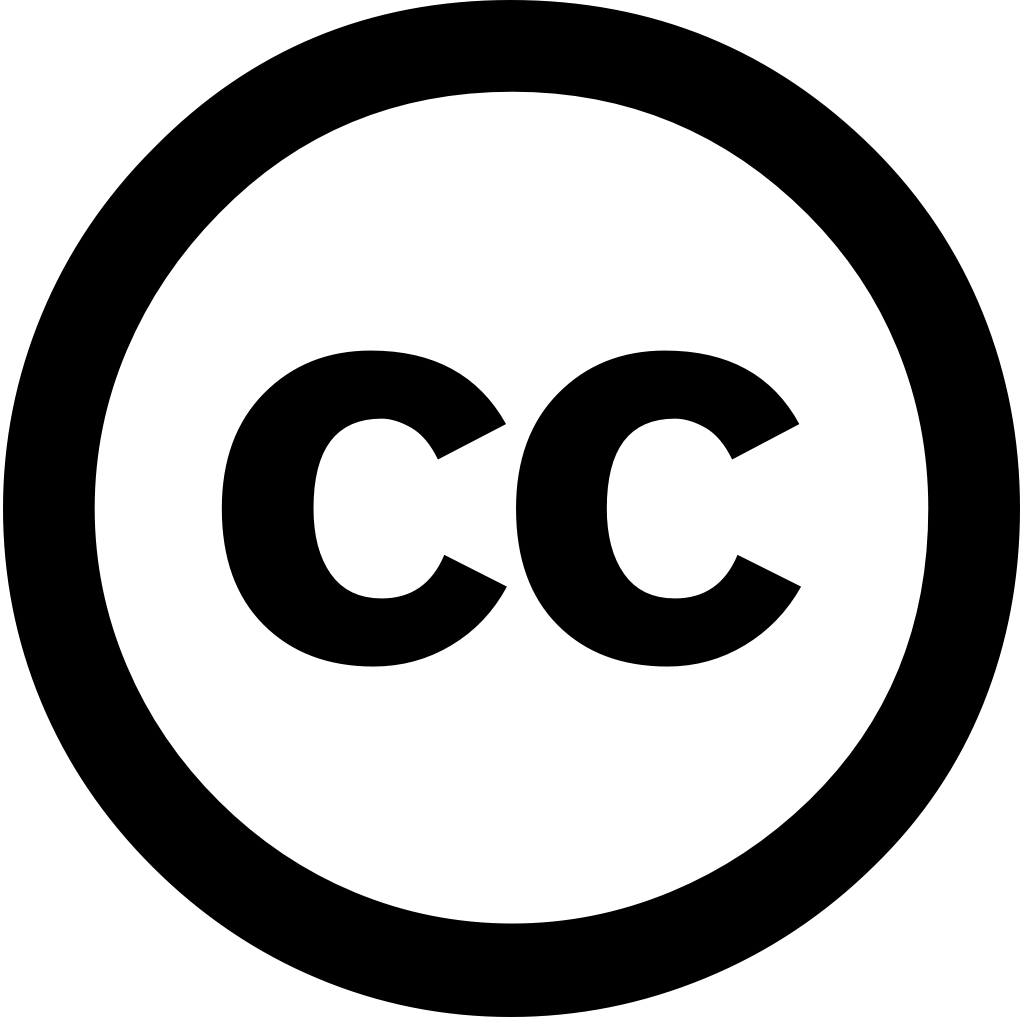
Research Square (Research Square), Journal Year: 2024, Volume and Issue: unknown
Published: Aug. 13, 2024
Language: Английский
Research Square (Research Square), Journal Year: 2024, Volume and Issue: unknown
Published: Aug. 13, 2024
Language: Английский
Scientific Reports, Journal Year: 2024, Volume and Issue: 14(1)
Published: Dec. 30, 2024
Language: Английский
Citations
1Remote Sensing, Journal Year: 2023, Volume and Issue: 15(12), P. 3015 - 3015
Published: June 9, 2023
Climate change has led to an increased frequency of extreme precipitation events, resulting in damage from rainstorms and floods. Rapid efficient flood forecasting is crucial. However, traditional hydrological simulation methods that rely on site distribution are limited by the availability data cannot provide fast accurate monitoring information. Therefore, this study took event Huoqiu County 2020 as example proposes a three-dimensional method based active passive satellites, which provides effective information support for disaster prevention mitigation. The experimental results indicated following: (1) flood-inundated area was 704.1 km2, with Jiangtang Lake section Huaihe River southern part Chengdong being largest affected areas; (2) water levels ranged 15.36 m 17.11 m, 4–6 higher than original level. highest level areas were flat south Lake, north Chengxi having greatest increase; (3) depth primarily between 4 7 total storage capacity 2833.47 million m3, capacity; (4) rainstorm caused direct economic loss approximately CNY 7.5 billion population 91 thousand people. Three-dimensional floods comprehensively reflects inundation status can valuable prediction management.
Language: Английский
Citations
3Water Science & Technology Water Supply, Journal Year: 2023, Volume and Issue: 23(11), P. 4403 - 4415
Published: Oct. 31, 2023
Abstract Flood routing is vital in helping to reduce the impact of floods on people and communities by allowing timely appropriate responses. In this study, empirical mode decomposition (EMD) signal technique combined with cascade forward backpropagation neural network (CFBNN) feed-forward (FFBNN) machine learning (ML) techniques model 2014 Ankara, Mera River. The data are split order avoid underfitting overfitting problems algorithm. While establishing algorithm, 70% were divided into training, 15% testing validation. Graphical indicators statistical parameters used for analysis performance. As a result, EMD has been found improve performance ML models. addition, EMD-FFBNN hybrid showed most accurate estimation results flood calculation. study's outputs can assist designing control structures such as levees dams help risk.
Language: Английский
Citations
3Research Square (Research Square), Journal Year: 2024, Volume and Issue: unknown
Published: Aug. 13, 2024
Language: Английский
Citations
0