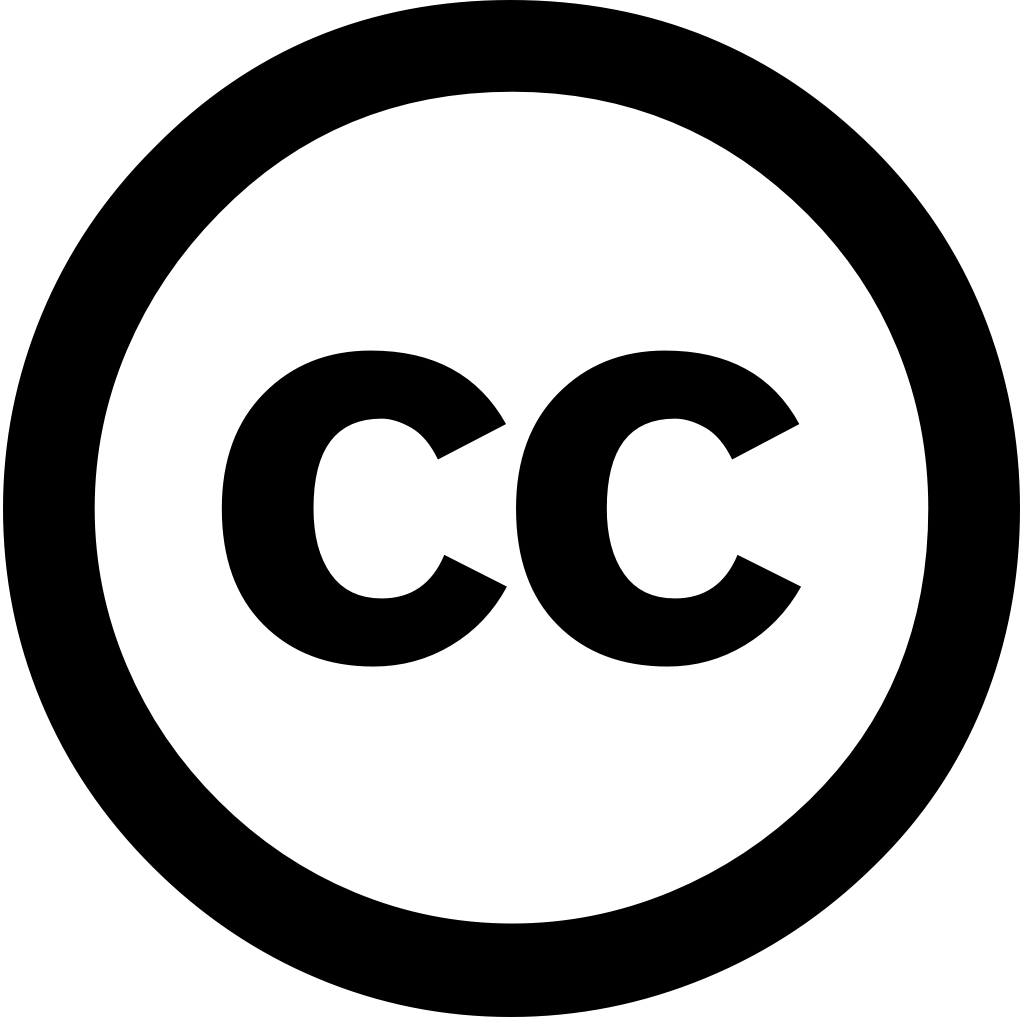
Published: July 17, 2023
<p>A systematic Review of YOLO for medical Object Detection (2018 - 2023)</p>
Language: Английский
Published: July 17, 2023
<p>A systematic Review of YOLO for medical Object Detection (2018 - 2023)</p>
Language: Английский
Machine Learning and Knowledge Extraction, Journal Year: 2023, Volume and Issue: 5(4), P. 1680 - 1716
Published: Nov. 20, 2023
YOLO has become a central real-time object detection system for robotics, driverless cars, and video monitoring applications. We present comprehensive analysis of YOLO’s evolution, examining the innovations contributions in each iteration from original up to YOLOv8, YOLO-NAS, with transformers. start by describing standard metrics postprocessing; then, we discuss major changes network architecture training tricks model. Finally, summarize essential lessons development provide perspective on its future, highlighting potential research directions enhance systems.
Language: Английский
Citations
890International Journal of Computational Intelligence Systems, Journal Year: 2023, Volume and Issue: 16(1)
Published: Aug. 2, 2023
Abstract Object detection is a critical and complex problem in computer vision, deep neural networks have significantly enhanced their performance the last decade. There are two primary types of object detectors: stage one stage. Two-stage detectors use architecture to select regions for detection, while one-stage can detect all potential single shot. When evaluating effectiveness an detector, both accuracy inference speed essential considerations. usually outperform terms accuracy. However, YOLO its predecessor architectures substantially improved In some scenarios, at which produce inferences more than This study explores metrics, regression formulations, single-stage detectors. Additionally, it briefly discusses various variations, including design, performance, cases.
