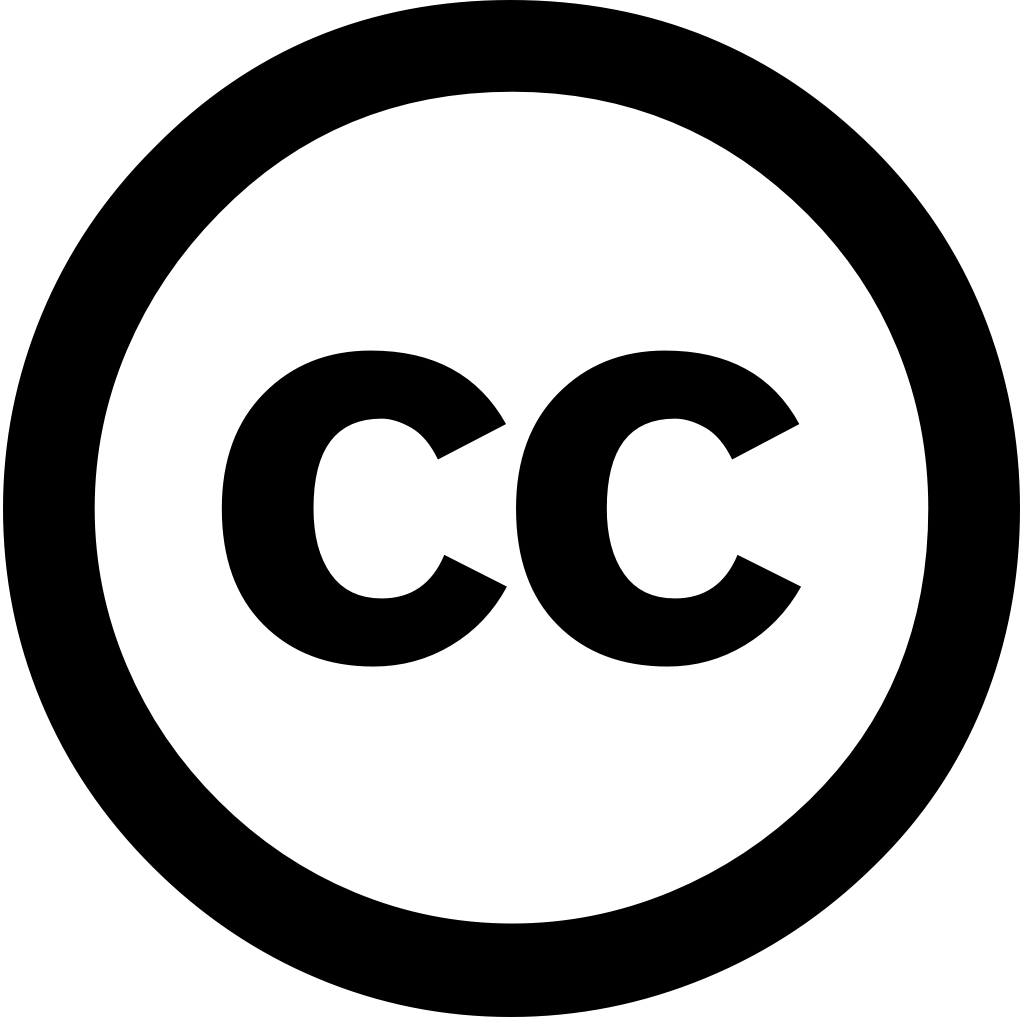
Research Square (Research Square), Journal Year: 2024, Volume and Issue: unknown
Published: April 19, 2024
Language: Английский
Research Square (Research Square), Journal Year: 2024, Volume and Issue: unknown
Published: April 19, 2024
Language: Английский
Multimedia Tools and Applications, Journal Year: 2024, Volume and Issue: 83(33), P. 79939 - 79961
Published: March 1, 2024
Language: Английский
Citations
1IEEE Access, Journal Year: 2024, Volume and Issue: 12, P. 53306 - 53319
Published: Jan. 1, 2024
Masks play a crucial role in preventing respiratory diseases and have diverse applications national public health industrial safety. Efficient mask-wearing detection systems are essential for ensuring accuracy real-time performance. To overcome the challenges of extensive model calculations, parameter volume, complex hardware deployment current system, lightweight mask with improved YOLOv5 is proposed. Firstly, this study proposes new network—EMA-FasterNet, as backbone network YOLOv5, which reduces computation while preserving information each channel. Secondly, using Depthwise Separable Convolutions (DepthSepConv) to replace some C3 modules Neck further compresses parameters, computation. Finally, prevent missing objects due removal overlapping candidate boxes, use Soft Non-Maximum Suppression (NMS) NMS. Compared YOLOv5s, proposed YOLOv5-S2C2 has high mAP both Dataset I II, parameters reduced by about 56% 57.0%, respectively. The volume 44% original inference speed on GPU 30%. These improvements show that achieves design excellent It also well suited efficient limited computational resources.
Language: Английский
Citations
0Research Square (Research Square), Journal Year: 2024, Volume and Issue: unknown
Published: April 19, 2024
Language: Английский
Citations
0