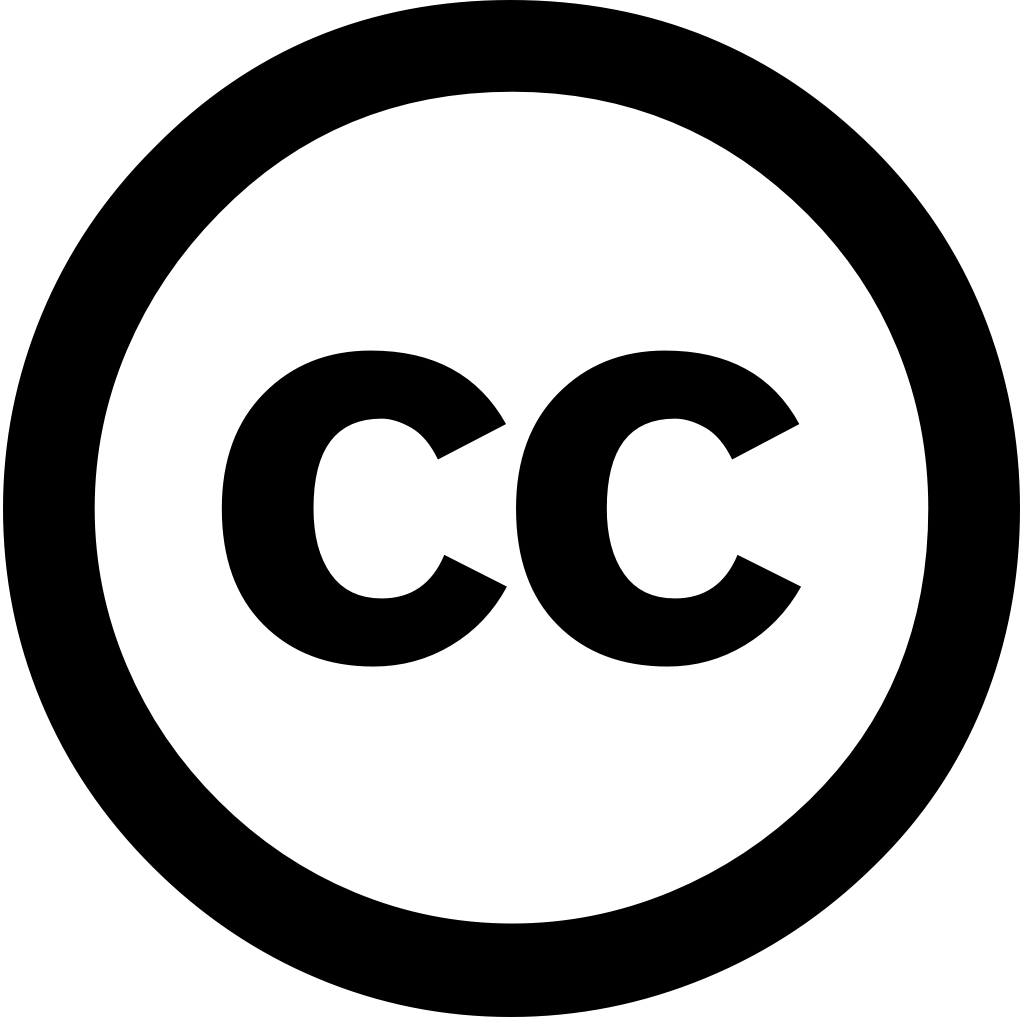
Machine Learning and Knowledge Extraction, Journal Year: 2025, Volume and Issue: 7(2), P. 33 - 33
Published: April 5, 2025
Regression is a fundamental task in machine learning, and neural networks have been successfully employed many applications to identify underlying regression patterns. However, they are often criticised for their lack of interpretability commonly referred as black-box models. Feature selection approaches address this challenge by simplifying datasets through the removal unimportant features, while improving explainability revealing feature importance. In work, we leverage mathematical programming most important features trained deep network with ReLU activation function, providing greater insight into its decision-making process. Unlike traditional methods, our approach adjusts weights biases via Mixed-Integer Linear Programming (MILP) model thereby uncover relationships. The formulation reported, which determines subset selected clustering applied reduce complexity model. Our results illustrate improved performance when implemented proposed approach, compared other approaches. Finally, analysis frequency across each dataset reveals contribution predictions, addressing nature network.
Language: Английский