Language: Английский
Citations
98IEEE Access, Journal Year: 2024, Volume and Issue: 12, P. 57815 - 57836
Published: Jan. 1, 2024
YOLO (You Only Look Once) is an extensively utilized object detection algorithm that has found applications in various medical tasks. This been accompanied by the emergence of numerous novel variants recent years, such as YOLOv7 and YOLOv8. study encompasses a systematic exploration PubMed database to identify peer-reviewed articles published between 2018 2023. The search procedure 124 relevant studies employed for diverse tasks including lesion detection, skin classification, retinal abnormality identification, cardiac brain tumor segmentation, personal protective equipment detection. findings demonstrated effectiveness outperforming alternative existing methods these However, review also unveiled certain limitations, well-balanced annotated datasets, high computational demands. To conclude, highlights identified research gaps proposes future directions leveraging potential
Language: Английский
Citations
49Machines, Journal Year: 2023, Volume and Issue: 11(2), P. 246 - 246
Published: Feb. 7, 2023
Forest fires are a serious ecological concern, and smoke is an early warning indicator. Early images barely capture tiny portion of the total smoke. Because irregular nature smoke’s dispersion dynamic surrounding environment, identification complicated by minor pixel-based traits. This study presents new framework that decreases sensitivity various YOLO detection models. Additionally, we compare performance speed different models such as YOLOv3, YOLOv5, YOLOv7 with prior ones Fast R-CNN Faster R-CNN. Moreover, follow use collected dataset describes three distinct areas, namely close, medium, far distance, to identify model’s ability recognize targets correctly. Our model outperforms gold-standard method on multi-oriented for detecting forest mAP accuracy 96.8% at IoU 0.5 using YOLOv5x. findings show extensive improvement in several data-augmentation techniques. YOLOv3 95%, compared 94.8% SGD optimizer. Extensive research shows suggested achieves significantly better results than most advanced object-detection algorithms when used datasets from wildfires, while maintaining satisfactory level challenging environmental conditions.
Language: Английский
Citations
47Computers and Electronics in Agriculture, Journal Year: 2024, Volume and Issue: 217, P. 108612 - 108612
Published: Jan. 5, 2024
Language: Английский
Citations
37Mathematics, Journal Year: 2024, Volume and Issue: 12(2), P. 297 - 297
Published: Jan. 17, 2024
Context: YOLO (You Look Only Once) is an algorithm based on deep neural networks with real-time object detection capabilities. This state-of-the-art technology widely available, mainly due to its speed and precision. Since conception, has been applied detect recognize traffic signs, pedestrians, lights, vehicles, so on. Objective: The goal of this research systematically analyze the algorithm, sign recognition systems, from five relevant aspects technology: applications, datasets, metrics, hardware, challenges. Method: study performs a systematic literature review (SLR) studies using published in years 2016–2022. Results: search found 115 primary research. After analyzing these investigations, following results were obtained. most common applications field are vehicular security intelligent autonomous vehicles. majority datasets used train, test, validate YOLO-based systems publicly emphasis Germany China. It also discovered that works present sophisticated detection, classification, processing metrics for by different versions YOLO. In addition, popular desktop data hardwares Nvidia RTX 2080 Titan Tesla V100 and, case embedded or mobile GPU platforms, Jetson Xavier NX. Finally, seven challenges face when operating real road conditions have identified. With mind, reclassified address each case. Conclusions: SLR current work development signs insights provided about future could be conducted improve field.
Language: Английский
Citations
26Solar, Journal Year: 2024, Volume and Issue: 4(3), P. 351 - 386
Published: June 26, 2024
This review presents an investigation into the incremental advancements in YOLO (You Only Look Once) architecture and its derivatives, with a specific focus on their pivotal contributions to improving quality inspection within photovoltaic (PV) domain. YOLO’s single-stage approach object detection has made it preferred option due efficiency. The unearths key drivers of success each variant, from path aggregation networks generalised efficient layer architectures programmable gradient information, presented latest YOLOv10, released May 2024. Looking ahead, predicts significant trend future research, indicating shift toward refining variants tackle wider array PV fault scenarios. While current discussions mainly centre micro-crack detection, there is acknowledged opportunity for expansion. Researchers are expected delve deeper attention mechanisms architecture, recognising potential greatly enhance capabilities, particularly subtle intricate faults.
Language: Английский
Citations
26Electronics, Journal Year: 2024, Volume and Issue: 13(1), P. 236 - 236
Published: Jan. 4, 2024
Recently, the field of vehicle-mounted visual intelligence technology has witnessed a surge interest in pedestrian detection. Existing algorithms for dense detection at intersections face challenges such as high computational weight, complex models that are difficult to deploy, and suboptimal accuracy small targets highly occluded pedestrians. To address these issues, this paper proposes an improved lightweight multi-scale algorithm, YOLOv8-CB. The algorithm introduces cascade fusion network, CFNet (cascade network), CBAM attention module improve characterization feature semantics location information, it superimposes bidirectional weighted path BIFPN structure fuse more effective features performance. It is experimentally verified compared with YOLOv8n model increased by 2.4%, number parameters reduced 6.45%, load 6.74%. inference time single image 10.8 ms. YOLOv8-CB higher lighter scenes streets or intersections. This proposed presents valuable approach device-side limited resources.
Language: Английский
Citations
24IEEE Access, Journal Year: 2024, Volume and Issue: 12, P. 94250 - 94295
Published: Jan. 1, 2024
Quality inspection and defect detection remain critical challenges across diverse industrial applications. Driven by advancements in Deep Learning, Convolutional Neural Networks (CNNs) have revolutionized Computer Vision, enabling breakthroughs image analysis tasks like classification object detection. CNNs' feature learning capabilities made through Machine Vision one of their most impactful This article aims to showcase practical applications CNN models for surface various scenarios, from pallet racks display screens. The review explores methodologies suitable hardware platforms deploying CNN-based architectures. growing Industry 4.0 adoption necessitates enhancing quality processes. main results demonstrate efficacy automating detection, achieving high accuracy real-time performance different surfaces. However, limited datasets, computational complexity, domain-specific nuances require further research. Overall, this acknowledges potential as a transformative technology vision applications, with implications ranging control enhancement cost reductions process optimization.
Language: Английский
Citations
23Digital Signal Processing, Journal Year: 2024, Volume and Issue: 152, P. 104594 - 104594
Published: May 22, 2024
Language: Английский
Citations
22