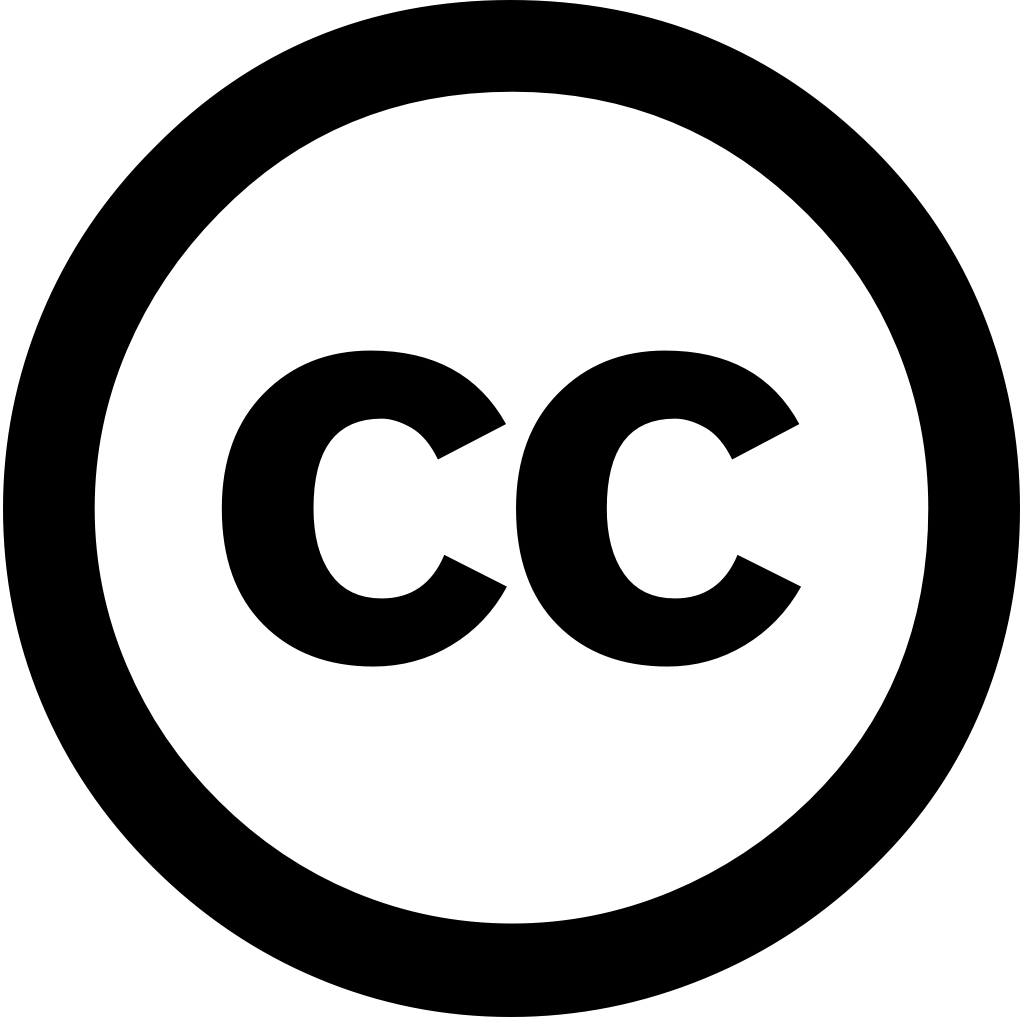
Geomechanics and Geophysics for Geo-Energy and Geo-Resources, Journal Year: 2024, Volume and Issue: 10(1)
Published: Oct. 4, 2024
Language: Английский
Geomechanics and Geophysics for Geo-Energy and Geo-Resources, Journal Year: 2024, Volume and Issue: 10(1)
Published: Oct. 4, 2024
Language: Английский
Natural Resources Research, Journal Year: 2025, Volume and Issue: unknown
Published: April 13, 2025
Language: Английский
Citations
0Energy Geoscience, Journal Year: 2025, Volume and Issue: unknown, P. 100411 - 100411
Published: April 1, 2025
Language: Английский
Citations
0International Journal of Hydrogen Energy, Journal Year: 2025, Volume and Issue: 128, P. 406 - 424
Published: April 17, 2025
Language: Английский
Citations
0Journal of Petroleum Exploration and Production Technology, Journal Year: 2025, Volume and Issue: 15(5)
Published: April 19, 2025
Language: Английский
Citations
0Petroleum Science and Technology, Journal Year: 2025, Volume and Issue: unknown, P. 1 - 20
Published: April 29, 2025
Language: Английский
Citations
0Journal of Alloys and Compounds, Journal Year: 2025, Volume and Issue: unknown, P. 180709 - 180709
Published: May 1, 2025
Language: Английский
Citations
0Energy Geoscience, Journal Year: 2024, Volume and Issue: 5(4), P. 100341 - 100341
Published: Sept. 6, 2024
Language: Английский
Citations
2SPE Journal, Journal Year: 2024, Volume and Issue: unknown, P. 1 - 17
Published: Oct. 1, 2024
Summary The identification of sweet spots, areas within a reservoir with the highest production potential, has been revolutionized by integration machine learning (ML) algorithms. This review explores advancements in sweet-spot techniques driven ML, analyzing 122 research papers published OnePetro, Elsevier, ScienceDirect, SpringerLink, GeoScienceWorld, and MDPI databases last 10 years. provides comprehensive analysis ML applications highlights best practices data collection, preprocessing, feature engineering, model selection, training, validation, optimization, evaluation. paper categorizes discusses different types used algorithms into six groups, analyzes combinations frequently for training visualizes distribution input parameters features each main categories. It also examines frequency target variables these models. In addition, it various supervised unsupervised key studies offering valuable insights researchers.
Language: Английский
Citations
2Journal of Petroleum Exploration and Production Technology, Journal Year: 2024, Volume and Issue: unknown
Published: Sept. 14, 2024
Language: Английский
Citations
1Journal of Petroleum Exploration and Production Technology, Journal Year: 2024, Volume and Issue: 14(10), P. 2687 - 2701
Published: Aug. 16, 2024
This study employs comprehensive source rock evaluation using seismic inversion, rock-eval pyrolysis, organic petrography and basin modeling techniques. The kerogen type is determined by the Van Krevelen diagram, confirming Bahu-01, Nandpur-01 Zakria-01 wells have III, whereas Panjpir-01 well exhibits II to III. TOC was calculated core data from (34) samples employing geochemistry technique post stack applied on 2D data. Bahu-01 indicates poor potential, with an average value of 0.34%. In contrast, represent moderate good richness, values 1.25% 1.36%, respectively. However, a 0.72%, fair richness. Maturity estimation reveals that Panjpir-01, vitrinite reflectance (%Ro) below 0.50, indicating immature rock. %Ro for 0.63, maturity in early oil window, peak generation occurring during Eocene age. Finally, proves mature western part only future hydrocarbon exploration should be focused area. integrated approach novel Punjab Platform. diverse methodologies enhanced our understanding about characteristics pursuing resources. An will also provide valuable insights numerous other basins worldwide.
Language: Английский
Citations
0