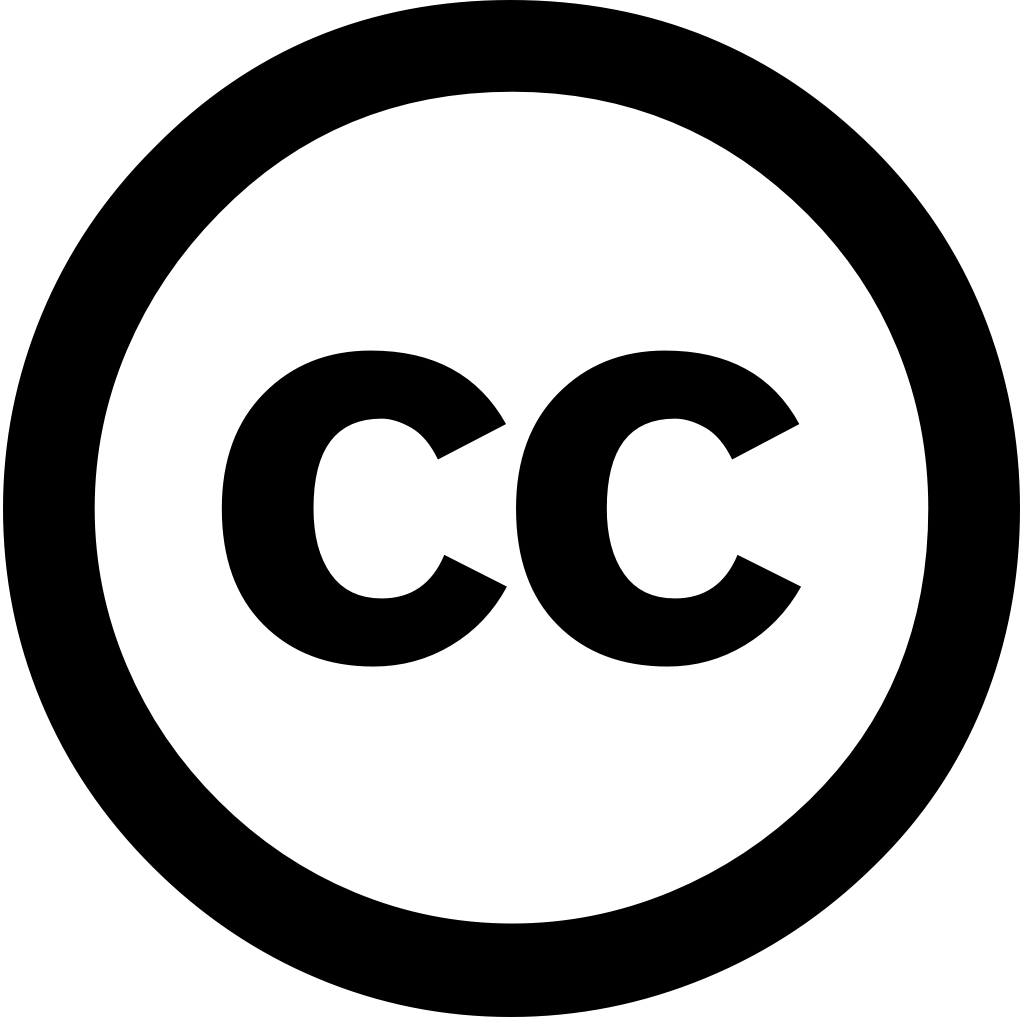
Research Square (Research Square), Journal Year: 2024, Volume and Issue: unknown
Published: Dec. 20, 2024
Language: Английский
Research Square (Research Square), Journal Year: 2024, Volume and Issue: unknown
Published: Dec. 20, 2024
Language: Английский
The Journal of Supercomputing, Journal Year: 2024, Volume and Issue: 80(16), P. 23867 - 23910
Published: July 29, 2024
Language: Английский
Citations
1Multimedia Tools and Applications, Journal Year: 2023, Volume and Issue: 83(11), P. 32411 - 32422
Published: Sept. 21, 2023
Language: Английский
Citations
3Communications in computer and information science, Journal Year: 2024, Volume and Issue: unknown, P. 129 - 140
Published: Jan. 1, 2024
Language: Английский
Citations
0The Journal of Supercomputing, Journal Year: 2024, Volume and Issue: 80(19), P. 27073 - 27094
Published: Aug. 29, 2024
Abstract We propose a framework designed to tackle multi-objective optimization challenge related the placement of applications in fog computing, employing deep reinforcement learning (DRL) approach. Unlike other techniques, such as integer linear programming or genetic algorithms, DRL models are applied real time solve similar problem situations after training. Our model comprises process featuring graph neural network and two actor-critics, providing holistic perspective on priorities concerning interconnected services that constitute an application. The incorporates relationships between crucial factor decisions: Services with higher dependencies take precedence location selection. experimental investigation involves illustrative cases where we compare our results baseline strategies algorithms. observed comparable Pareto set negligible execution times, measured order milliseconds, contrast hours required by alternative approaches.
Language: Английский
Citations
0International Journal of Communication Systems, Journal Year: 2024, Volume and Issue: unknown
Published: Sept. 8, 2024
Summary On‐demand manufacturing in Industry 4.0 requires flexibility of the networks which can be provided with fifth generation (5G) mobile communications wireless connectivity. A key component efficient utilization radio resources a scenario is network resource management (NRM). We show how NRM automated artificial intelligence (AI). introduce several futuristic industrial use cases that require AI various parts process. analyze components' benefits and disadvantages deployment scenarios. The findings used by business stakeholders interested deploying 5G cellular to choose best implementation strategy for particular case. there are many viable options process automation, but cost has considered all cases. Also, we point out an essential component, standardized information flow on status productivity performance indicators (KPIs), needed successful application AI.
Language: Английский
Citations
0National Academy Science Letters, Journal Year: 2024, Volume and Issue: unknown
Published: Nov. 25, 2024
Language: Английский
Citations
0Research Square (Research Square), Journal Year: 2024, Volume and Issue: unknown
Published: Dec. 20, 2024
Language: Английский
Citations
0