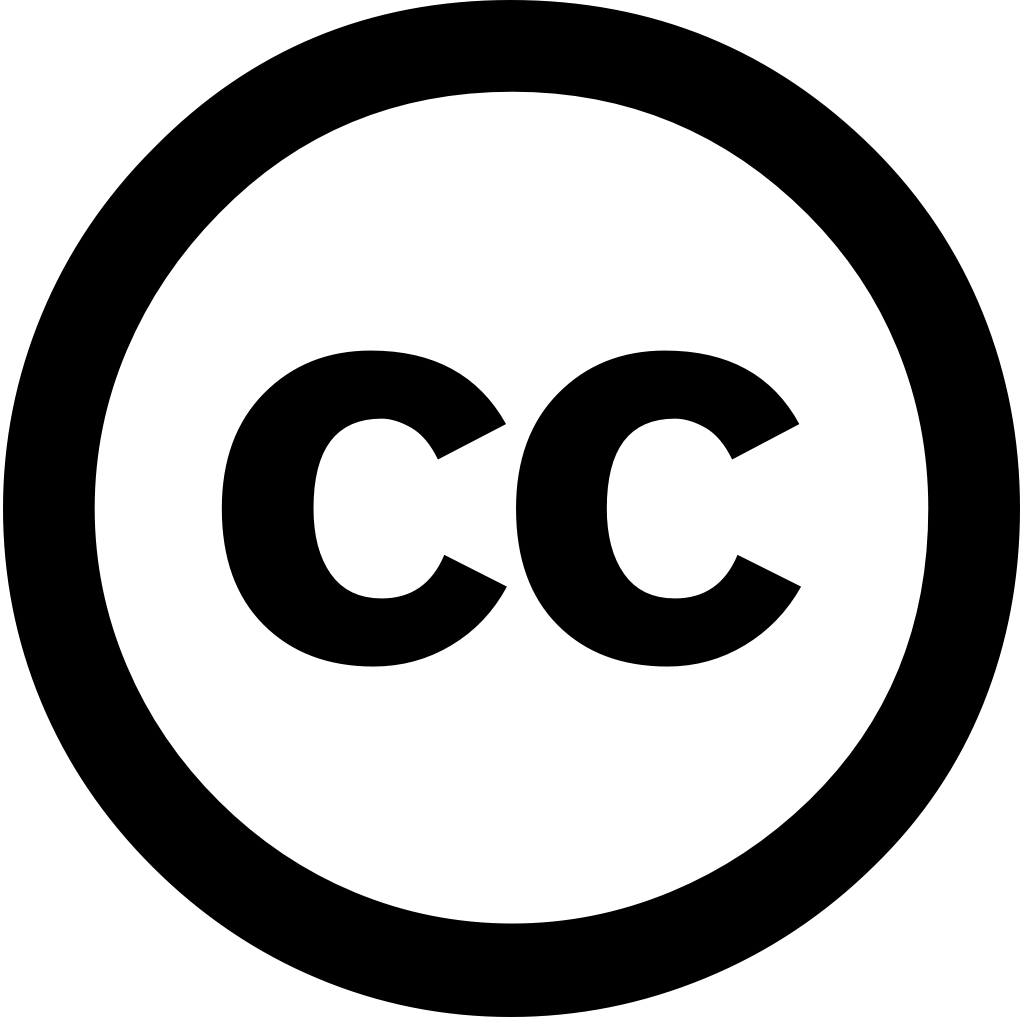
Research Square (Research Square), Journal Year: 2024, Volume and Issue: unknown
Published: Aug. 13, 2024
Language: Английский
Research Square (Research Square), Journal Year: 2024, Volume and Issue: unknown
Published: Aug. 13, 2024
Language: Английский
Applied Sciences, Journal Year: 2023, Volume and Issue: 13(22), P. 12147 - 12147
Published: Nov. 8, 2023
This paper offers a comprehensive overview of machine learning (ML) methodologies and algorithms, highlighting their practical applications in the critical domain water resource management. Environmental issues, such as climate change ecosystem destruction, pose significant threats to humanity planet. Addressing these challenges necessitates sustainable management increased efficiency. Artificial intelligence (AI) ML technologies present promising solutions this regard. By harnessing AI ML, we can collect analyze vast amounts data from diverse sources, remote sensing, smart sensors, social media. enables real-time monitoring decision making applications, including irrigation optimization, quality monitoring, flood forecasting, demand enhance agricultural practices, distribution models, desalination plants. Furthermore, facilitates integration, supports decision-making processes, enhances overall sustainability. However, wider adoption faces challenges, heterogeneity, stakeholder education, high costs. To provide an management, research focuses on core fundamentals, major (prediction, clustering, reinforcement learning), ongoing issues offer new insights. More specifically, after in-depth illustration algorithmic taxonomy, comparative mapping all specific tasks. At same time, include tabulation works along with some concrete, yet compact, descriptions objectives at hand. leveraging tools, develop plans address world’s supply concerns effectively.
