PM 2.5 Concentration 7-days Prediction in the Beijing-Tianjin-Hebei Region Using a Novel Stacking Framework
Abstract
High-precision
prediction
of
near-surface
PM2.5
concentration
is
an
significant
theoretical
prerequisite
for
effective
monitoring
and
prevention
air
pollution,
also
provides
guiding
suggestions
health
risk
control.
In
view
the
fact
that
control
variables
existing
models
are
mostly
dependent
on
influencing
factors
at
near-surface,
it
often
difficult
to
fully
explore
continuous
spatio-temporal
characteristics
in
PM2.5.
this
study,
MODIS
remote
sensing-derived
Aerosol
Optical
Depth
(AOD)
daily
data,
atmospheric
environment
ground
station
data
meteorological
introduced
identify
strong
correlation
factors.
A
highly
robust
seven-day
model
constructed
based
Stacking
algorithm
combined
with
various
machine
learning
methods
improve
generalisation
ability
model;
estimation
integrated
compared
analyzed
LSTM,
RF
KNN
models.
The
results
demonstrated
basis
RF-LSTM-Stacking
exhibited
a
better
fit,
R²,
RMSE,
MAE
values
0.95,
7.74
µg/m³,
6.08
respectively.
This
approach
improved
accuracy
by
approximately
17%
single
model.
Based
was
evident
LSTM-RF
model,
fusion-based
algorithm,
significantly
enhanced
provided
reference
predicting
early
warning
monitoring.
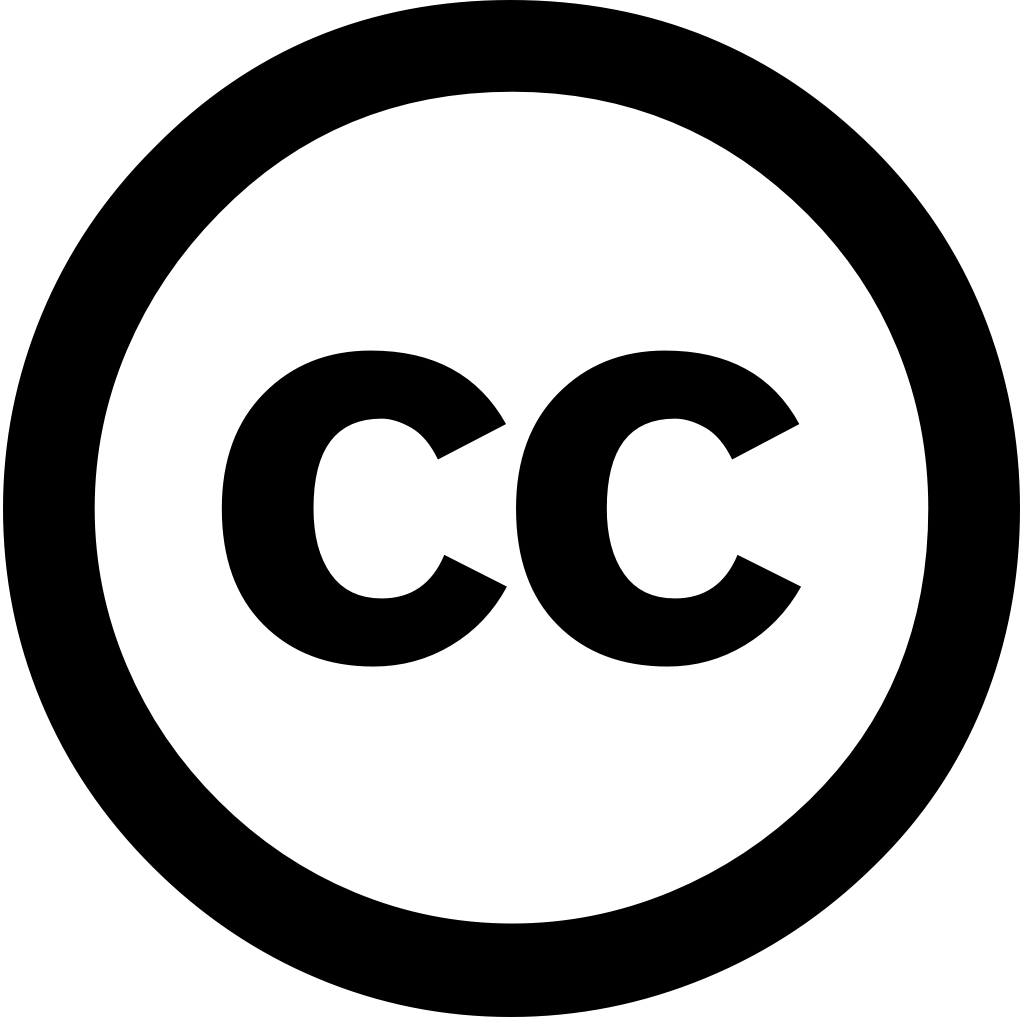
Research Square (Research Square), Journal Year: 2025, Volume and Issue: unknown
Published: Feb. 17, 2025
Language: Английский