Language: Английский
Citations
51Water Resources Management, Journal Year: 2025, Volume and Issue: unknown
Published: Feb. 11, 2025
Language: Английский
Citations
3Environmental Science and Pollution Research, Journal Year: 2023, Volume and Issue: 30(35), P. 83845 - 83872
Published: June 23, 2023
Language: Английский
Citations
30Engineering Applications of Artificial Intelligence, Journal Year: 2022, Volume and Issue: 117, P. 105545 - 105545
Published: Nov. 10, 2022
Language: Английский
Citations
38Scientific Reports, Journal Year: 2023, Volume and Issue: 13(1)
Published: Jan. 27, 2023
Abstract Accurate medium and long-term runoff forecasts play a vital role in guiding the rational exploitation of water resources improving overall efficiency use. Machine learning is becoming common trend time series forecasting research. Least squares support vector machine (LSSVM) grey model (GM(1,1)) have received much attention predicting rainfall last two years. “Decomposition-forecasting” has become one most important methods for data. Complete ensemble empirical mode decomposition with adaptive noise (CEEMDAN) method powerful advantages dealing nonlinear strong fitting ability good robustness. Gray can solve problems little historical data low serial integrity reliability. Based on their respective advantages, combined CEEMDAN–LSSVM–GM(1,1) was developed applied to prediction lower Yellow River. To verify reliability model, results were compared single LSSVM CEEMDAN–LSSVM CEEMDAN–support machines (SVM)–GM(1,1). The show that high accuracy are better than other models, which provides an effective regional application prospects.
Language: Английский
Citations
22International Journal of Applied Earth Observation and Geoinformation, Journal Year: 2023, Volume and Issue: 119, P. 103330 - 103330
Published: April 29, 2023
Global Digital Elevation Models (GDEMs) have been increasingly used to assess the risk of flooding worldwide. However, their effectiveness and performance flood models in areas with complex terrain, such as Jeddah, Saudi Arabia, not comprehensively studied. This study aims compare five distinct − 30-m SRTM, ASTER, 90-m MERIT, 10 m Sentinel-1 DEM, 12.5 ALOSPALSAR - estimating inundation extent depth Jeddah watershed, including three dams. Both hydrological hydraulic modeling approaches were utilized achieve this objective. The findings revealed that all produced similar watershed boundaries mountain area (Wadi Qaws), except for ALOS-PALSAR, which generated a different boundary from previous reports studies. GDEMs failed accurately delineate drainage one dams, SRTM ALOS-PALSAR. Moreover, ASTER products provided closest estimates ground observations, producing peak discharges 114.1 m3/s 110 m3/s, respectively. For entire encompassing mountain, urban, coastal areas, demonstrated significant differences boundary, streams, outlet location, discharge at outlet. In addition, each GDEM's map was significantly distinct. Overall, results suggest outperformed other area.
Language: Английский
Citations
19Natural Resources Research, Journal Year: 2023, Volume and Issue: 33(1), P. 163 - 190
Published: Dec. 10, 2023
Language: Английский
Citations
19Journal of Hydrology, Journal Year: 2024, Volume and Issue: 636, P. 131279 - 131279
Published: May 7, 2024
Language: Английский
Citations
6Water, Journal Year: 2023, Volume and Issue: 15(20), P. 3576 - 3576
Published: Oct. 12, 2023
Rainfall–runoff modeling has been the core of hydrological research studies for decades. To comprehend this phenomenon, many machine learning algorithms have widely used. Nevertheless, a thorough comparison and effect pre-processing on their performance is still lacking in literature. Therefore, major objective to simulate rainfall runoff using nine standalone hybrid models. The conventional models include artificial neural networks, least squares support vector machines (LSSVMs), K-nearest neighbor (KNN), M5 model trees, random forests, multiple adaptive regression splines, multivariate nonlinear regression. In contrast, comprise LSSVM KNN coupled with gorilla troop optimizer (GTO). Moreover, present study introduces new combination feature selection method, principal component analysis (PCA), empirical mode decomposition (EMD). Mean absolute error (MAE), root mean squared (RMSE), relative RMSE (RRMSE), person correlation coefficient (R), Nash–Sutcliffe efficiency (NSE), Kling Gupta (KGE) metrics are used assessing developed proposed applied data collected Wadi Ouahrane basin, Algeria. According results, KNN–GTO exhibits best (MAE = 0.1640, 0.4741, RRMSE 0.2979, R 0.9607, NSE 0.9088, KGE 0.7141). These statistical criteria outperform other by 80%, 70%, 72%, 77%, 112%, 136%, respectively. provides worst results without data. findings indicate that selection, PCA, EMD significantly improves accuracy rainfall–runoff modeling.
Language: Английский
Citations
16Expert Systems with Applications, Journal Year: 2023, Volume and Issue: 222, P. 119811 - 119811
Published: March 8, 2023
Language: Английский
Citations
15